Data Analysis of Pollution: Insights and Innovations
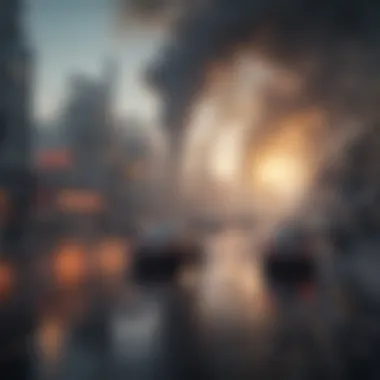
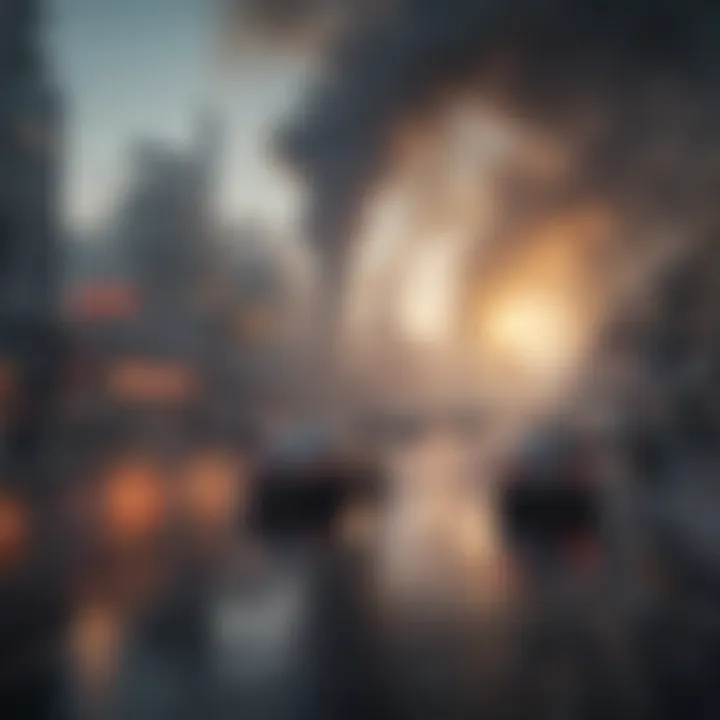
Intro
Pollution remains one of the pressing issues of our time, affecting both environmental and public health. With increasing urbanization, industrial activities, and various human behaviors, the need for effective data analysis in pollution control has become paramount. Understanding the dynamics of pollutants and their impact requires robust methods of data collection and interpretation. This article aims to provide a comprehensive overview of methods, insights, and challenges in pollution data analysis, serving a variety of readers from students to established researchers.
Research Overview
Summary of Key Findings
Pollution data analysis reveals several critical trends. Firstly, air quality has deteriorated in various urban areas, particularly due to increased vehicular emissions and industrial discharges. Secondly, water pollution from agricultural runoff has led to a noticeable decline in freshwater quality. Solid waste management, another vital aspect, showcases alarming trends in increasing plastic use and inadequate recycling efforts.
The research draws attention to technology's role, highlighting the emergence of advanced tools like machine learning and satellite data that facilitate better understanding and management of pollution. Data-driven methodologies have enabled policymakers to make informed decisions in addressing these challenges, though significant obstacles remain.
Importance of the Research
The significance of this research lies in its multifaceted approach to pollution. With the capacity for data analysis to shape environmental policies and public awareness, understanding pollution trends equips stakeholders with the necessary insights for effective action. Without proper analysis, efforts to mitigate pollution can be misguided and ineffective, harming both ecosystems and human health.
Methodology
Study Design
The approach adopted in this research involved a mixed-methods design, integrating quantitative and qualitative analyses. This included statistical evaluations of pollution data retrieved from various sources. The incorporation of various data collection methodologies ensured a more comprehensive understanding of pollution trends.
Data Collection Techniques
Data collection included several techniques to gather relevant information on pollution levels. Importance was given to:
- Remote Sensing: Using satellite imagery to track land-use changes and their impact on pollution.
- Surveys and Sampling: Conducting on-ground surveys to assess public perception and health impacts.
- Existing Databases: Leveraging data from environmental agencies and health organizations to analyze trends over time.
Collectively, these techniques provide a clearer and more detailed picture of pollution, highlighting areas in need of immediate attention while also tracking progress over time.
Foreword to Pollution Data Analysis
Understanding pollution and its various forms is crucial in today's world. Pollution affects not just our environment but also our health and well-being. Given this, data analysis plays a significant role in identifying trends and impacts of pollution. Collecting and analyzing data can reveal patterns that might remain unnoticed without a structured approach. The insights gained can inform policy decisions and foster environmental protection initiatives.
Defining Pollution
Pollution refers to the introduction of harmful substances or contaminants into the environment. These substances disrupt natural processes and detrimentally affect living organisms. Common types of pollution include air, water, soil, and noise. Each type presents unique challenges and requires distinct monitoring and analysis methods. Understanding these definitions is the foundation for any analysis.
Importance of Data Analysis in Environmental Studies
Data analysis is essential in environmental studies for several reasons:
- Informed Decision-Making: Using collected data helps policymakers make rational, informed decisions regarding environmental regulations and standards.
- Trend Identification: Analyzing data over time reveals trends in pollution levels, which can indicate whether conditions are improving or worsening.
- Resource Allocation: With clear analysis, governments and organizations can allocate resources where they are most needed, optimizing response efforts.
- Public Awareness: Data accessibility can increase public awareness and engagement about pollution issues, promoting community action for environmental health.
"Data is the new oil, and it fuels compliance and progressive action for tackling pollution."
As pollution issues become more pressing, a solid grasp of data analysis will contribute to creating effective strategies and solutions.
Types of Pollution Analyzed
The analysis of different types of pollution plays a crucial role in understanding the broader environmental impact. Each type highlights unique challenges and requires tailored data collection and analysis methods. Focusing on air, water, soil, and noise pollution allows for a structured approach to environmental management. By categorizing pollution types, researchers can more effectively identify trends and offer informed solutions. Moreover, targeted analysis can enhance public awareness, policy formulation, and regulatory standards.
Air Pollution
Air pollution constitutes a critical area of concern, affecting both human health and the environment. It results from various sources, including vehicular emissions, industrial discharges, and domestic heating. The data analysis in this realm involves monitoring specific pollutants such as nitrogen dioxide, sulfur dioxide, and particulate matter. Effective analysis can provide insights into emission sources and their health impacts, guiding policies aimed at improving air quality. Furthermore, advancements in real-time monitoring systems have improved data accuracy, allowing for more responsive regulatory actions.
Water Pollution
Water pollution directly impacts ecosystems and human health. It occurs through contamination from industrial processes, agricultural runoff, and untreated sewage. Analyzing water pollution entails examining chemical, biological, and physical parameters. Strategies like watershed assessments and water quality modeling are vital to understand the distribution of pollutants. On a broader level, comprehensive water pollution data supports effective management practices and policies, particularly in agricultural and urban environments. This analysis can also reveal long-term trends, aiding in predictive modeling for future water quality scenarios.
Soil Contamination
Soil contamination is often overlooked yet profoundly impacts agriculture and biodiversity. Contaminants such as heavy metals, pesticides, and industrial waste can diminish soil quality and productivity. Data analysis in this area focuses on identifying contamination sources and mapping affected areas. Techniques like geospatial analysis can elucidate the extent of contamination, enabling remediation efforts. Understanding soil quality trends informs agricultural practices and sustainable land use, ultimately benefiting the environment and human health.
Noise Pollution
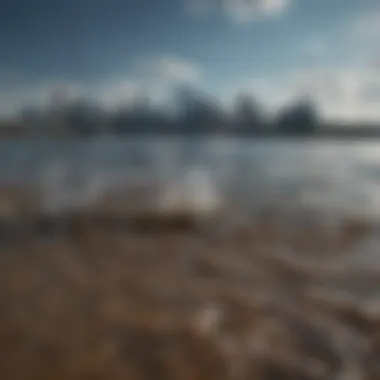
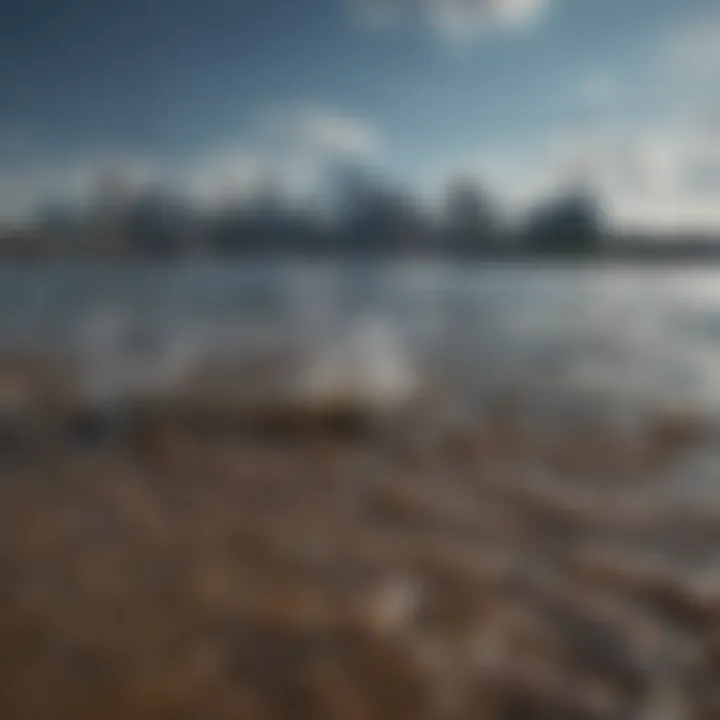
Noise pollution is another significant yet frequently disregarded aspect of environmental concerns. Sources include traffic, construction, and industrial activity. The analysis of noise pollution requires a different set of methodologies, often involving sound level monitoring and human impact studies. Data on noise exposure can drive urban planning decisions and public awareness campaigns, especially in densely populated areas. Research indicates that long-term exposure to noise can lead to adverse health effects, underlining the need for rigorous data collection and analysis.
Data Collection Methods
Data collection methods play a crucial role in pollution data analysis. They provide the foundation for gathering accurate and reliable information on pollution levels across diverse environments. Without robust data collection methods, analysis becomes unreliable, leading to misguided conclusions and ineffective solutions. The right techniques not only enhance the precision of collected data but also help in identifying pollution trends and sources. It is important to choose methods that are suitable for the specific type of pollution being studied, as this can influence the outcomes and subsequent actions.
Field Monitoring Techniques
Field monitoring techniques involve direct measurement of pollutants in their environment. These methods are typically more hands-on and can yield immediate results. Devices like air quality monitors, water sampling kits, and soil sensors are commonly used. Field monitoring has several advantages:
- Real-time Data: Measurements taken on-site give up-to-date information about pollution levels.
- Contextual Understanding: Observing the environment provides insights into the conditions affecting pollution.
- Local Relevance: Data is specific to the area and can thus inform local policy decisions.
However, there are challenges associated with field monitoring. The need for trained personnel to operate devices and interpret results can lead to inconsistencies. Moreover, external factors such as weather conditions can impact the reliability of the data.
Remote Sensing Technologies
Remote sensing technologies offer a different approach to data collection. These methods involve gathering information through satellites, drones, or aerial surveys. Remote sensing is particularly useful for large areas where it would be impractical to monitor pollution through field methods alone. Some significant benefits include:
- Wide Coverage: Remote sensing can cover extensive geographic areas, enabling comprehensive data collection.
- Cost-Effective: Once setup is established, it can significantly lower costs compared to traditional methods over time.
- Historical Data: Remote sensors often archive images and data over time, allowing for trend analysis and comparisons.
Despite its advantages, remote sensing can have limitations. The resolution of the data may not always be sufficient for specific studies, and the interpretation of remote data often requires skilled analysts.
Laboratory Analysis Approaches
Laboratory analysis approaches involve collecting samples from the environment for detailed examination. This method is essential for understanding the chemical makeup of pollutants and their effects. Common practices include:
- Chemical Testing: Analyzing samples to identify the concentration and types of pollutants present.
- Biological Assessments: Studying samples to evaluate the impact of pollutants on local ecosystems.
- Physical Properties Measurement: Understanding factors like pH, conductivity, or turbidity in water samples.
The strength of laboratory analysis lies in its ability to provide thorough and controlled assessments of pollutants. However, challenges include the time required for analysis and the potential for contamination during sampling and processing.
Understanding the strengths and limitations of various data collection methods is essential for informed decisions in pollution management.
Data Analysis Techniques
Data analysis techniques play a pivotal role in understanding pollution data. They not only aid in assessing the current state of environmental conditions but also help predict future trends. These techniques empower researchers to break down complex data sets derived from multiple pollution sources, thus allowing for informed decision-making. By employing these analytical methods, it is possible to derive actionable insights which can influence policies and public health initiatives.
Statistical Analysis
Statistical analysis is a fundamental component of pollution data analysis. This method allows researchers to examine relationships between variables, revealing patterns and trends that would otherwise remain hidden. Various statistical tools, such as regression analysis, are commonly employed to assess the impact of pollution levels on public health outcomes. By calculating measures like mean, median and standard deviation, researchers can summarize large data sets effectively.
Moreover, hypothesis testing can be used to affirm or dispute theories related to pollution. For instance, if a specific pollutant is suspected to affect respiratory health, statistical tests can determine if the observed health outcomes significantly relate to pollutant levels.
Geospatial Analysis
Geospatial analysis enhances our understanding of pollution by spatially analyzing data. This technique utilizes geographic information systems (GIS) to map pollution sources and their effects. Geospatial data allows for a visual representation of pollution distribution across different regions, making it easier to identify hotspots. For example, air quality data can be mapped to determine which areas are most adversely affected by industrial emissions.
Additionally, the spatial correlation between pollution and socio-economic data can provide insights into environmental justice issues. Understanding how pollution affects various communities unequally is critical for developing targeted interventions.
Predictive Modeling
Predictive modeling is another crucial method in pollution data analysis. By using historical data, these models forecast future pollution levels and trends. They rely on algorithms that analyze past patterns to predict future occurrences. For instance, machine learning algorithms can ingest large data sets to learn from historical pollution data and different variables that contribute to pollution levels.
Such predictive capabilities can be invaluable in environmental planning. Policymakers can use these models to anticipate pollution spikes and enact preventative measures. Moreover, industries can apply predictive models to better anticipate their emissions during peak production times.
"The application of predictive modeling will be essential for proactive environmental management in the coming decades."
In summary, statistical analysis, geospatial analysis, and predictive modeling are integral to understanding and combatting pollution. Each technique offers distinct advantages and insights that can guide research and public policy. By utilizing these methods, stakeholders can address pollution more effectively, working towards sustainable solutions.
Challenges in Pollution Data Analysis
Data analysis in the realm of pollution is profound in addressing environmental issues. However, it comes with a series of challenges that can hinder effective understanding and action. These challenges are not merely technical but also systemic, impacting the entire process of pollution data interpretation.
Data Quality and Reliability
The reliability of pollution data is central to any analysis. Poor data quality can lead to incorrect conclusions and misguided policies. Factors affecting data quality include sensor malfunction, human error in data entry, and insufficient calibration of measuring instruments. Moreover, different countries and organizations may adhere to varying standards in data collection. Thus, ensuring consistency is necessary to maintain data integrity.
It is vital for researchers to routinely verify and validate data. This can involve cross-referencing data against known benchmarks or using statistical methods to identify anomalies. When policymakers rely on compromised data, the implications can be far-reaching—resulting in ineffective regulations or misallocation of resources. The importance of stringent data quality measures cannot be overstated, as they provide the foundation for sound decision-making.
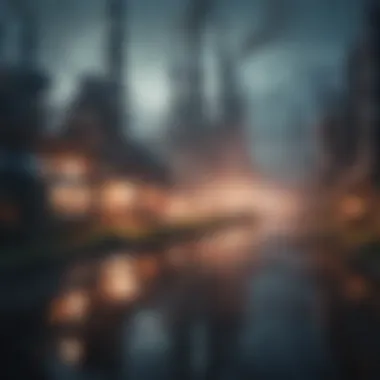
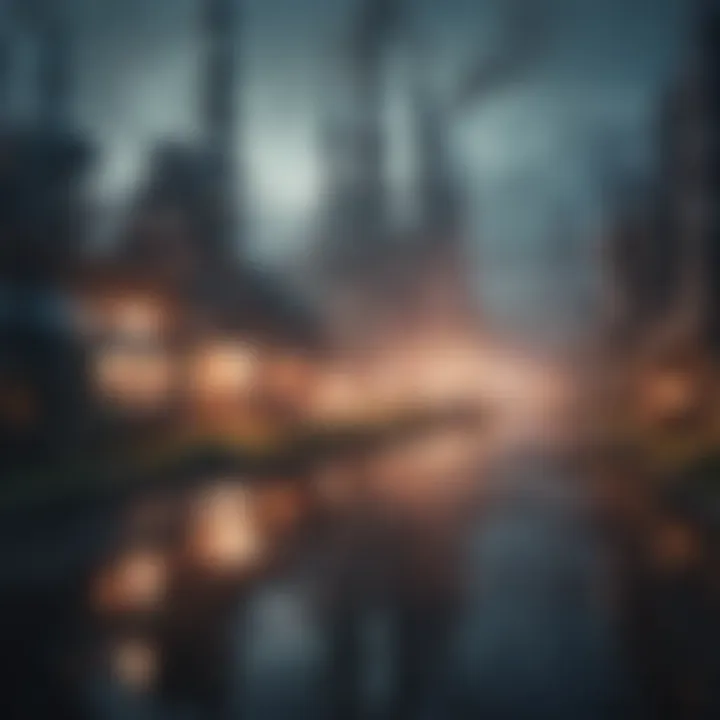
Interoperability of Data Sources
In pollution data analysis, the ability to integrate various data sources is critical. Data can come from diverse sources, including governmental agencies, non-profits, and private organizations. Each source may use different formats, terminologies, and methodologies. Consequently, analysts face the challenge of integrating these heterogeneous datasets into a cohesive framework. This interoperability is not simply a technical hurdle; it also requires establishing common standards and protocols.
Efforts to enhance interoperability through emerging technologies, like Application Programming Interfaces (APIs) and standard data formats, have made strides. However, the task is ongoing. Effective interoperability promotes comprehensive analyses, leading to better insights into pollution trends and sources. Furthermore, it can enhance collaboration among different stakeholders, creating a unified front in addressing environmental challenges.
Analytical Limitations
Analytical limitations are inherent in any data analysis process, particularly regarding pollution data. These limitations can arise from the methods employed, model assumptions, or data availability. For instance, statistical models may oversimplify complex relationships between pollutants and health effects. Furthermore, predictive models depend heavily on historical data; if the environmental conditions change, the predictions may become less reliable.
Moreover, there is often a scarcity of data, especially in underserved regions. This absence of data can lead to a skewed understanding of pollution's impact. As such, researchers must be cautious when extrapolating findings from limited datasets. Acknowledging these analytical limitations helps frame research within a more realistic context and points towards areas that require further exploration.
"Challenges in data quality, interoperability, and analytical limitations pose significant obstacles to understanding pollution effectively."
While these challenges are daunting, recognizing them is the first step towards improvement. Enhanced training for data analysts, better tools for data integration, and continuous evaluation of methodologies can provide substantial progress in overcoming these barriers. Addressing these issues is essential for effective pollution management and for developing actionable strategies that benefit both the environment and public health.
Technological Innovations in Pollution Monitoring
Technological innovations are vital in enhancing our understanding and assessment of pollution. This section will elucidate how emerging technologies enable more accurate monitoring, provide timely analysis, and foster effective responses to environmental issues. By adopting these new tools, we can significantly improve the methods of pollution monitoring and obtain invaluable insights for better decision-making in environmental policy and public health.
Advancements in Sensor Technologies
The field of sensor technology has seen remarkable progress. Modern sensors are now more sophisticated, allowing for greater sensitivity and precision when detecting pollutants. They can be deployed in diverse environments, from urban centers to remote areas. Key benefits of these advancements include:
- Real-time Monitoring: Sensors can provide immediate data on pollution levels, enabling swift action when thresholds are exceeded.
- Cost-Effectiveness: Contemporary sensors are generally more affordable, allowing for widespread deployment across various locations without substantial investment.
- Miniaturization: Smaller and lighter sensors can be fitted in more places, including personal devices, improving accessibility to pollution data.
One notable example is the use of low-cost air quality sensors, like those developed by companies such as PurpleAir. These devices create networks that gather localized air quality data, which can be used by communities to make informed choices regarding their health and environment. This transition to lower-cost, high-accuracy sensors aids not only academia but local communities as well.
Artificial Intelligence and Machine Learning Applications
Artificial Intelligence (AI) and Machine Learning (ML) are becoming essential in the analysis of pollution data. These technologies process vast amounts of data and identify patterns that may not be immediately visible. Applications include:
- Predictive Analytics: AI algorithms predict pollution trends based on historical data, enabling proactive measures to mitigate impacts.
- Data Integration: Machine learning simplifies and enhances the integration of diverse data sources, improving the usability of pollution data for research and policy.
- Automated Analysis: With AI, the analysis of large datasets can be automated, making it faster and more efficient.
"Technological innovations, particularly in sensors and AI, are reshaping our approach to monitoring pollution and its impact on health and policy."
In the context of pollution analysis, these innovations represent a crucial leap forward, providing the tools necessary to confront the pressing environmental challenges of today.
Case Studies in Pollution Data Analysis
Case studies in pollution data analysis are critical. They provide concrete examples of how data can inform our understanding of pollution and its impacts. These studies illustrate real-world applications of data collection and analysis methods. They also demonstrate how findings can shape policies, drive community action, and inform public health decisions.
By examining specific instances of pollution assessment, researchers can identify patterns and trends that are not always apparent in broader datasets. This localized analysis enhances our understanding of environmental factors that contribute to pollution. The benefits of case studies include the ability to gather in-depth data, engage stakeholders, and inform preventative measures.
Additionally, case studies facilitate knowledge sharing between regions with similar conditions. They provide a valuable learning framework for future research and policy development, showcasing both successful strategies and challenges encountered. Here are two noteworthy examples focusing on urban air quality and agricultural water quality:
Urban Air Quality Assessments
Urban areas often face significant air quality issues. Evaluating these issues through case studies helps provide insights into pollutants, sources, and health outcomes. For instance, a study in Los Angeles explored variations in air pollutants like nitrogen dioxide and particulate matter across neighborhoods. They deployed sensors strategically throughout the city.
This assessment revealed strong correlations between vehicle traffic and higher pollution levels. Key findings showed that disadvantaged communities often bore the brunt of poor air quality. As a result, local authorities were encouraged to implement transportation policies aimed at reducing emissions.
Such studies can lead to practical solutions. By analyzing air quality data, cities can develop targeted interventions like tree planting initiatives. They can also enhance public transportation systems. This not only improves air quality but promotes better public health outcomes for urban residents.
Water Quality Modeling in Agricultural Areas
Agricultural regions present unique challenges for water quality assessment. In areas heavily reliant on farming, activities can lead to nutrient runoff into water systems. One prominent case study focused on the Chesapeake Bay. Researchers examined the impact of agricultural practices on water quality.
Using a combination of field monitoring and modeling techniques, they identified sources of pollution. The study highlighted how excess fertilizer led to algal blooms, which suffocated aquatic life. Key stakeholders included farmers, environmentalists, and policymakers, who collaborated to devise solutions.
As a result of the analysis, conservation practices were implemented, such as cover cropping and buffer zones. These measures helped mitigate nutrient runoff. This case study exemplifies how localized water quality modeling can lead to significant environmental improvements and sustain agricultural productivity.
Understanding these case studies shapes how we approach pollution analysis. They reflect the importance of context in developing effective strategies to combat pollution. As we move forward, incorporating additional case studies could enhance our comprehension of pollution dynamics and foster innovative solutions.
Implications of Pollution Data Analysis
The implications of pollution data analysis extend beyond the immediate findings of studies. They influence regulatory policies, public health responses, and broader environmental strategies. Through effective data analysis, stakeholders gain insights that inform decision-making processes. Such analysis often identifies pollution trends, allowing authorities to understand the sources and effects of contaminants.
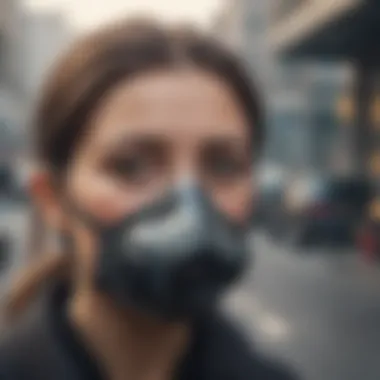
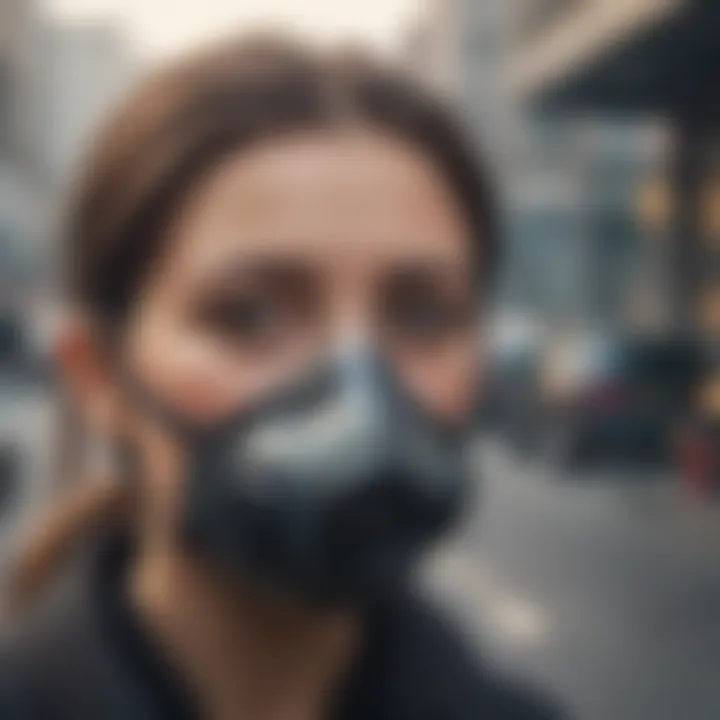
Policy Development and Environmental Regulation
Data-driven policymaking is crucial for effective environmental regulation. Reliable pollution data informs legislation aimed at minimizing ecological harm. For example, air quality data can lead to stricter emissions standards for industries that contribute to urban smog.
The importance of sound policy development includes:
- Establishing realistic environmental goals
- Understanding the effectiveness of current regulations
- Identifying areas needing stricter oversight
Moreover, transparent data collection promotes public trust. When citizens see that regulations are based on sound analysis, their confidence in government actions increases. Therefore, open access to pollution data not only aids in policymaking, but it also engages communities in environmental protections.
Public Health Considerations
Pollution has a direct link to public health. Analyzing data related to pollutants gives insight into the potential health risks communities face. Health outcomes associated with air quality, for instance, can often be predicted through data analysis. Chronic illnesses, respiratory diseases, and even impacts on mental well-being can be traced back to pollution exposure.
Key public health considerations include:
- Health risk assessments: Identifying vulnerable populations that may be disproportionately affected by pollution.
- Prevention measures: Using data to design targeted interventions before health issues arise.
- Long-term health tracking: Monitoring how policy changes affect community well-being over time.
"The link between pollution and health is clear, and data analysis serves as our primary tool to understand that connection more deeply."
In summary, the implications of pollution data analysis are profound. They unlock the potential for informed policymaking and safeguard public health. As experts continue to refine techniques, the outcomes of such analyses promise to protect both our environment and society.
Future Trends in Pollution Data Analysis
Understanding the future trends in pollution data analysis is crucial for multiple reasons. First, it allows researchers and policy makers to anticipate environmental changes and their implications. Second, staying informed about emerging technologies helps improve data collection methods and analytical techniques, ensuring reliable results. Such knowledge is essential for addressing the ongoing pollution crisis effectively.
Integrating Big Data Solutions
The integration of big data solutions into pollution analysis signifies a major turning point. Large datasets from various sources, including satellite imagery and IoT devices, provide comprehensive insights into pollution patterns. By harnessing big data analytics, environmental professionals can uncover trends that were previously hidden due to data limitations.
Key benefits of integrating big data include:
- Enhanced Monitoring: Continuous data collection leads to real-time monitoring of pollution levels.
- Informed Decision Making: Policymakers can make better decisions based on robust data analysis.
- Predictive Insights: Data-driven forecasting can identify potential pollution hotspots before they arise.
However, challenges also exist. Data privacy concerns, the need for standardized data formats, and ensuring data quality must be accounted for. Stakeholders need to work collaboratively to address these issues, creating a more structured environment for data sharing.
The Role of Citizen Science
Citizen science plays an increasingly significant role in pollution data analysis. Individuals contribute to data collection efforts through mobile applications and sensors, thus multiplying the amount of information available for analysis. This grassroots involvement empowers communities, fostering a sense of ownership in local environmental decisions.
Advantages of citizen science include:
- Broader Data Coverage: More data points across varied locations can reveal localized pollution issues.
- Community Engagement: It raises public awareness about environmental challenges, encouraging proactive behavior.
- Cost-Effectiveness: Engaging citizens can reduce the costs associated with traditional data collection methods.
"Citizen science offers a unique opportunity to bridge the gap between experts and the public while enhancing the depth and breadth of data available for analysis."
Yet, the credibility of citizen-contributed data must be assessed critically. Establishing guidelines and best practices will ensure data accuracy. Training and support can improve the quality of citizen science contributions, maximizing its potential for impactful analysis.
In summary, the future of pollution data analysis relies heavily on integrating big data solutions and harnessing the power of citizen science. These trends not only enhance data quality but also engage the community in environmental stewardship. Recognizing these developments is essential for effective pollution monitoring and remediation strategies.
Culmination
The importance of the conclusion in this article lies in summarizing the extensive exploration undertaken regarding pollution data analysis. Through various sections, we identified significant trends and challenges, while showcasing innovative solutions. This section serves as a reflective synthesis of the complexities inherent in understanding how pollution affects our environment and public health.
Key findings highlighted throughout the article underscore the pressing need for reliable data collection methods, advanced analytical technologies, and the collaboration of policymakers with scientists. A comprehensive conclusion encapsulates these insights. It cements how pollution affects diverse ecosystems and human health, thereby enhancing the urgency for robust analysis and timely responses.
"Data analysis in pollution is not just about numbers; it signifies the foundational step towards sustainable environmental practices."
Furthermore, the conclusion promotes a holistic view of the topic. It clarifies how understanding pollution patterns can lead to better policy formulation, informed public health decisions, and community engagement in environmental initiatives. By synthesizing findings into actionable insights, this article aims to stimulate further discussions on pollution and data analysis.
Summary of Key Findings
In reviewing the findings presented, it is clear that the integration of advanced data analysis techniques is crucial. The key points are:
- Diversity of Pollution Types: Various pollution types, including air, water, and soil, present distinct challenges and data collection needs.
- Importance of Data Quality: Reliable data is essential for making informed conclusions about pollution trends and impacts.
- Technological Advances: Innovations in remote sensing and machine learning provide powerful tools to enhance data analysis capabilities.
- Collaboration Across Sectors: The intersection of public health and environmental policy requires shared understanding and cooperation among different stakeholders.
Recommendations for Future Research
Future research should focus on the following areas to further enhance pollution data analysis:
- Data Standardization: Develop standardized protocols for data collection and sharing. This will improve the interoperability of data from multiple sources.
- Longitudinal Studies: Conduct long-term studies to track changes over time. Understanding long-term trends will help mitigate future pollution challenges.
- Community Engagement: Research the role of citizen science in data collection to foster local involvement and awareness within communities.
- Impact Assessment: Explore the direct impact of pollution on public health, particularly in vulnerable populations, to strengthen the correlation between data and policy.
These recommendations could lead to more comprehensive insights and solutions, ultimately enabling a proactive approach to managing pollution.