Datasets Transforming Medical Diagnosis and Healthcare

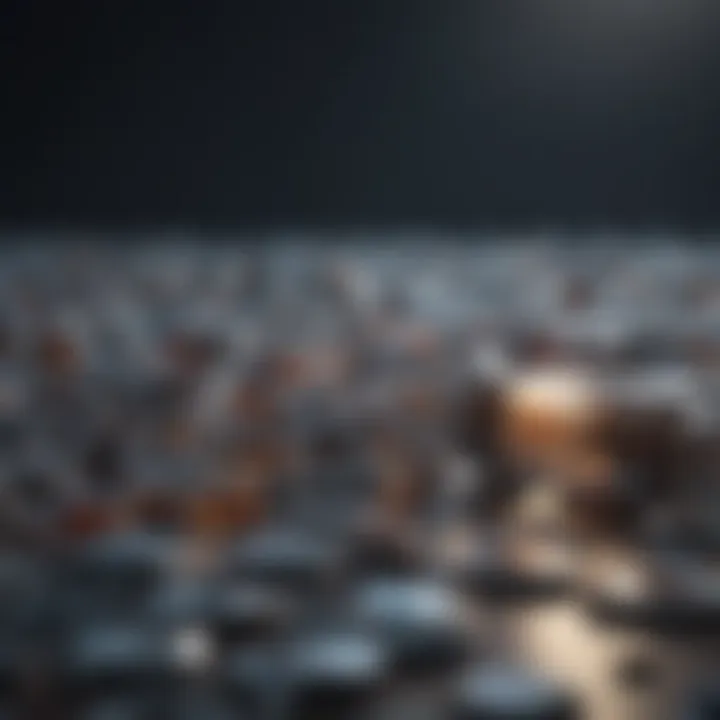
Intro
In the modern healthcare landscape, datasets are the backbone of trustworthy medical diagnosis. They serve as the foundation for machine learning algorithms, clinical studies, and evidence-based practices. Whether it's predicting disease outcomes, enhancing diagnostic precision, or personalizing treatment plans, datasets hold immense power to redefine patient care.
The significance of these datasets cannot be overstated. They are complex not just in their structure, but in how they are created, managed, and utilized. From chirpy novice researchers to seasoned professionals, understanding the role and challenges of these data resources is essential.
As we move forward, this article will dissect the various aspects of medical datasets, revealing the nuts and bolts of their creation, the methodologies employed, and the ethical implications surrounding their use.
Research Overview
Summary of Key Findings
Let's kick things off with a broad look at why datasets matter in medical diagnosis. A few key points emerge:
- Enhanced Diagnostic Accuracy: Properly curated datasets can significantly boost the accuracy of diagnoses, reducing the chances of misdiagnosis.
- Diverse Applications: They are used in a plethora of scenarios, from imaging studies to genomics, enabling dynamic patient management strategies.
- Tailored Medicine: The use of datasets allows for more personalized treatment options based on individual patient backgrounds and disease markers.
Importance of the Research
Exploring medical datasets provides essential insights into how healthcare systems can evolve. The implications are wide-reaching:
- Healthcare Efficiency: By leveraging data correctly, healthcare providers can enhance workflow and improve patient outcomes.
- Informed Decision-Making: Accurate datasets enable clinicians to make more informed choices based on evidence rather than intuition alone.
- Future Innovations: As technology progresses, understanding dataset dynamics can fuel innovations in diagnostics and treatment approaches.
Methodology
Study Design
To dissect the effectiveness of medical datasets, a comprehensive review methodology is employed. This includes analyzing existing literature, examining case studies, and synthesizing data from varied sources to draw meaningful conclusions. A multi-faceted approach ensures that the research covers various dimensions of dataset utility in diagnostics.
Data Collection Techniques
When it comes down to collecting data, various methods are utilized:
- Surveys and Questionnaires: Gathering qualitative data from healthcare professionals and patients can yield deep insights.
- Clinical Trials: These provide robust datasets that are often used for developing new therapies and treatments.
- Historical Medical Records: Analysis of past patient data can inform current practices and uncover trends over time.
Utilizing these techniques not only enhances the robustness of the findings but also provides a clear lens through which the evolving dynamics of medical diagnosis can be observed.
"In a world increasingly driven by data, recognizing the potential of medical datasets is not just an option; it's a necessity."
With this foundation laid, the subsequent sections will further elaborate on the challenges associated with dataset utilization and the ethical considerations that accompany their application in medical practice.
Prolusion to Medical Datasets
The landscape of healthcare is rapidly changing, driven by advancements in technology and data science. Central to this evolution are medical datasets, essential tools that enhance our understanding of health conditions, improve diagnosis accuracy, and enable tailored treatment plans. Without these datasets, healthcare practitioners would be navigating the complex realm of patient care with little more than anecdotal evidence and intuition.
Importance of Datasets in Healthcare
Datasets are the backbone of modern healthcare analytics. They compile vast amounts of information from various sources, providing insights that help drive decision-making processes.
- Improved Diagnostic Accuracy: Reliable datasets allow physicians to identify patterns and correlations that might not be visible at first glance. For example, analyzing a large dataset of patient records can highlight which symptoms often precede a particular diagnosis, leading to quicker and more accurate assessments.
- Enhanced Research Capabilities: Medical research has profoundly benefited from access to extensive datasets. Researchers can identify trends over time, study genetic factors affecting diseases, and assess the efficacy of new treatments across diverse populations. The fruits of this endeavor lie in the more effective therapies and interventions that emerge from robust data analysis.
- Resource Allocation: Hospitals and healthcare organizations can utilize datasets to optimize resource distribution. By analyzing patient data, facilities can predict patient volume, allowing them to staff appropriately, stock necessary supplies, and ultimately improve patient care.
The significance of datasets in healthcare cannot be overstated; they serve as a compass in uncertain waters, guiding health professionals toward better outcomes.
Historical Context and Evolution
The journey of medical datasets is intertwined with the evolution of medical practice itself, tracing back to the days of rudimentary record-keeping.
Initially, patient information was stored in physical files, lacking any standardized protocol. As medicine grew more complex, the need for systematic data representation became apparent. With the advent of electronic health records in the late 20th century, a revolution began. This electronic transition allowed for easier storage, retrieval, and sharing of patient information. Today, we witness an explosion of data inflow, driven by numerous modalities - from wearable devices to clinical trials.
- From Paper to Pixels: The shift from analog records to digital systems was monumental. Early datasets were often fragmented, with healthcare providers relying on disparate software solutions that limited data sharing. This created silos that hindered collaborative care.
- Integration and Interoperability: The push for standardized formats and interoperability among systems has enabled comprehensive data sharing. When data from various sources—such as diagnostic imaging, lab results, and genetic screenings—are pooled together, it delivers a thorough picture of a patient.
- Current Trends: Recently, the integration of big data analytics and artificial intelligence is reshaping the dataset landscape. These technologies hold the promise of predictive analytics, enabling healthcare providers to foresee issues before they arise, thus paving the way for preventative medicine.
The evolution of medical datasets reflects the ongoing transformation of healthcare itself—aimed at improving outcomes, enhancing the accuracy of diagnoses, and personalizing treatment protocols.
Types of Medical Datasets
The realm of medical datasets is vast and incredibly diverse, encompassing a variety of data types that serve distinct yet interconnected functions. Each category of dataset possesses unique characteristics and potential applications in the field of medical diagnosis. Understanding these types is crucial for students, researchers, educators, and professionals who seek to leverage data for improved patient care and efficacious diagnostic processes. The following sections delve into specific types of datasets, shedding light on their importance in the medical community.
Clinical Data Datasets
Clinical data datasets form the backbone of evidence-based medicine, providing critical insights into patient demographics, clinical traits, treatment responses, and outcomes. These datasets are democratized snapshots of patient encounters, collected through clinical trials, observational studies, and daily practice. The richness of clinical data lies in its ability to facilitate tailored treatment options and improve outcomes.
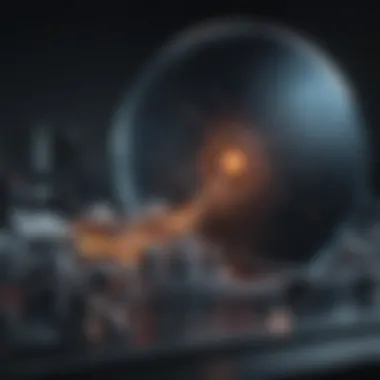
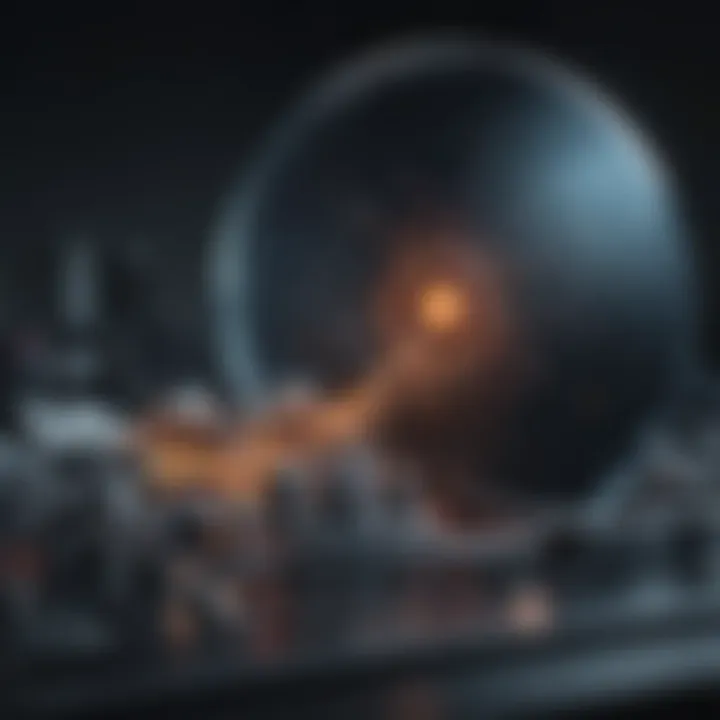
Key benefits include:
- Enhancing the precision of diagnostic tools
- Informing clinical guidelines through aggregated patient data
- Driving research on drug efficacy and treatment protocols
However, leveraging clinical data presents challenges, such as data consistency and the need for vigilant data stewardship to safeguard patient confidentiality and integrity. An example can be drawn from various databases like SEER (Surveillance, Epidemiology, and End Results) which provide key information on cancer incidence and survival, assisting oncologists in making informed decisions.
Genomic and Genetic Information
The advent of genomic medicine has revolutionized diagnostics by enabling a deeper understanding of the genetic underpinnings of diseases. Genomic datasets include sequences of DNA, RNA, and proteomic information, facilitating the identification of mutations linked to specific conditions. By employing technologies like next-generation sequencing, researchers can parse complex data to unveil relationships between genes and diseases, paving the way for personalized treatment strategies.
Such datasets are invaluable in understanding hereditary conditions, screening for predispositions to diseases, and tailoring therapeutic interventions based on an individual’s genetic makeup. However, they also raise ethical questions regarding patient consent and data usage which can not be overlooked.
Some notable examples of genetic databases include The Cancer Genome Atlas (TCGA) and the Genome Aggregation Database (gnomAD).
Imaging Data and Radiology
Medical imaging datasets encompass a wealth of visual data derived from various imaging modalities such as MRI, CT scans, and X-rays. These datasets are crucial for training AI models that can detect anomalies and assist radiologists in diagnostics. The key advantage of imaging data is its ability to provide visual context, which is often more interpretable than numerical data alone.
For instance, large-scale imaging databases have been established to foster research aiming at early stage detection of ailments such as lung cancer or Alzheimer’s disease.
Applications of imaging data include:
- Automated detection of tumors
- Disease progression tracking
- Enhancement of diagnostic accuracy through advanced image analysis techniques
The challenge remains in ensuring quality and consistency across images, as variations in technique, devices, or operator skill can inflate diagnostic discrepancies.
Electronic Health Records (EHR)
Electronic Health Records serve as comprehensive repositories of patient data collected over time. Unlike clinical datasets that focus on specific episodes of care, EHRs compile longitudinal data encompassing demographics, medical history, prescriptions, lab results, and physician notes. This holistic view allows for more thorough monitoring of patient health and makes it significantly easier to identify trends over time.
Benefits of EHRs include:
- Improved coordination of care through accessible patient information
- Enhanced clinical decision-making
- Reducing redundancies in testing
Nevertheless, the sheer volume of data can lead to challenges in extraction, privacy concerns, and potential issues with data integrity which must be navigated carefully. Furthermore, interoperability between different EHR systems remains a topic of significant discussion and development.
"The ability to aggregate and analyze data from EHRs allows healthcare providers to make informed decisions that significantly affect patient outcomes."
In summary, these diverse datasets—clinical data, genomic information, imaging data, and EHRs—are instrumental in propelling medical diagnostics forward. Each type not only enriches our understanding of various health issues but also enhances the capability of healthcare professionals to provide accurate, timely, and personalized patient care. The continuing evolution of these datasets can ultimately lead to breakthroughs in modern medicine.
Methods of Dataset Creation
Creating a medical dataset is not merely a mechanical process; it is an intricate dance of methodologies that can profoundly influence the outcomes of medical diagnoses. In this section, we dissect the various methods of dataset creation, emphasizing why these approaches are vital not only for collection but also for ensuring quality and reliability. The pursuit of medical excellence is intertwined with the art and science of converting raw data into actionable insights through structured datasets.
Data Collection Techniques
When it comes to gathering data, the methods chosen can shape the entire dataset. There are several ways to collect data, each with its pros and cons:
- Surveys and Questionnaires: These are often used to gather information directly from patients regarding their symptoms, medical history, and health status. Though easy to administer, they can introduce bias if not crafted carefully.
- Clinical Trials: This method involves collecting data from structured experiments or studies where participants receive specific treatments. Clinical trials yield high-quality data, but they are resource-intensive.
- Observational Studies: Here, researchers gather data without intervention. Although this method allows collection of real-world data, it can be challenging due to confounding factors.
- Wearable Devices: Technology allows for continuous monitoring of patient health metrics. Data from these devices can be real-time and vast, but reliability is dependent on device accuracy.
The chosen method of data collection impacts the dataset's quality and the results derived from it. Careful consideration of these techniques forms the foundation for robust datasets.
Data Annotation and Labeling
Once data is collected, the next step revolves around its annotation and labeling. This is an essential phase, particularly for training machine learning models, where labeled data acts as a teacher. Effective labeling allows algorithms to learn the patterns behind conditions accurately.
To enhance data relevance and utility, several strategies can be employed:
- Expert Review: Having medical professionals label the data ensures that the information is precise and relevant.
- Crowdsourcing: This method utilizes the collective intelligence of a large group, but quality control becomes paramount to avoid inaccuracies.
- Automated Labeling: While faster, relying solely on automated processes can introduce errors, especially if the algorithms miss nuanced details.
The success of a dataset largely depends on this annotation process since mislabeled data can lead to faulty conclusions and poor diagnostic predictions.
Data Normalization and Preprocessing
After data labeling, normalization and preprocessing must be conducted. These steps involve transforming raw data into a consistent format that can be effectively used for analysis. While this might sound technical, it is crucial for maintaining the integrity and usability of the dataset.
Normalization involves scaling data, adjusting for variations, and eliminating bias. Techniques vary, including:
- Min-Max Scaling: Adjusting the data to a fixed range, typically 0 to 1. This method works well for ensuring uniformity.
- Z-score Normalization: This approach standardizes data based on the mean and standard deviation, which is valuable when dealing with outliers.
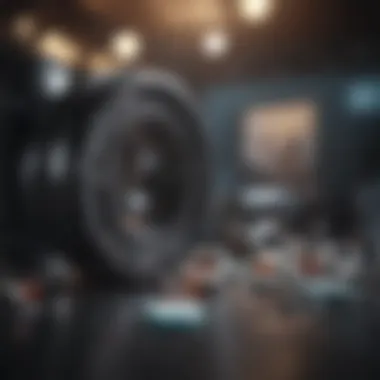
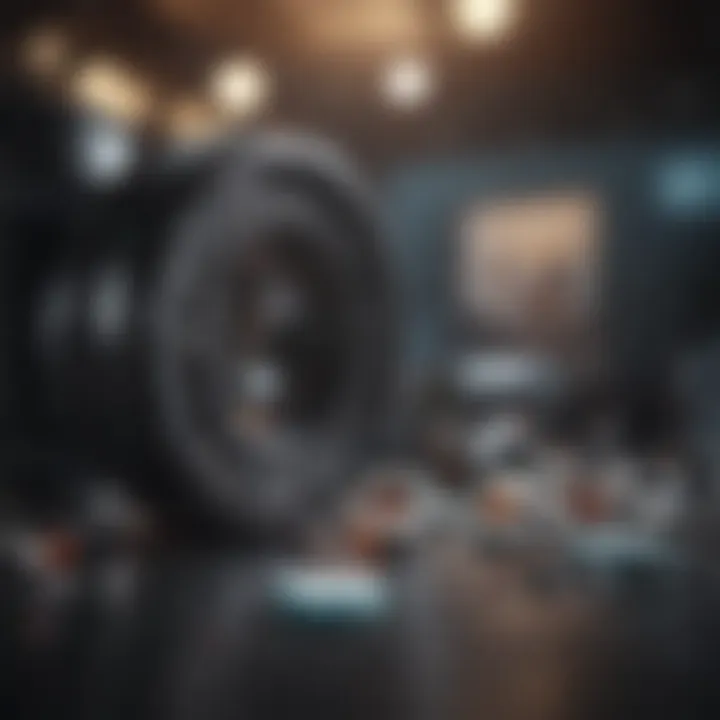
Preprocessing, on the other hand, encompasses handling missing values, removing duplicates, and addressing irrelevant features. Failure to properly preprocess data can severely distort findings and misinform diagnosis.
"Quality data is the bedrock of reliable medical diagnosis, and the process of collection, annotation, and normalization is where the magic truly happens."
Closure
In summation, understanding methods of dataset creation is critical for anyone involved in medical diagnostics. Each phase from data collection to normalization plays a pivotal role in shaping the final dataset. Adopting meticulous techniques ensures that datasets are not only rich in information but also reliable for extracting meaningful clinical insights.
Applications of Medical Datasets
The increasing relevance of medical datasets in diagnostic practices cannot be overstated. They are the backbone of modern healthcare analytics, enabling the processing of vast quantities of information that contribute to patient care decisions. With advancements in technology, the applications of these datasets have expanded significantly, allowing healthcare professionals to leverage data in innovative ways. The main advantage lies in the potential for improved diagnostic accuracy, which can ultimately lead to better patient outcomes.
Machine Learning in Diagnostics
Machine learning, a field of artificial intelligence, leverages medical datasets to train algorithms capable of identifying patterns and making predictions. This approach is particularly valuable in diagnostic settings where precision is critical. Utilizing datasets like those collected from imaging studies, such as x-rays or MRIs, algorithms can discern nuances that might escape even the most seasoned radiologists.
For instance, algorithms have been designed to detect early signs of diseases such as cancer. When trained with thousands of previously diagnosed cases, these machine learning models learn not just to identify clear indicators but also subtle anomalies that signal potential issues. This can significantly reduce false negatives, ensuring that conditions are caught at an earlier stage, when they are more treatable.
Moreover, machine learning can support decision-making by prioritizing cases based on urgency. With the right datasets, healthcare professionals can receive alerts for critical cases, streamlining their workflow and allowing them to allocate resources more effectively. The importance of these models in diagnostic procedures cannot be ignored; they essentially act as a second pair of eyes, providing extra assurance in the fast-paced world of healthcare.
Predictive Analytics in Healthcare
Predictive analytics, a cousin of machine learning, harnesses medical datasets to forecast future events related to patient health. By examining historical data, healthcare practitioners can anticipate complications before they arise, significantly improving patient management strategies. For example, a hospital might analyze patterns of patient readmissions within its dataset, identifying at-risk populations and implementing targeted interventions.
There are clear benefits to employing predictive analytics:
- Proactive Care: Anticipating potential health issues can lead to early interventions, such as lifestyle changes, which might prevent more severe problems.
- Resource Optimization: By understanding trends in patient flow, hospitals can better allocate resources, reduce waiting times, and streamline service delivery.
- Improved Outcomes: Clinicians are equipped with valuable insights, ultimately leading to a higher standard of patient care.
However, leveraging predictive analytics is not without challenges. Data quality and completeness remain significant hurdles, as any errors in datasets can lead to misguided predictions. Therefore, constant validation and refinement of the input data are essential for the accuracy of predictions.
Clinical Decision Support Systems
Clinical Decision Support Systems (CDSS) are tools designed to assist healthcare providers in making informed decisions. These systems rely heavily on medical datasets. They analyze the data and offer evidence-based recommendations tailored to individual patient cases. Such systems help mitigate human error, especially in complex clinical environments.
One prominent example is the integration of clinical guidelines and patient data to suggest diagnostic tests or treatments. If a doctor is unsure whether to prescribe a specific medication, a CDSS can analyze patient history and current health conditions to produce recommendations based on the latest research.
The benefits of CDSS include:
- Enhanced Decision-Making: By providing real-time access to clinical guidelines and past patient data, these systems serve as an invaluable resource for clinicians.
- Increased Efficiency: They reduce the time spent on research and allow more focus on patient interaction.
- Personalized Treatment Plans: CDSS tools can tailor recommendations based on a patient’s unique history and conditions, leading to more effective care.
Nonetheless, reliance on these systems brings forth ethical considerations, such as maintaining patient data privacy. Ensuring that the data is used responsibly and ethically is paramount in the deployment of these systems across healthcare settings.
"The effectiveness of machine learning, predictive analytics, and clinical decision support systems hinges on the quality of the datasets they rely on. Such tools not only enhance diagnostic accuracy but also optimize overall healthcare delivery."
In summary, the applications of medical datasets—ranging from machine learning algorithms to predictive analytics and clinical decision support systems—highlight their role as critical tools in modern healthcare. They provide substantial benefits that improve diagnostic accuracy, promote patient safety, and elevate the standard of care.
Challenges in Utilizing Medical Datasets
The world of medical datasets is layered with complexities that can complicate their practical application. While these datasets are powerful tools that can enhance diagnosis and treatment, they come with a spectrum of challenges that must be navigated with caution. Each of these challenges—data quality, access and availability, and algorithm limitations—serves as a reminder that while data can be a friend, it also has its quirks and pitfalls. Understanding these challenges is imperative for anyone involved in healthcare technology, as they directly affect the efficacy and reliability of medical diagnoses.
Data Quality and Completeness
Data quality is the bedrock of effective medical datasets. If the data is flawed, incomplete, or out-of-date, the conclusions drawn from it can lead to misguided decisions. Inaccuracy can stem from several sources: human errors during data entry, inconsistencies in measurements, or even outdated protocols used for data collection. The hefty price of poor quality data can manifest in diagnostic inaccuracies, potentially leading to incorrect treatments and disastrous patient outcomes.
Histories of inconsistent clinical coding and variability in patient reporting also contribute to gaps in completeness. For instance, suppose a patient presents with vague symptoms that are not adequately documented in the Electronic Health Record. If the medical dataset lacks a comprehensive view, healthcare providers may miss crucial diagnostic clues. Ultimately, the quality of data not only affects individual diagnoses but can also skew large-scale research outcomes, making it a significant hurdle in the utilization of medical datasets.
Access and Availability Issues
Access to medical datasets is another complex issue, often shaped by legal, ethical, and logistical challenges. Particularly in the realm of patient data, privacy regulations like HIPAA in the United States enforce strict guidelines regarding who can access and utilize health information. While these regulations are essential for protecting patient privacy, they can limit researchers' abilities to gather comprehensive datasets.
Moreover, even when legal barriers are lifted, logistical hurdles can still impede progress. For example, datasets might be stored in disparate systems with varying formats, making it difficult for researchers to combine them for analysis.
The result? Researchers may find themselves working with isolated pockets of data rather than a holistic view that could greatly enhance their studies. This fragmentation can stifle innovation, leaving industry players scrambling for quality datasets when every second counts.
Computer Algorithm Limitations
Lastly, the effectiveness of medical datasets is also tied to the algorithms used to analyze them. As advances in machine learning and artificial intelligence continue to reshape healthcare technology, algorithms must evolve in tandem to keep pace. Unfortunately, many existing algorithms possess inherent limitations that can hinder their performance, especially in diverse patient populations.
Take, for instance, the issue of training datasets that lack adequate representation of different demographics. When algorithms are trained primarily on data from one group, they may not perform well on others, leading to biased results. This limitation underscores the need for diversified datasets that mirror real-world demographic complexities.
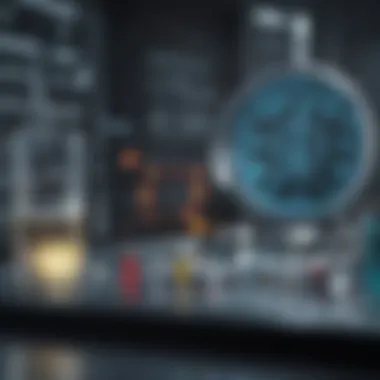
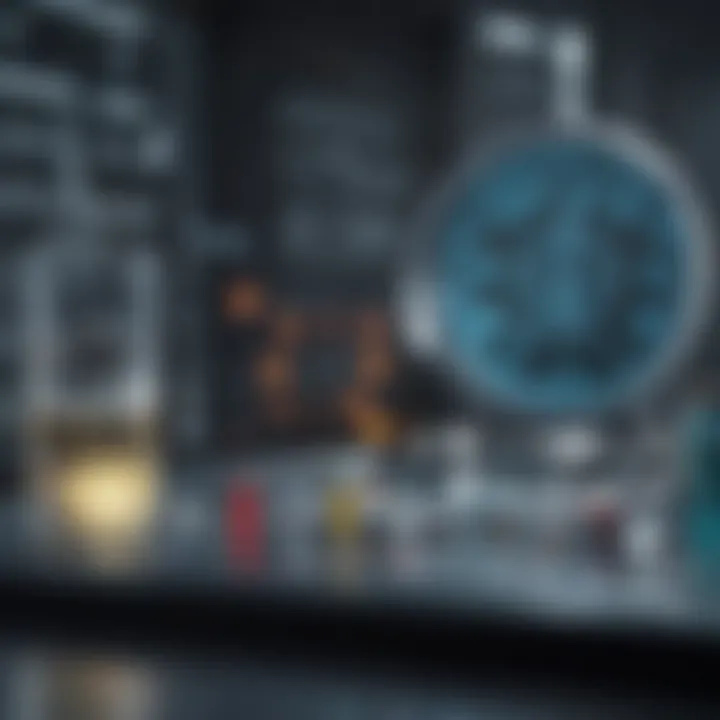
"The effectiveness of a data-driven approach hinges significantly on the underlying algorithms and the diversity of datasets used."
As we navigate the challenges of utilizing medical datasets, it's crucial to recognize that addressing these issues is not just about overcoming obstacles; it's about enhancing the quality of care delivered to patients. Awareness and strategic planning can lead to solutions that harness the full potential of medical datasets and facilitate better outcomes in diagnosing and treating diverse health conditions.
Ethical Considerations in Medical Datasets
The ethical implications surrounding medical datasets are not just footnotes in a discussion; they are foundational. As the landscape of healthcare shifts with the influx of big data and artificial intelligence, deciding how to handle this information becomes a pressing concern. Striking a balance between innovation and ethical responsibilities is vital. These considerations affect not only the patients whose data is being utilized but also the integrity of the healthcare system itself.
Patient Privacy and Data Security
In today's digital age, safeguarding a patient's privacy should be regarded as non-negotiable. When medical datasets contain sensitive personal information, unauthorized access could lead to dire consequences for individuals.
"Data breaches can erode trust between patients and healthcare providers, making individuals wary of sharing vital health information."
To address these concerns, the implementation of stringent security measures is paramount. Hospitals and research facilities must adopt advanced encryption and anonymization techniques to ensure that personal data is protected. Moreover, educating healthcare professionals about data privacy laws, such as HIPAA in the United States, is essential to bolster compliance and reinforce ethical standards.
Bias and Fairness in Data
Handling datasets is like walking a tightrope; one misstep, and bias can seep in. This is especially true when certain demographics are underrepresented in medical research. This can lead to algorithms that are only good for a subset of the population, leaving others vulnerable. For instance, if a dataset primarily includes data from one ethnic group, the resulting analyses may not be applicable or accurate for others.
To foster fairness, developers must ensure diverse representation in their datasets. This can be accomplished by collecting data from varied demographics. Regular audits of algorithms and outcomes should also be enforced to identify and address any biases that emerge over time. As more healthcare professionals become aware of these biases, the calls for equity in data usage will grow louder, pushing the industry toward a more inclusive practice.
Regulatory Framework and Compliance
Compliance with established regulations is another crucial aspect when working with medical datasets. Following the legalities surrounding data utilization not only safeguards patients but builds a framework for ethical research practices.
Different regions have varying regulatory frameworks that govern data usage. For example, the European Union has enacted the General Data Protection Regulation (GDPR), which sets strict guidelines about how personal information must be handled. In contrast, countries like the USA have their own mix of federal and state regulations.
Adhering to these guidelines ensures that data is used responsibly. Organizations should routinely review and update their policies to ensure they are in line with current regulations and best practices. Establishing a culture of compliance can significantly mitigate the risk of legal repercussions while promoting ethical standards in data management.
In summary, the ethical considerations in medical datasets cannot be overlooked. Addressing patient privacy, mitigating bias, and ensuring regulatory compliance are all integral to the responsible use of data in healthcare. As we advance into a future where data and diagnostics increasingly intertwine, fostering an ethical landscape will ensure that innovation does not come at the cost of integrity.
Future Trends in Medical Datasets
The landscape of medical datasets is evolving rapidly, propelled by advancements in technology and data analytics. Understanding the future trends in this domain is crucial, not just for professionals in healthcare, but also for researchers and educators striving to harness these tools for improved patient outcomes. As data technology becomes further integrated into healthcare systems, we witness a plethora of benefits, informing diagnostic practices and enhancing overall patient care. Here, we unravel key elements shaping the future of medical datasets and consider the corresponding implications.
Integration of AI and Big Data
The marriage of artificial intelligence (AI) and big data has placed a powerful emphasis on predictive capabilities within medical datasets. With vast amounts of health data being generated daily, from wearables to machine-generated diagnostics, the bulk of this information can be overwhelming. However, AI serves as a maestro in orchestrating this data, identifying patterns that could go unnoticed by human eyes.
- Enhanced Diagnostic Accuracy: AI algorithms can sift through immense datasets to aid in diagnosing conditions earlier, like detecting early signs of diseases such as Alzheimer’s or various cancers, highlighting anomalies that may not be apparent in standard clinical assessments.
- Real-world Applications: Machine learning models can be developed to predict patient outcomes based on historical data, thus guiding clinicians in making more informed decisions tailored to individual patient profiles.
- Continuous Learning: Once deployed, these algorithms continue to adapt and learn, improving their accuracy and applicability in real-time.
"AI empowers healthcare professionals, helping transform insights into action."
Personalized Medicine and Tailored Datasets
Personalized medicine is the cornerstone of modern healthcare, enabling tailored treatments based on an individual’s unique genetic makeup or health history. Datasets designed with this personalization in mind offer a profound opportunity for better patient engagement and outcomes.
- Genetic and Phenotypic Data: The advent of genomic testing allows healthcare providers to access specific genetic information. Coupled with electronic health records (EHRs), this data breeds a potent mix for crafting customized treatment plans that account for both hereditary and environmental factors.
- Patient-Centric Data Creation: The move towards patient-reported outcomes adds depth to these datasets, allowing patients to have a voice in the treatment process based on their experiences and preferences.
- Benefits of Tailored Approaches: Tailoring treatments not only enhances efficacy but also minimizes adverse effects, as interventions are designed to align with the patient’s profile.
Real-time Data Monitoring and Analysis
The future of medical datasets also promises more robust real-time monitoring systems that can actively analyze patient data as it comes in. This real-time capability is set to revolutionize healthcare delivery and diagnostics.
- Wearable Technology Integration: Wearables can track vital signs continuously, transmitting this information to centralized databases. Here, data can undergo immediate analysis to identify potential health issues as they arise, giving providers a chance to act before a condition escalates.
- Emergency Response: Timely alerts generated from monitoring systems can initiate preventive measures, ensuring critical care is provided without delay.
- Streamlining Healthcare Operations: Hospitals can leverage these datasets for operational analytics, leading to more efficient resource allocation, maximizing patient care, and minimizing costs.
Culmination
In a rapidly evolving healthcare landscape, the significance of medical datasets cannot be overstated. As this article has illustrated, datasets serve as the backbone of medical diagnostics, providing a foundation for clinical decision-making and enhancing patient outcomes. Their role is not just instrumental; it's pivotal in enabling healthcare professionals to access comprehensive information quickly and efficiently.
Summarizing Key Insights
To wrap up the central ideas discussed in this comprehensive exploration:
- Crucial Role in Diagnosis: Datasets enrich the diagnostic process, allowing for more accurate identification of conditions through data-driven insights.
- Variety and Complexity: Different types of datasets—ranging from clinical observations to genomic information—offer diverse perspectives that are essential for holistic patient care.
- Methodologies and Challenges: Creating and maintaining these datasets involves meticulous processes like data collection, annotation, and normalization, which come with their own unique pitfalls.
- Ethical Considerations: Protecting patient privacy and ensuring data security are not mere suggestions; they are essential in fostering public trust and compliance with regulations.
- Future Directions: The integration of artificial intelligence and personalized medicine heralds an era where datasets are not just supplementary tools but central elements in individualized patient care.
The Path Forward in Medical Data Utilization
Moving forward, there are several vital considerations that determine the trajectory of medical data utilization:
- Focus on Quality: The importance of data quality remains paramount. Ensuring datasets are accurate, complete, and representative can significantly affect diagnostic outcomes.
- Regulatory Compliance: As data landscapes evolve, so too must the frameworks governing their use. Staying abreast of new laws and regulations is essential.
- Emphasis on Diversity: Efforts must be made to include diverse populations to mitigate biases and increase the fairness of diagnostic outcomes.
- Advancing Technologies: Rapid development in AI and machine learning can enhance analysis capabilities. Engaging with these technologies will lead to better, more precise medical insights.
"Improvement in medical data utilization is a continuous journey rather than a destination. The progress made today paves the way for breakthroughs tomorrow."
The nuances explored here present a picture of a sector in motion. By strategically leveraging datasets, we not only refine our current practices but also pave the way for groundbreaking advancements in medical diagnosis. As we look ahead, embracing a collaborative mindset among healthcare practitioners, data scientists, and regulatory bodies becomes increasingly crucial to ensure that the potential of these datasets is fully realized.