Machine Learning's Impact on ECG Diagnostics
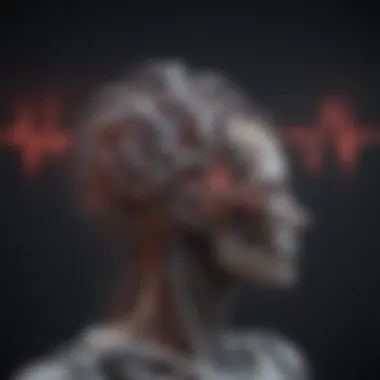
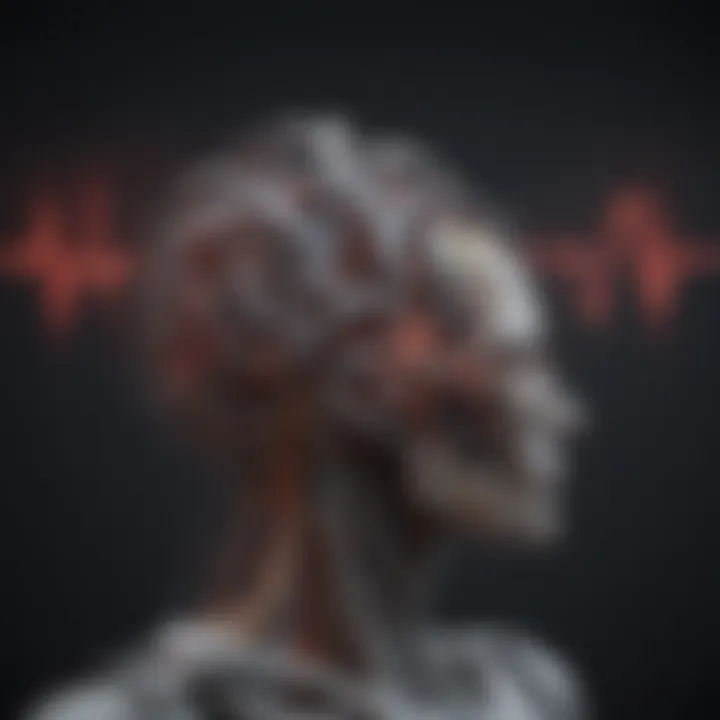
Intro
Electrocardiograms (ECGs) have long been a cornerstone in cardiology, providing critical insights into heart health. Traditional ECG analysis has relied heavily on manual interpretation by skilled clinicians. However, the rise of machine learning technology is transforming this landscape. Advanced algorithms are now analyzing vast amounts of ECG data, enhancing diagnostic accuracy and patient care.
This article investigates how machine learning integrates with ECG technology. It delves into the principles underpinning ECG analysis, the hurdles encountered in traditional methods, and the application of machine learning in interpreting ECG records. By uncovering the implications of these technologies, the narrative aims to elucidate the future of cardiovascular diagnostics and the potential impact on patient outcomes and clinical workflows.
Research Overview
Summary of Key Findings
Recent studies demonstrate that machine learning can substantially increase the precision of ECG interpretation. Key findings show that algorithms trained on extensive datasets outperform traditional analysis in identifying arrhythmias and other cardiac conditions. These insights suggest a shift towards automated systems capable of real-time monitoring and alerting clinicians of potential issues.
Importance of the Research
Understanding the integration of machine learning in ECG analysis holds significant weight in modern cardiology. This research not only aims to improve diagnostic accuracy but also addresses challenges like the shortage of cardiologists. The findings advocate for a more efficient healthcare delivery model where machine learning aids in managing both routine and complex cases.
Methodology
Study Design
The studies included in this article primarily utilize retrospective data analysis. Various machine learning models were implemented, focusing on supervised learning, where algorithms learn from labeled datasets. This approach allows for continuous improvement through training on evolving ECG data.
Data Collection Techniques
Data for these studies were collected from a variety of sources, including hospital databases and publicly available ECG datasets. Protocols ensured that the data was anonymized and standardized. Machine learning models were trained using diverse ECG records, which facilitated robust recognition of patterns associated with various cardiovascular conditions.
"Machine learning not only enhances diagnostic capabilities, it paves the way for revolutionizing patient care in cardiology."
Through this research overview and methodology, it becomes clear that the union of machine learning and ECG technology offers a promising future for cardiac diagnostics.
Prelude to ECG Technology
Electrocardiograms (ECG) are critical tools in diagnosing and monitoring cardiac health. Understanding the fundamentals of ECG technology lays the groundwork for appreciating how machine learning can enhance ECG analysis. This section articulates the significance of integrating machine learning into ECG technology, aiming to create a comprehensive view of its applications, historical context, and advancements.
Historical Development
The history of ECG technology traces back to the early 20th century. Willem Einthoven, a Dutch physiologist, is credited with the invention of the ECG in 1903. He developed the first practical electrocardiogram using an instrumentation known as the string galvanometer. This innovative technology allowed physicians to visualize the electrical activity of the heart.
Over the decades, advancements in technology have gradually improved the accuracy and accessibility of ECG equipment. The introduction of portable ECG machines in the 1960s fundamentally changed the landscape, enabling a wider array of clinical settings to utilize this diagnostic tool. The progression from analog to digital systems further refined the analysis process, increasing the precision of readings and facilitating data storage.
Present-Day Applications
Today, ECG technology serves a myriad of applications within the medical field. Hospitals routinely use ECGs for monitoring patients in critical care, aiding in the diagnosis of conditions such as arrhythmias, myocardial infarction, and electrolyte imbalances. Moreover, advances in telemedicine have allowed for remote ECG monitoring, enabling physicians to assess patients from a distance.
There is also a growing interest in wearable technologies that incorporate ECG capabilities. Devices such as smartwatches can capture and analyze heart rate data in real time, offering users insight into their cardiac health. In doing so, they empower individuals to take proactive measures regarding their health.
In conjunction with machine learning, ECG analysis is poised to reach new heights. These sophisticated algorithms can process vast datasets, identifying complex patterns that may elude traditional diagnostic methods. The synergy between machine learning and ECG technology represents a significant leap forward in enhancing diagnostic accuracy and improving patient outcomes.
Understanding the Essentials of ECG
The understanding of electrocardiograms (ECG) is crucial to the integration of machine learning in cardiovascular diagnostics. An ECG provides a visual representation of the electrical activity of the heart over time. This information is indispensable for diagnosing various cardiac conditions. Moreover, when machine learning is applied to ECG data, it enhances both accuracy and efficiency in cardiovascular care. To appreciate the benefits of machine learning in ECG analysis, it is essential to first grasp the basic principles of ECG technology.
Basic Principles of ECG
An electrocardiogram records the heart's electrical impulses using electrodes attached to the skin. These impulses result from the contraction and relaxation of the heart muscle. The common output of an ECG includes waves labeled P, Q, R, S, and T, which correspond to different phases of the heart cycle. For instance, the P wave represents atrial depolarization, while the QRST sequence corresponds to ventricular depolarization and repolarization.
Understanding the waveforms is necessary for health professionals to interpret the ECG accurately. Each segment carries significant clinical value, indicating a variety of conditions such as arrhythmias, heart block, or even ischemia. Increasingly, machine learning algorithms analyze these patterns, seeking anomalies that a human eye might overlook. This underscores the importance of the basic principles of ECG in informing the application of advanced technologies in healthcare.
Types of ECG Leads
ECG leads serve as the channels through which the heart’s electrical signals are measured. Each lead offers a unique perspective on the heart's activity, providing complementary information.
- Standard Limb Leads (I, II, III): These leads provide a bipolar view of the heart's electrical activity.
- Augmented Leads (aVR, aVL, aVF): These leads enhance the recording from the limb leads, offering additional insight into the heart's electrical axis.
- Precordial Leads (V1-V6): These leads are placed on the chest and provide a detailed view of the heart's electrical activity from multiple angles.
Each type of lead plays a role in diagnosing specific heart conditions. The distinct views enable machine learning models to accurately identify patterns and anomalies by analyzing a more comprehensive dataset gathered from multiple leads.
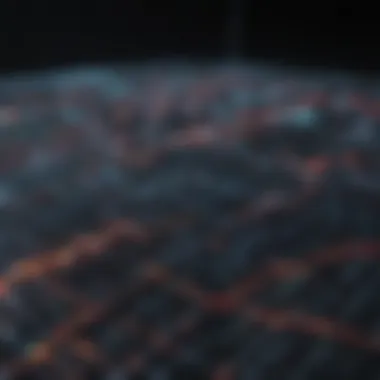
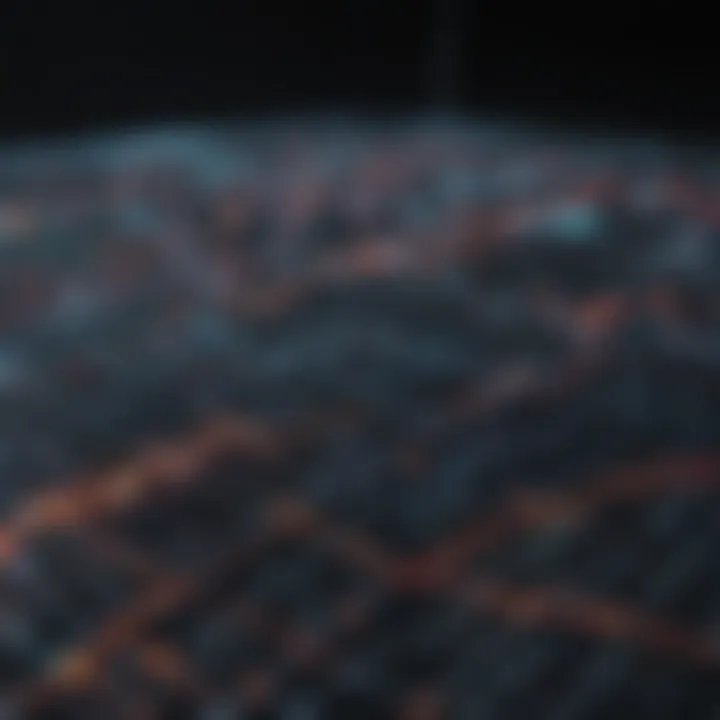
Norms and Anomalies in ECG Patterns
A core element in ECG analysis is distinguishing between normal patterns and anomalies. Normal ECG readings present a set of predictable waveforms and intervals, while deviations from these norms can indicate underlying health concerns.
Some common anomalies include:
- Arrhythmias: These reflect abnormal rhythms that can result from various causes.
- Myocardial Ischemia: It suggests insufficient blood flow to the heart muscle, often characterized by ST-segment changes.
- Heart Blocks: These are classified based on the severity of the conduction delay and can significantly impact treatment options.
Machine learning excels in this area by rapidly analyzing vast datasets. It can identify subtle deviations in ECG patterns that traditional methods might miss, facilitating earlier and more accurate diagnoses.
Machine learning algorithms significantly improve diagnostic accuracy by analyzing ECG data and identifying anomalies that may not be visible to the human eye.
Understanding these essentials of ECG allows professionals to appreciate the valuable role of machine learning in enhancing cardiac care. By fusing traditional ECG knowledge with state-of-the-art machine learning techniques, healthcare can achieve a new level of diagnostic precision and patient outcomes.
Preface to Machine Learning
Understanding machine learning is paramount in analyzing electrocardiogram (ECG) data. The intersection of these two fields presents unique opportunities to enhance cardiac diagnostics. Machine learning, as a subset of artificial intelligence, equips systems to learn from data patterns. It does this without explicit programming for every decision, allowing for complex analyses of ECG signals.
The importance of machine learning lies in its potential to interpret vast amounts of data quickly and accurately. This capability is essential in cardiology, where timely analysis can significantly influence patient outcomes. As healthcare faces increasing data complexity, machine learning provides tools to sift through noise, identifying critical patterns in heart activity.
Moreover, the integration of machine learning into ECG analysis can lead to improved trends in predictions and diagnostics. It can help identify anomalies that may not be readily visible to clinicians.
In traditional methods, it often requires deep expertise and time to interpret ECG results. However, with machine learning, the process can be expedited. The technology is evolving to a point where it not only supports clinicians but can also perform preliminary analyses independently. This duality enhances the workflow in clinical settings, allowing for more time to address patient care issues.
Ultimately, understanding the fundamentals of machine learning and its algorithms is necessary to appreciate its full role in ECG analysis. The next sections will delve deeper into these fundamentals, revealing how they can reshap the future of cardiac care.
Fundamentals of Machine Learning
Machine learning relies on algorithms that enable the extraction of information from data. To understand how this works in ECG analysis, it is essential to explain a few key concepts. Firstly, we have supervised and unsupervised learning. In supervised learning, algorithms are trained on labeled datasets containing input-output pairs. Unsupervised learning, on the other hand, deals with unlabeled data, seeking patterns or groupings.
Another crucial concept is feature extraction. In the context of ECG data, features can include heart rate variants, intervals between detections, or even wave shapes. Extracting these features allows machine learning algorithms to recognize patterns associated with normal and abnormal heart signaling.
Additionally, a process known as model training is critical. This involves feeding data into a chosen algorithm, which learns to make predictions based on identified features. The quality and accuracy of the model depend heavily on the quality of the data and feature selection.
Types of Machine Learning Algorithms
Many machine learning algorithms prove beneficial in analyzing ECG data. Each has distinct advantages depending on the application.
- Decision Trees: These algorithms split data into branches, representing decisions. They are easy to interpret, making them suitable for preliminary analyses.
- Support Vector Machines (SVM): SVM can classify different types of heart rhythms by finding a hyperplane that best separates data points.
- Neural Networks: Particularly deep learning neural networks, which mimic brain functions, are effective for complex pattern recognition within ECG signals.
- k-Nearest Neighbors (k-NN): This algorithm classifies data points based on the proximity of their k-nearest neighbors. It is simple yet efficient for small datasets.
- Random Forests: This ensemble method combines several decision trees, improving robustness and accuracy.
Machine learning sports a plethora of algorithms, each tailored for various tasks within ECG analysis. Understanding these algorithms will not only inform their application in the medical field but also enhance the overall reliability of diagnostics.
Integration of Machine Learning and ECG Analysis
The integration of machine learning with electrocardiogram (ECG) analysis is a pivotal subject in contemporary medicine. This union enhances the ability of healthcare professionals to interpret complex data derived from ECG readings, fostering improved clinical outcomes. The advent of machine learning algorithms enables the analysis of massive volumes of data with high accuracy and efficiency. It allows practitioners to recognize patterns and anomalies that might remain undetected in conventional evaluations. As the healthcare sector moves towards data-driven decision-making, understanding this integration becomes increasingly crucial.
The significance of machine learning in ECG extends beyond mere analysis. It is about evolving practice standards, enhancing the accuracy of diagnoses, and providing more tailored patient care. Traditional methods often struggle with subjectivity and variability among practitioners. However, machine learning introduces a level of objectivity and rigor, improving the consistency and reliability of ECG interpretations.
Role of Data in Machine Learning
Data serves as the foundational element of machine learning. In the context of ECG analysis, data includes recordings from various patients, annotated patterns, and outcomes. The effectiveness of machine learning models hinges on the quantity and quality of this data. Sufficient data allows algorithms to learn and adapt, ultimately leading to better diagnostic predictions.
In ECG analysis, diverse data types can enhance model performance. Examples include:
- Historical ECG recordings: Past data provides context for current assessments.
- Clinical patient data: Information on demographics and health conditions can inform algorithmic predictions.
- Electrophysiological markers: Detailed signals from ECGs can be used to train the models effectively.
Machine learning algorithms utilize this data to identify correlations and trends. This improves their ability to detect abnormal heart rhythms, ischemic events, and other cardiovascular conditions.
Training Machine Learning Models with ECG Data
Training machine learning models is an essential process that requires methodical attention. The training phase involves feeding a model with previously collected ECG data and teaching the model to recognize specific patterns associated with various heart conditions. This process usually entails supervised learning, where models are trained on labeled datasets.
The training of these algorithms can be broken down into several critical steps:
- Data selection: Choosing a diverse dataset that represents many conditions and variables is vital.
- Preprocessing: Cleaning the data, such as removing noise and ensuring uniformity, helps enhance model performance.
- Feature extraction: This step involves identifying the most relevant characteristics of ECG signals, which are crucial for making accurate predictions.
- Model evaluation: Using separate validation datasets to assess the model's diagnostic accuracy and prevent overfitting.
- Continuous Learning: Allowing the model to learn from new data as it becomes available keeps the model fresh and relevant.
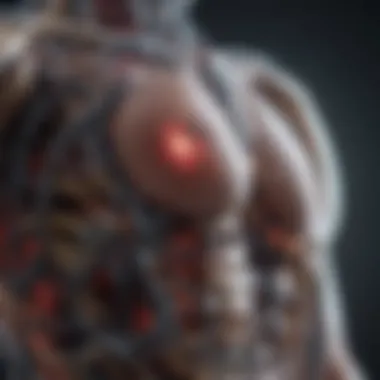
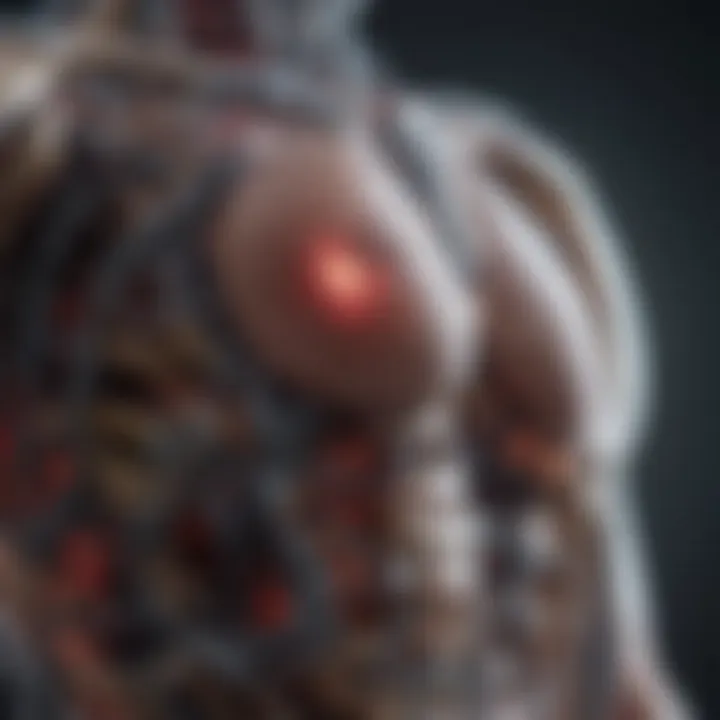
An effective training process enables the model to generalize its learning to unseen ECG traces, providing a robust tool for clinicians in real-time diagnostic situations.
Benefits of Machine Learning in ECG Analysis
In recent years, the amalgamation of machine learning with electrocardiogram (ECG) technology has gained prominence. By harnessing complex algorithms, machine learning enhances the analysis of ECG data significantly. This section underscores the diverse benefits that machine learning brings to ECG analysis, focusing on improved diagnostic accuracy, efficiency in analysis, and the potential for predictive analytics in cardiology.
Improved Diagnostic Accuracy
One notable advantage of utilizing machine learning in ECG analysis is its potential to improve diagnostic accuracy. Traditional methods often rely on human interpretation of ECG waveforms, which can lead to variability and errors. In contrast, machine learning algorithms can analyze large datasets consistently and quickly.
Research indicates that these algorithms can identify subtle patterns that human experts might overlook. For example, convolutional neural networks processed with thousands of well-labeled ECG samples improve their ability to spot arrhythmias and other cardiac conditions. This leads to more reliable outcomes and enhances the overall quality of care.
Additionally, machine learning models can adapt and improve over time. As new data is fed into the system, the models learn to refine their predictions, continually boosting diagnostic precision.
Efficiency in Analysis
Another significant benefit is the efficiency that machine learning brings to ECG analysis. Manual examination of ECG recordings can be time-consuming, distracting healthcare professionals from other critical tasks. Machine learning automates this process.
Algorithms can swiftly analyze large volumes of ECG data, helping in real-time assessment. For instance, in emergency rooms where every second counts, algorithms can immediately identify life-threatening cardiac events, allowing quick intervention.
Furthermore, automation minimizes the possibility of human error during analysis, ultimately optimizing clinical workflows. By reducing the time needed for analysis, healthcare providers can allocate resources more effectively, focusing on patient care rather than data processing.
Predictive Analytics in Cardiology
Machine learning unlocks the capability for predictive analytics within cardiology. Instead of merely identifying existing conditions, these algorithms can forecast potential cardiovascular issues based on patient data. They can analyze trends and correlations found within the ECG readings alongside other factors, such as patient history and demographics.
This predictive capacity enables proactive care strategies, allowing clinicians to implement preventive measures before serious conditions arise. For instance, machine learning models can identify patients at high risk for heart failure. This capability significantly enhances patient outcomes and reduces healthcare costs in the long run.
"Machine learning is not just the future, but the present of cardiovascular diagnostics, providing timely insights that save lives."
In summary, the integration of machine learning into ECG analysis provides clear benefits like improved diagnostic accuracy, greater efficiency, and enhanced predictive capabilities. As technology continues to evolve, the role of machine learning in cardiology will likely expand, paving the way for more refined and proactive healthcare solutions.
Challenges in Implementing Machine Learning for ECG
Implementing machine learning within the realm of electrocardiogram (ECG) analysis is a necessity for transforming cardiovascular healthcare. However, this integration brings forth several challenges. As the healthcare industry advances towards precision medicine, understanding the obstacles of machine learning application is essential. Acknowledging these challenges aids in enhancing the efficiency and accuracy of ECG analysis, which is crucial when it comes to patient care.
Data Quality and Quantity
The backbone of any machine learning model is its data, particularly in the context of ECG analysis. High-quality, well-annotated, and diverse datasets are fundamental for training robust models. Poor data quality can lead to biased outcomes, thereby affecting diagnostic accuracy. Insufficient sample sizes may result in overfitting, where the model performs well on training data but fails to generalize to new inputs.
To realize the full potential of machine learning in ECG, there needs to be a dedicated effort to curate and standardize ECG data from various sources. Collecting large datasets that are both balanced and representative ensures that models can learn effectively from a wide range of heart conditions. This can also involve synthesize data to enhance quantity without compromising quality.
Interpretability of Machine Learning Models
A significant hurdle in using machine learning for ECG analysis is the interpretability of the models. Many advanced algorithms, such as deep learning, act as black boxes, providing complex outputs that are not easily understood. Clinicians need to trust and comprehend the reasoning behind a model’s diagnosis or prediction.
Without interpretability, the adoption of machine learning solutions can be hesitant among practitioners. It is important for model outputs to be explained in simpler terms, allowing healthcare providers to integrate results into their clinical decision-making confidently. Developing tools that can elucidate how inputs affect outputs will significantly improve acceptance in clinical settings.
Integration into Clinical Practice
Incorporating machine learning into existing clinical workflows is challenging. The typical healthcare setting is already complex, with various stakeholders, protocols, and technologies at play. Introducing new algorithms requires careful consideration of how they fit into current practices.
Training staff on new systems and ensuring that these technologies complement traditional methods can pose logistical issues. Moreover, regulatory frameworks must also be adapted to accommodate these changes. The need for real-time processing of ECG data, alongside the accuracy and reliability of machine learning models, leads to additional demands on infrastructure and resources.
Integrating machine learning frameworks requires a multidisciplinary approach, considering technical, operational, and human factors to achieve effective implementation.
Case Studies of Machine Learning in ECG
The integration of machine learning into electrocardiogram (ECG) analysis represents a significant evolution in cardiovascular diagnostics. Case studies provide concrete examples of how these advanced algorithms contribute to clinical practice. By examining real-world applications, we can better understand the potential benefits and also the challenges in utilizing machine learning for ECG interpretation.
Successful Applications
One prominent example of machine learning in ECG is the development of algorithms designed to detect atrial fibrillation (AF). Atrial fibrillation is a common arrhythmia that increases the risk of stroke and heart failure. Research shows that machine learning models, when trained on extensive datasets of ECG signals, can identify AF with high sensitivity and specificity. For instance, a study published in Nature Biomedical Engineering highlighted a convolutional neural network that outperformed traditional models in AF detection, achieving over 95% accuracy.
- Improved Outcomes: Early and accurate detection of AF using machine learning algorithms can lead to timely intervention. This is crucial in preventing complications associated with the condition.
- Scalability: As these models can analyze large volumes of ECG data quickly, they are well-suited for use in telehealth and remote monitoring scenarios.
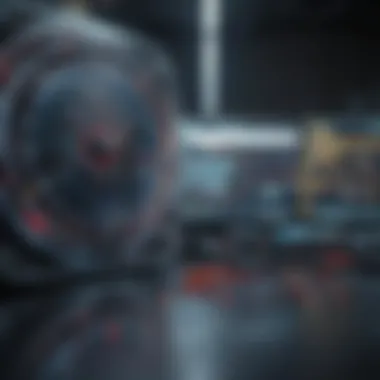
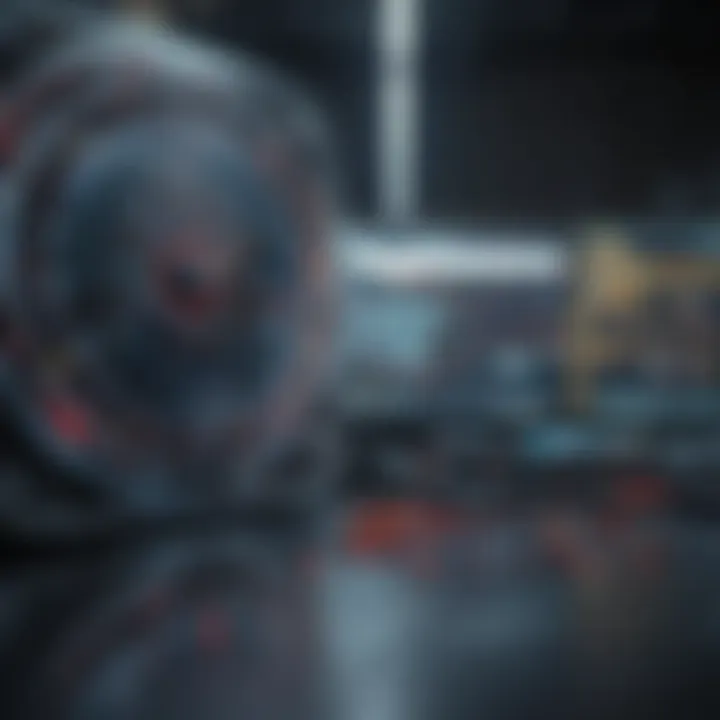
Another noteworthy application is the use of machine learning for the identification of myocardial infarctions or heart attacks. Models that analyze ECG traces have demonstrated the ability to recognize patterns indicative of ischemia far more efficiently than manual interpretation. A study involving the integration of algorithms by the Massachusetts Institute of Technology resulted in a classification system that could distinguish between normal and abnormal heart rhythms with reduced false positive rates.
Comparative Studies
Comparative studies highlight how machine learning models stack against traditional methods in ECG analysis. For example, some research has focused on comparing neural networks to the standard algorithmic approaches used in eCG interpretation. These studies often reveal that machine learning not only matches but frequently surpasses the performance of classic diagnostic tools.
- Performance Metrics: Specific performance metrics, such as area under the curve (AUC) and accuracy rates, have shown that machine learning models can provide enhanced diagnostic insights. In one case, a deep learning model for diagnosing left ventricular hypertrophy demonstrated an AUC of 0.93 compared to the 0.79 AUC achieved by standard diagnostic techniques.
- Limitations: Despite their impressive capabilities, machine learning models still face limitations, such as reliance on the quality of training data and the potential for overfitting. These limitations raise critical questions about the robustness and generalizability of the findings.
Research indicates that machine learning is paving the way for more precise ECG interpretation. As these technologies continue to evolve and improve, they promise significant advancements in the field of cardiology. They not only enhance diagnostic accuracy but also improve patient outcomes through earlier detection and treatment of cardiovascular issues.
Future Prospects of ECG and Machine Learning
Exploring the future prospects of machine learning in ECG analysis is critical. This evolving synergy promises several significant improvements in the cardiology field. The integration of advanced technologies into ECG interpretation can streamline workflows, enhance diagnostic accuracy, and improve patient outcomes. In this section, we will delve into emerging technologies in ECG and the specific predictions for the future of cardiology.
Emerging Technologies in ECG
Technological advancements are bringing new opportunities for ECG analysis. One notable emerging technology is wearables, like smartwatches and fitness trackers. These devices collect real-time ECG data, allowing continuous monitoring outside of traditional clinical settings. They can detect anomalies and send alerts to healthcare professionals immediately.
Moreover, improved imaging techniques, such as high-resolution ultrasound and portable imaging devices, complement machine learning models. Combining data from various sources enhances the model's effectiveness and robustness, making it vital for precise diagnostics. Other technologies, like telemedicine platforms, facilitate remote consultations. This integration allows professionals to evaluate ECG data with advanced machine learning algorithms, leading to timely interventions.
Key Technologies to Observe:
- Continuous ECG monitoring via wearables
- High-resolution imaging techniques
- Telemedicine platforms for remote diagnostics
Predictions for Cardiology
The future of cardiology will likely be shaped by the greater application of machine learning in ECG analysis. With advancements in algorithms and increasing datasets, predictive analytics will become more accurate. It is expected that, in the next few years, machine learning models will be able to predict cardiac events before they happen. This paradigm shift could significantly reduce morbidity associated with cardiovascular diseases.
Academics speculate that training and validation datasets will expand, incorporating diverse demographics. This might lead to models that are more representative and less biased, ensuring fair outcomes across various population groups. As machine learning evolves, it may also facilitate personalized medicine in cardiology. Tailoring interventions based on individual risk profiles could lead to more effective treatment plans.
One potential development might be the integration of artificial intelligence in electrophysiology. AI-driven tools could analyze ECG data for arrhythmias with greater precision. This will enable cardiologists to focus on nuanced aspects of care rather than routine diagnostic tasks.
Ethical Considerations in Machine Learning and ECG
The intersection of machine learning and electrocardiogram (ECG) analysis brings forth numerous ethical considerations. These challenges are crucial to address, as they influence the effectiveness and acceptance of these technologies in clinical practices. As machine learning increasingly becomes involved in healthcare, including ECG diagnostics, understanding ethical ramifications can protect patient rights and enhance trust in the emerging systems.
Bias in Data and Algorithms
Bias in data and algorithms poses a significant threat in the realm of machine learning. This issue arises when the training data used to develop algorithms is not representative of the broader population. If machine learning models are trained on biased datasets, they may produce incorrect or unfair results. In the context of ECG analysis, this bias can lead to disparities in diagnosing various groups.
For example, if an algorithm largely trained on data from one demographic fails to account for the physiological variations in other populations, it may lead to misdiagnosis or inadequate treatment recommendations for those underrepresented. In turn, this exacerbates existing healthcare inequalities.
To mitigate bias, developers need to prioritize diversity in their datasets. Moreover, transparency in algorithm development should be promoted. Regular audits of machine learning systems can help identify and rectify biased outcomes. Understanding these ethical dimensions is vital because it ensures fair treatment of all patients and maintains the integrity of the healthcare system.
Patient Privacy and Data Security
Patient privacy and data security are paramount when integrating machine learning with ECG analysis. With the increasing collection of sensitive health information, ensuring the confidentiality and protection of patient data is critical. Machine learning models often require vast amounts of data to function optimally. This necessity raises concerns about how this data is collected, stored, and processed.
Healthcare institutions must adhere to regulations like the Health Insurance Portability and Accountability Act (HIPAA) to protect patient information. Additionally, when using third-party algorithms, the responsibility for data security can become complex. This complexity necessitates rigorous vetting of external systems to ensure they comply with security standards.
Furthermore, organizations should invest in encryption methods and secure data storage solutions. They must also educate staff on best practices for handling sensitive data. The implications of failing to secure patient data are severe, including breaches that could compromise patient trust.
"Implementing effective data security measures enhances the overall trustworthiness of machine learning in ECG analysis."
In summary, addressing ethical considerations in machine learning and ECG analysis is essential. By confronting biases in data and ensuring patient privacy, practitioners can create a more equitable and trustworthy health system. Attention to these issues will help secure patient outcomes and support the responsible advancement of technology in cardiology.
Culmination
The conclusion of this article encapsulates the transformative impact of machine learning on electrocardiogram analysis. It marks a pivotal moment in cardiology, highlighting how these advanced technologies redefine diagnostic protocols. The integration of machine learning offers numerous benefits, including enhanced accuracy in detecting abnormalities, increased efficiency in data analysis, and the potential for predictive analytics that can forecast cardiovascular events.
Summary of Key Insights
Several key insights emerge from the exploration of machine learning's role in ECG analysis:
- Diagnostic Precision: Machine learning models have displayed remarkable capabilities in recognizing patterns that may be missed by traditional approaches. This precision is crucial for timely and accurate patient assessments.
- Data-Driven Insights: Leveraging large datasets allows for better training of machine learning algorithms. This leads to refined models that improve over time, enhancing their effectiveness.
- Streamlining Workflows: Automating ECG analysis not only reduces the workload on healthcare professionals but also improves patient care timelines, facilitating quicker decision-making during clinical assessments.
- Predictive Capabilities: Through continuous learning, machine learning models can identify risk factors and predict future cardiac events, which is invaluable for preventative care.
Final Thoughts on Future Developments
Looking ahead, the integration of machine learning in ECG analysis suggests several promising developments:
- Advancements in Algorithms: As research progresses, further refinements to machine learning algorithms tailored for ECG data will emerge, potentially enhancing diagnostic capabilities even more.
- Broader Applications: The future may see machine learning extending its reach beyond ECGs, applying similar methodologies in other areas of cardiology and related fields.
- Regulatory Frameworks: As machine learning becomes more entrenched in clinical practice, the establishment of rigorous regulatory standards will be essential to ensure patient safety and data integrity.
- Increased Accessibility: As technology advances, machine learning tools may become more accessible to healthcare facilities worldwide, improving cardiology diagnostics across diverse regions.