Exploring Thermodynamics Simulation Techniques and Uses
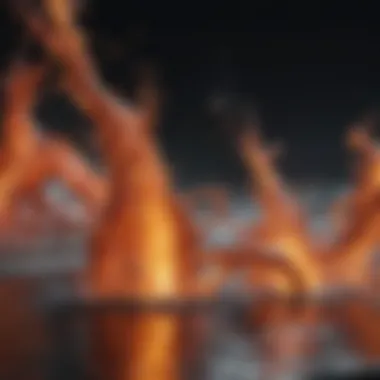
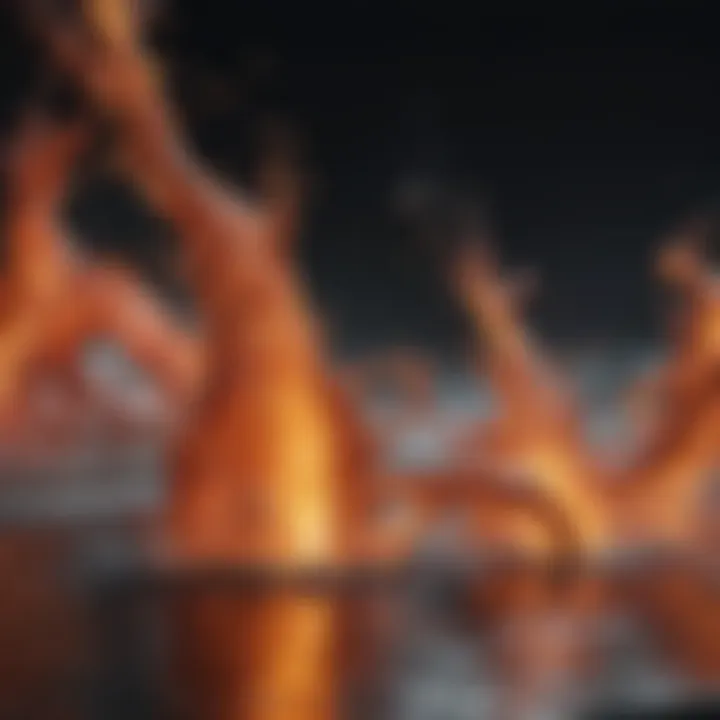
Intro
Thermodynamics simulation stands as a pivotal branch of computational science, encompassing the analysis of energy and matter transfer in various systems. As a discipline, it bridges theoretical principles with practical applications across numerous fields, including engineering, chemistry, and material science. This article aims to dive into the frameworks, methodologies, and significant implications of thermodynamics simulations, emphasizing their role in research and industry advancement.
Understanding thermodynamics is critical for professionals who seek to optimize systems for energy efficiency, safety, and economic viability. With the rise of computational power, simulations have become an essential tool for researchers and practitioners who require precise predictions of system behavior under varying conditions.
In this exploration, various aspects of thermodynamics simulation will be dissected. The discussion will include foundational principles, notable advancements in computational methods, and diverse applications ranging from industrial processes to climate modeling. By studying case examples, the narrative seeks to provide a detailed insight into how these simulations enhance scientific understanding and empower innovative solutions in real-world contexts.
The discussion commences with a thorough overview of research in this area, where we summarize key findings and highlight the importance of ongoing research.
Prelude to Thermodynamics Simulation
Understanding thermodynamics simulation is vital in numerous scientific disciplines. The importance lies in its ability to analyze complex thermodynamic systems, providing insights that are not easily obtainable through experimental methods alone. With the advent of advanced computational tools, thermodynamics simulation has become an indispensable technique. It not only enhances our comprehension of fundamental principles but also refines practical applications in industries like energy, materials science, and environmental studies.
Thermodynamics itself is the study of energy transfer and transformation in physical systems. Its significance extends far beyond theory; it has real-world applications that impact technology and scientific research. Simulation serves as a bridge between theoretical frameworks and practical execution. This article will explore the foundations of thermodynamics and the essential role simulation plays in revealing the intricacies of related systems.
The main benefits of thermodynamics simulation include:
- Safety: This approach allows for the exploration of extreme conditions without the risks associated with physical experiments.
- Cost-Efficiency: Simulations can often be performed more economically than extensive laboratory work.
- Time-Saving: They provide rapid insights, enabling quicker iterations in design and testing phases.
However, considerations must be made when employing simulations. The accuracy of results is dependent on chosen models, boundary conditions, and computational resources. It is crucial for researchers to understand these factors to ensure reliable outcomes.
Ultimately, the interplay of thermodynamics and simulation unlocks possibilities for innovation in research and industry. The subsequent sections will delve deeper into defining thermodynamics, exploring what simulation means in this context, and highlighting its applications in various fields.
Defining Thermodynamics and Its Importance
Thermodynamics is the branch of physics that deals with the relationships between heat and other forms of energy. It involves the study of laws that govern energy conversion and the behavior of matter under energy changes. The four fundamental laws of thermodynamics form the backbone of this field and provide a framework to analyze energy processes in both isolated and open systems.
The laws are:
- Zeroth Law: Defines thermal equilibrium.
- First Law: Relates to energy conservation.
- Second Law: Addresses entropy and irreversible processes.
- Third Law: Pertains to the behavior of systems at absolute zero temperature.
Understanding these principles is essential because they guide the design and operation of engines, refrigerators, and various thermal systems utilized across industries. The importance of thermodynamics extends to fields such as chemistry, biology, and environmental science. It lays the groundwork for analyzing phenomena such as phase transitions, chemical reactions, and biological processes.
What is Simulation in Scientific Research?
Simulation in scientific research refers to the use of computational models to replicate and analyze the behavior of complex systems. It allows scientists and engineers to explore scenarios and predict outcomes without physically conducting experiments. This method is useful in various research domains, including thermodynamics, where it enables the study of systems that are challenging to observe directly.
Simulations can be broadly categorized into two types: deterministic and probabilistic. Deterministic simulations rely on precise mathematical models and produce consistent results under given conditions. Probabilistic simulations, on the other hand, incorporate uncertainty and randomness, often reflecting the variability found in real-world systems.
"Simulation is not just a tool; it is an essential part of modern scientific inquiry."
In the context of thermodynamics, simulations can help elucidate complex thermodynamic properties and predict behavior under varying conditions. They assist in research and development by allowing repeated testing of hypotheses, optimizing designs, and running sensitivity analyses. As technology evolves, the capabilities of simulations continue to expand, further enhancing their relevance in scientific exploration.
Fundamental Principles of Thermodynamics
Understanding the Fundamental Principles of Thermodynamics is crucial in the context of thermodynamics simulations. These principles form the backbone that guides many scientific investigations. They enable researchers to predict the behavior of physical systems under varying conditions. In simulations, these principles guide model development and interpretation of results. Knowledge of them reduces errors and enhances the predictive power of simulations.
Laws of Thermodynamics
The laws of thermodynamics consist of four main principles that govern energy transfer and transformation.
- Zeroth Law: This law establishes thermal equilibrium. If two systems are in thermal equilibrium with a third system, they are also in equilibrium with each other. This law underpins the concept of temperature, which is fundamental for simulations involving thermal dynamics.
- First Law: This law, also known as the law of energy conservation, asserts that energy in a closed system remains constant. Energy can neither be created nor destroyed; it can only change forms. In simulations, this law is essential when modeling energy interactions between systems.
- Second Law: This law addresses the concept of entropy, stating that total entropy can never decrease over time. It implies that processes occur in a direction of increasing disorder. Understanding this principle is vital for creating realistic models in simulations that depict spontaneous processes.
- Third Law: This law states that as the temperature of a system approaches absolute zero, its entropy approaches a constant minimum. This has implications for understanding systems that operate near cryogenic temperatures and is crucial in simulations that involve such conditions.
These laws provide a framework for analyzing thermodynamic systems, allowing researchers to validate their simulations against known physical laws.
Thermodynamic Properties of Substances
Thermodynamic properties are unique characteristics of substances that define their behavior under different conditions. Key properties include:
- Temperature: This is a measure of the average kinetic energy of the particles in a substance. In simulations, accurate temperature representation is key to predicting thermodynamic phenomena like phase changes.
- Pressure: This denotes the force exerted by the substance per unit area. Knowing how pressure influences phase transitions can be essential in thermodynamic modeling and simulations.
- Volume: The space occupied by a substance, which is particularly relevant in the context of gases. Understanding the relationship between volume and pressure is essential, especially in ideal gas law applications.
- Enthalpy: A measure of total heat content, crucial in processes involving heat transfer. Enthalpy changes can indicate whether a reaction is exothermic or endothermic, information critical for simulations of chemical reactions.
- Entropy: As mentioned earlier, this property quantifies disorder within a system. Recognizing entropy changes helps in determining the spontaneity of reactions in simulations.
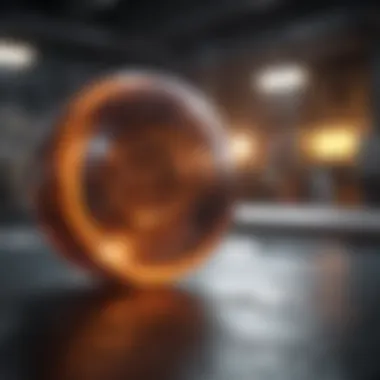
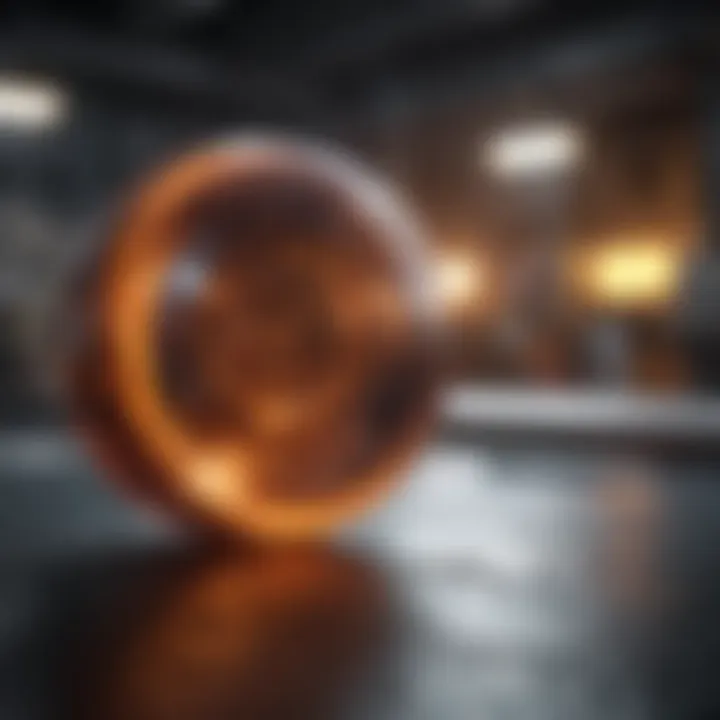
Studying these properties enables researchers to create accurate models that can predict outcomes reliably. The application of these principles in thermodynamic simulations serves as an important bridge between theory and practical applications.
Types of Thermodynamic Simulations
Thermodynamic simulations play a vital role in understanding complex systems across various scientific fields. In this section, we will delve into the different types of simulations employed in thermodynamics. Each type offers unique insights and methods for analyzing the behavior of substances under varying conditions. This understanding is key for students, researchers, and professionals who rely on accurate predictions in their work.
Equilibrium versus Non-Equilibrium Simulations
Equilibrium simulations focus on systems that have reached a state where macroscopic properties do not change over time. This stability allows for the analysis of important properties like pressure, temperature, and volume.
Key features of equilibrium simulations include:
- Thermodynamic Consistency: Results from these simulations are often consistent with established laws of thermodynamics.
- Statistical Mechanics Foundation: They are based on fundamental principles of statistical mechanics that pertain to large numbers of particles.
On the other hand, non-equilibrium simulations examine systems that are in flux. These systems do not maintain constant properties, and their behavior can change rapidly due to external influences.
Some fascinating aspects of non-equilibrium simulations are:
- Dynamic Processes: They are crucial for understanding dynamic processes like heat transfer, chemical reactions, and shock wave propagation.
- Complex Modeling: Non-equilibrium simulations often require advanced modeling techniques, making them resource-intensive but invaluable for accurate predictions.
Molecular Dynamics Simulations
Molecular dynamics simulations offer an atomic-level view of systems. They track the movements of atoms and molecules over time based on their interactions. This is achieved through numerical integration of Newton's equations of motion.
- Real-time Interaction: It allows scientists to observe how molecular interactions affect material properties in real-time.
- Versatile Applications: Commonly used in fields like biochemistry, material sciences, and nanotechnology, these simulations provide deep insights into molecular behavior.
Moreover, molecular dynamics can help predict outcomes in commercial applications. It can save time and resources by enabling research teams to identify promising materials before experimentation.
Monte Carlo Simulations
Monte Carlo simulations use random sampling to calculate results. This method is particularly useful for systems that are too complex for analytical solutions.
Benefits of Monte Carlo simulations include:
- Statistical Error Reduction: They help mitigate errors caused by small sample sizes, improving the reliability of predictions.
- Flexibility: This method is adaptable to various kinds of thermodynamic systems, making it broadly applicable in research and industry.
In practice, Monte Carlo simulations can simulate various processes, like phase transitions, and provide valuable data for researchers looking to enhance materials or understand reactions.
"Different types of simulations open unique windows into the behaviors of materials and systems, allowing for enhanced understanding and innovation across fields."
By emphasizing the differences between equilibrium and non-equilibrium, as well as the capabilities of molecular dynamics and Monte Carlo methods, researchers can select the most appropriate tools for their specific inquiries. Understanding these types deepens the comprehension of thermodynamic phenomena and guides practitioners in both academic and industrial settings.
Methods and Techniques in Thermodynamic Simulations
The methods and techniques utilized in thermodynamic simulations are crucial for understanding complex physical phenomena. They underpin the processes which model real-world behaviors of various systems. Knowing the appropriate method increases the accuracy and efficiency of simulations. Given the wide variety of scenarios—ranging from industrial applications to basic research—each technique has unique strengths and weaknesses. This section explores several of the predominant techniques employed today, as well as their specific benefits and considerations.
Computational Fluid Dynamics (CFD) Methods
Computational Fluid Dynamics (CFD) stands out as a fundamental tool in thermodynamics simulation. CFD enables the analysis of fluid flow and heat transfer using numerical methods and algorithms. The advantage of CFD is its ability to solve complex equations governing fluid motion, including the Navier-Stokes equations. This allows for a detailed insight into how fluids behave under different conditions.
CFD is particularly valuable in industrial settings, where understanding fluid dynamics is essential for optimizing processes such as combustion, mixing, and heat exchangers. However, using CFD requires an understanding of both the underlying physics and the numerical methods involved. Adequate meshing and boundary conditions play critical roles in the accuracy of results.
Finite Element Analysis (FEA)
Finite Element Analysis (FEA) is another significant method used in thermodynamic simulations. FEA involves breaking down a complex structure into smaller, simpler parts called finite elements. This method allows for analyzing stress, strain, and thermal conditions within materials under various loads or boundary conditions.
In practical terms, FEA is applied in scenarios where materials experience non-uniform stresses or varying temperature distributions. This technique is widely used in engineering fields, especially in structural and mechanical design. The precision obtained through FEA is often higher than other methods; however, it can entail higher computational costs and require careful attention to element size and quality.
Stochastic Simulations
Stochastic simulations consider randomness and uncertainty in their models. This approach is advantageous in scenarios where variability in system parameters is significant. For example, in predicting how temperature or pressure might fluctuate, stochastic simulations can provide a range of possible outcomes instead of a single deterministic solution.
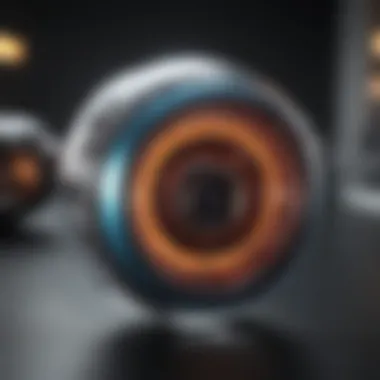
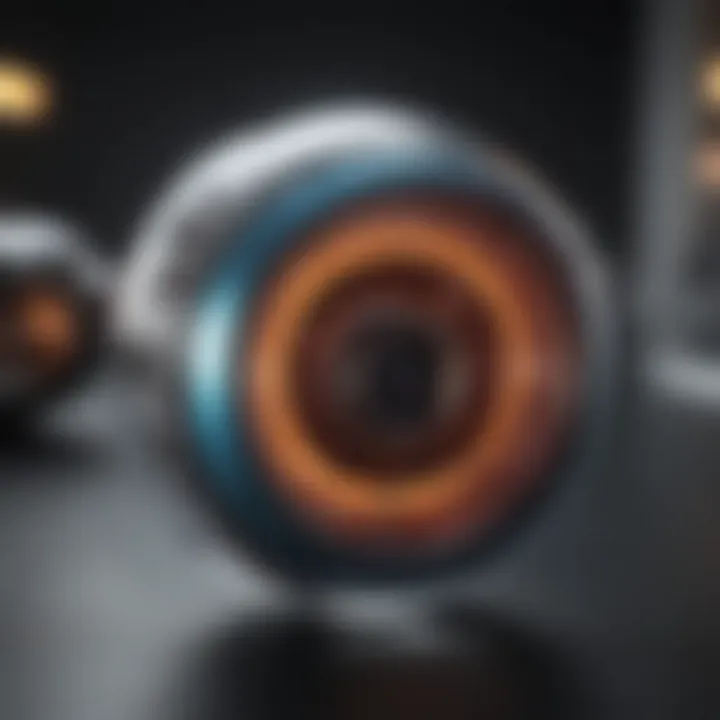
These simulations often use statistical methods to analyze the effects of random variables. This is particularly useful in fields like environmental science, where variability can deeply affect results. However, the key challenge of stochastic methods lies in their complexity. They may require advanced programming skills and greater computational resources to analyze the generated data effectively.
"Understanding and correctly applying the right method can dramatically enhance the validity of simulations in thermodynamics."
In summary, the choice of method in thermodynamic simulations is vital. Each technique offers distinct advantages tailored to specific applications, and careful consideration is necessary to ensure the results align with the practical realities of the systems being modeled. Continual advancements in computational techniques further enrich the field, allowing for more accurate and applicable simulations.
Software Tools for Thermodynamics Simulation
The realm of thermodynamics simulation is increasingly complex. Consequently, effective software tools are essential for both researchers and industry professionals. These tools facilitate accurate modeling and analysis of thermodynamic systems, making the understanding of physical phenomena more accessible. The selection of appropriate software can greatly impact the efficiency and accuracy of simulations. This section will dissect two primary categories of software tools used in thermodynamics simulation: commercial software solutions and open-source platforms. Each category showcases unique advantages and potential limitations.
Commercial Software Solutions
Commercial software options dominate the thermodynamics simulation landscape. They offer developers a comprehensive framework designed for specific applications. Popular software includes ANSYS Fluent, COMSOL Multiphysics, and Aspen Plus. These tools excel in their usability and integration capabilities.
Key benefits of commercial software solutions include:
- Robust User Support: Many companies provide extensive documentation and customer support.
- Advanced Features: Additional functionalities are often provided, such as multi-phase flow modeling and chemical reaction simulations.
- Regular Updates: These software solutions receive updates that improve accuracy and expand capabilities, keeping pace with advancements in the field.
However, there are inherent drawbacks. Commercial software typically comes with hefty licensing fees. This can limit access for smaller institutions or individual researchers. Additionally, users may face proprietary limitations, hindering customization for specific research needs.
This trade-off between cost and features often leads to a dilemma for many in the field. Evaluation of these tools should consider the balance between financial commitment and the need for robust capabilities.
Open-Source Platforms
Open-source platforms offer an alternative to commercial software. They are often free to use, providing researchers with valuable tools without significant financial barriers. Examples of popular open-source tools include OpenFOAM, R, and Python’s CoolProp library. These platforms are appealing because of their flexibility and transparency. Users can modify source code to tailor the software to their needs, which is a monumental advantage for creative problem-solving in thermodynamics.
Advantages of open-source platforms are:
- Cost-Effectiveness: No licensing fees allow widespread accessibility.
- Community Support: Many open-source projects have vibrant communities and forums for sharing knowledge and solving problems collaboratively.
- Customization: Users can adapt tools to specific project requirements, enhancing efficiency.
On the flip side, these platforms may lack the polished interfaces of commercial options, and user support may not be as structured. New users might encounter a steeper learning curve. It’s essential to recognize that while open-source tools foster innovation, they may require more initial investment in learning and customization.
"The choice between commercial and open-source software often lies in the specific needs of the user and the context of the research."
In summary, the landscape of software tools for thermodynamics simulation presents varied options. Each option brings its own set of advantages and challenges. Thoughtful consideration of these elements allows researchers and professionals to make informed decisions, maximizing the potential of their simulations.
Applications of Thermodynamics Simulation
The scope of thermodynamics simulation extends across multiple fields, offering crucial insights into both industrial and academic contexts. Its applications are pivotal for enhancing operational efficiencies, advancing theoretical knowledge, and driving innovations. By employing diverse simulation methodologies, researchers and professionals can model complex thermodynamic systems, analyze varied conditions, and predict behaviors of materials or processes under different constraints. This section delves into the multifaceted applications of thermodynamics simulations, highlighting specific areas where their impacts are most pronounced.
Industrial Applications
In various industries, thermodynamics simulation proves vital. It allows engineers to optimize processes such as heat exchangers and reactors. For instance, in chemical engineering, modeling a reaction's thermodynamic properties can guide choices regarding temperature and pressure, leading to better yield. A notable tool in this area is Aspen Plus, which helps in simulating chemical processes quite effectively.
Additionally, in oil and gas industries, simulations assist in reservoir modeling. Understanding fluid behavior at various depths assists in extraction planning, minimizing waste, and improving sustainability.
Moreover, industries such as aerospace and automotive utilize these simulations to study engine cycles and thermodynamic efficiency. By enabling the analysis of thermal management systems, companies can reduce energy consumption and increase overall efficiency.
Research in Material Sciences
Material sciences benefit significantly from thermodynamics simulations. This field involves the study of new materials and their properties, crucial for technological advancements. By simulating material interactions at the atomic level, researchers can predict behavior under various conditions. This is especially important in battery technology, where understanding thermal behaviors can lead to improvements in energy density and safety.
Simulations often employ methods like molecular dynamics and Monte Carlo. This allows scientists to analyze phase transitions, phase stability, and thermal properties, leading to materials that are both high-performing and safe.
Furthermore, the simulation facilitates the design of alloys and composites. By identifying optimal compositions and processing methods, researchers can innovate materials tailored for specific applications, from electronics to construction.
Environmental Studies and Sustainability
The influence of thermodynamics simulation on environmental studies is growing in importance. With climate change being a pressing global issue, understanding thermodynamic behaviors in natural processes helps inform solutions. For example, simulations can model atmospheric thermodynamics, aiding in weather predictions and climate modeling.
In sustainability research, these simulations guide renewable energy projects. Understanding how to optimize the efficiency of solar panels or wind turbines requires extensive modeling of energy transfers and storage systems. By simulating different configurations, researchers can determine which designs maximize energy output while minimizing material use.
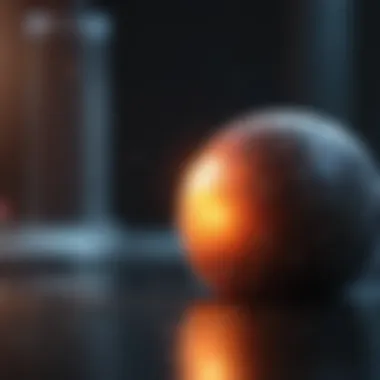
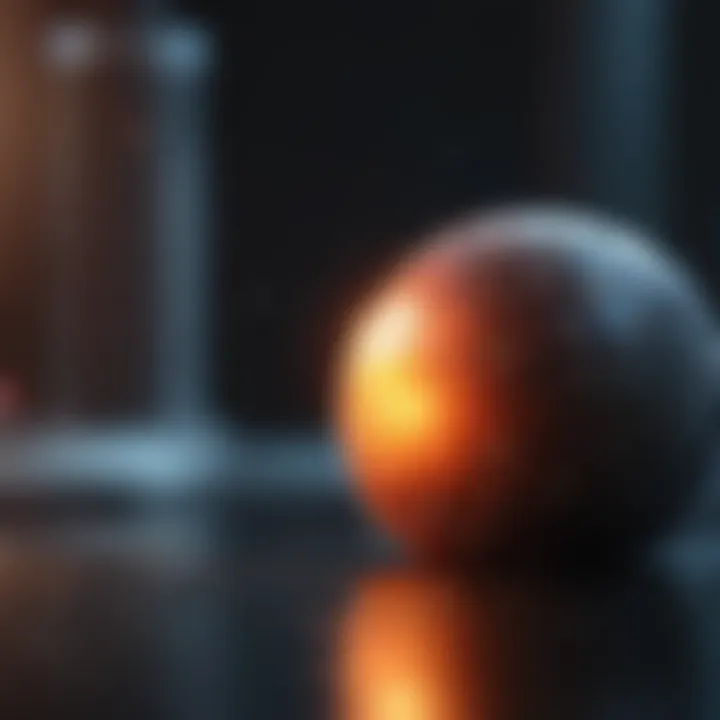
Moreover, thermodynamics simulations contribute significantly to waste management strategies. By analyzing thermodynamic processes involved in recycling, researchers can create more efficient methods to recover valuable materials, thus reducing landfill waste.
"Simulating thermodynamic systems enables researchers to derive critical insights that aid technological and environmental advancements."
The applications of thermodynamics simulation are extensive. They overlap between enhancing industrial processes, innovating in material sciences, and guiding research in environmental sustainability. As methodologies evolve, so too will the potential benefits that stem from accurate simulations across these vital fields.
Challenges in Thermodynamics Simulation
In the pursuit of accurately modeling thermodynamic phenomena, researchers and practitioners encounter numerous challenges. Addressing these challenges is crucial since inaccuracies can lead to flawed conclusions, which can, in turn, have significant repercussions in fields ranging from engineering to environmental science. This section delves into the complexities surrounding thermodynamic systems and examines the limitations of current simulation models, emphasizing their implications.
Complexity of Thermodynamic Systems
Thermodynamic systems can be intricate and multifaceted. The interaction of numerous variables - such as temperature, pressure, phase transitions, and chemical reactions - contributes to their complexity. Additionally, systems often involve non-linear behaviors that are not easily predictable.
A notable example is the behavior of fluids under varying conditions. Fluid dynamics can be highly sensitive to small changes in parameters, making it challenging to simulate accurately. In real-world applications, many systems do not exist in isolation. They interact with their environment, which adds another layer of complexity.
Key factors contributing to this complexity include:
- Multiscale phenomena: Different physical phenomena occur at different scales, from atomic interactions to bulk material properties.
- Coupled processes: Processes such as heat transfer, mass transfer, and chemical kinetics often interconnect, requiring a comprehensive understanding of each to model effectively.
- Dynamic conditions: Real-world systems frequently operate under changing conditions, making static models insufficient.
These complexities necessitate advanced techniques and rigorous methodologies in simulations. Developing accurate models that capture all relevant behaviors becomes a formidable challenge, often requiring significant computational resources and expertise.
Limitations of Current Simulation Models
Despite advancements in computational power and simulation methodologies, current models still face limitations that can restrict their effectiveness. These limitations often stem from the assumptions and approximations made during the modeling process.
Common limitations include:
- Simplifying assumptions: Many models rely on assumptions to make calculations feasible. These simplifications can overlook critical interactions or variables.
- Validation issues: Ensuring that a model accurately reflects real-world behavior is essential. However, validation can be challenging due to the difficulty of obtaining comprehensive empirical data.
- Computational expense: High-fidelity simulations require substantial computational resources, which may not be accessible for all researchers or institutions.
- Scalability problems: While simulations might work well for small systems, scaling them up to more complex scenarios can introduce discrepancies.
Current models may fail to account for all intricacies, which can lead to significant errors in predictions.
Future Directions of Thermodynamics Simulation Research
The field of thermodynamics simulation is poised for significant advancements. These developments are not only pertinent to the research community but also critical for various industrial applications. As scientists and engineers encounter increasingly complex problems, how simulations evolve will determine how effectively they can model and predict thermodynamic behavior. Two main areas stand out for their evolution—integrating artificial intelligence and machine learning, and enhancing computational power. Both of these directions promise to increase the accuracy and efficiency of simulations, which in turn can lead to better decision-making and innovation in a multitude of industries.
Integrating AI and Machine Learning
The integration of AI and machine learning into thermodynamics simulations offers promising avenues for automation and optimization. These technologies can analyze vast datasets to identify patterns and correlations that might not be evident through traditional analytical methods. Machine learning algorithms can also optimize parameters within simulations, reducing the time and resources needed for modeling complex systems.
- Predictive modeling can significantly improve when AI algorithms learn from historical data, allowing for real-time adjustments and predictions based on changing conditions.
- Enhanced data analysis enables scientists to process and interpret simulation outputs more efficiently. By employing AI techniques, researchers can generate insights that inform experimental design and strategy.
- Machine learning can be applied to reduce model errors, increasing the reliability of outcomes derived from thermodynamic simulations.
These factors lead to a more dynamic approach to simulation, where AI continuously learns and adapts, improving its predictions and analyses over time.
Enhancements in Computational Power
The increase in computational power has been one of the most transformative aspects of scientific research in recent years. With advances in hardware, including multi-core processors and graphics processing units (GPUs), researchers can perform more complex and detailed simulations than ever before. This improved capacity allows for more realistic modeling of thermodynamic systems, accounting for intricate interactions that were previously too computationally intensive to analyze.
- The availability of cloud computing resources has democratized access to significant computational power, enabling smaller research teams and institutions to engage in advanced simulation projects.
- Parallel computing techniques can decrease simulation time, allowing for more extensive and quicker iterations in research. This can lead to faster experiment cycles and quicker insights, which are crucial in fast-paced industries like pharmaceuticals and energy.
- Enhanced visualizations and real-time monitoring of simulations improve understanding among interdisciplinary teams, facilitating collaboration and the sharing of findings.
"The integration of machine learning with thermodynamic simulation has the potential to radically transform the landscape of scientific and industrial research."
Research in this domain will likely lead to more refined simulations, opening doors to innovations that were once thought out of reach. The convergence of AI, machine learning, and advanced computational techniques is set to redefine how we approach complex thermodynamic challenges.
Ending
The conclusion of this article serves to synthesize the key insights about thermodynamics simulation and its implications across various scientific fields. It is essential to recognize the intricate relationship between thermodynamics and simulation methodologies. By summarizing the core concepts discussed, readers can appreciate how simulated models provide essential insights into real-world thermodynamic processes.
Recap of Key Points
- Definition and Importance: Thermodynamics is a cornerstone of physics and engineering. The simulation of thermodynamic systems enhances understanding of both fundamental and complex phenomena. It allows practitioners to predict behavior under various conditions.
- Simulation Types: Different approaches, such as molecular dynamics, Monte Carlo methods, and computational fluid dynamics, have unique applications that cater to specific research needs. Each method contributes to a refined analysis of thermodynamic behaviors.
- Software Tools: A variety of software platforms are available for conducting thermodynamic simulations. These tools range from commercial solutions like ANSYS and COMSOL Multiphysics to open-source alternatives such as OpenFoam. Knowing the strengths and limitations of each enhances the effectiveness of simulations.
- Applications and Impact: The practical implications of thermodynamics simulations span numerous industries, from energy and aerospace to environmental science. Their role in research, especially in material sciences, cannot be overstated. It aids in explores complex interactions and designs for improved materials.
- Challenges and Future Directions: Despite the advancements, challenges remain, such as complexity in modeling. Continuous research is vital. Exploring synergies with artificial intelligence and machine learning can greatly enhance the predictive capabilities of simulations, pointing towards a promising future.
Significance of Continued Research in This Field
Continued research in thermodynamics simulation is crucial for several reasons. As industries face increasingly intricate systems and sustainability challenges, there is a pressing need to develop more accurate models. Research fosters innovation in methodologies, leading to better simulations that can capture realities of thermodynamic processes more closely.
Moreover, integrating new technological advances, such as machine learning algorithms, into simulation processes can unveil patterns and insights that are not evident through traditional approaches. This integration opens new avenues for exploration, allows for efficient data processing, and can ultimately save time and resources in both academic and industrial settings.
Additionally, investing in research ensures that the simulation tools remain relevant and adaptive to the needs of diverse fields. The ability to simulate and analyze thermodynamic phenomena will underpin advancements in energy solutions, materials technology, and even climate science. Thus, fostering a culture of inquiry and innovation in thermodynamics simulation not only expands the field but also catalyzes practical solutions to contemporary challenges.