Exploring the Significance of Absolute Data
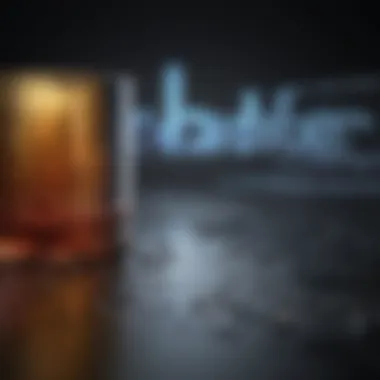
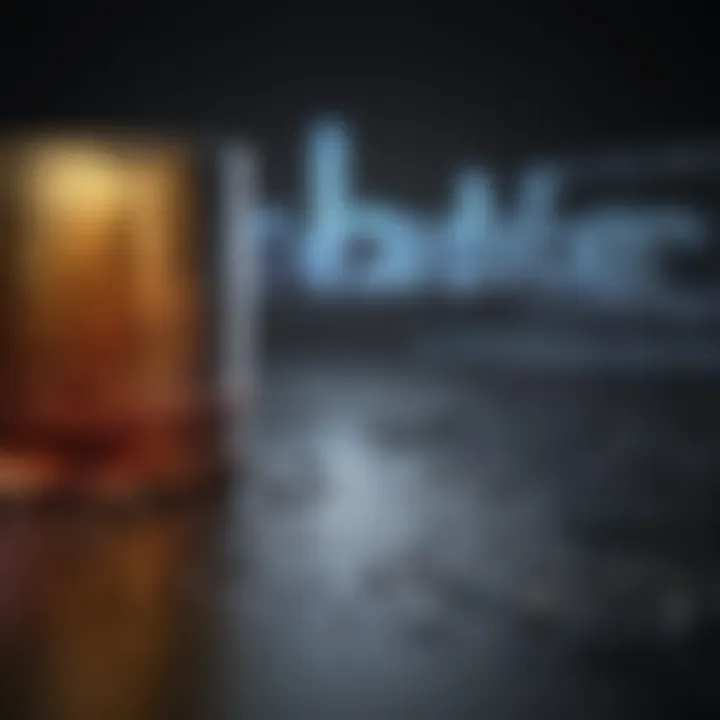
Intro
In today’s data-driven world, the concept of absolute data has emerged as a critical focus for researchers, analysts, and decision-makers. As we navigate the complexities of various scientific disciplines and technological applications, the need for uncompromised accuracy has never been more pronounced. Absolute data refers to information that is free from any modifications or subjective interpretations. Its integrity is essential for ensuring reliability in conclusions drawn from data analyses.
This article aims to explore absolute data comprehensively, offering insights into its definition, significance, and the methods used to obtain it. By juxtaposing absolute data against relative data, we can appreciate the role it plays in fostering informed decision-making processes. Furthermore, potential limitations and ethical implications associated with its use will be examined. In doing so, we will gain a clearer understanding of how absolute data enhances the quality of scientific inquiry and maintains information integrity.
Research Overview
Summary of Key Findings
Absolute data contributes significantly to enhancing the reliability of research outcomes. Our exploration into this aspect reveals the following key points:
- Absolute data delivers consistent and verifiable information.
- It aids in establishing scientific principles and theories through well-documented evidence.
- Contrasts with relative data, which can lead to misinterpretations.
These findings underscore the indispensable role absolute data plays in various research fields, ensuring that conclusions are rooted in solid, verifiable truth rather than subjective assessments.
Importance of the Research
Understanding absolute data goes beyond merely defining the term. Its necessity is relevant in several domains, especially where precision is paramount.
- In scientific research, it forms the basis for experiments and findings.
- In technology, it supports the foundation upon which algorithms and models operate.
- In clinical settings, it can mean the difference between effective treatment and misdiagnosis.
The ripple effects of using reliable absolute data are profound. They instill confidence in research outputs and foster trust in the methods used to obtain data. As the landscape of data science continues to evolve, acknowledging the value of absolute data will be essential for future advancements.
Methodology
Study Design
To delve into absolute data, a well-structured study design is necessary. This involves:
- Defining the criteria for what qualifies as absolute data.
- Selecting relevant fields for analysis, such as scientific research, technology, and clinical studies.
Such a design paves the way for systemic examination of data integrity.
Data Collection Techniques
Effective data collection methods ensure that the gathered information is accurate and free from bias. Common techniques include:
- Surveys: Implementing structured questionnaires aimed at retrieving specific data points without distortion.
- Experiments: Controlling variables to ensure that results are attributable solely to the independent variable.
- Observational Studies: Collecting data without interference, allowing for genuine behavior or phenomena analysis.
These techniques serve as the backbone for compiling absolute data, ensuring that the outcomes reflect true values and relationships. The culmination of these methods guarantees the integrity of the data collected, which is vital for any reliable research and decision-making processes.
“The essence of absolute data lies in its unyielding nature, standing as the benchmark against which all other data types may be measured.”
Through this analysis, we establish the importance of absolute data in maintaining high standards in scientific research and discussions surrounding decision-making. This exploration takes us one step closer to ensuring that decisions made in crucial contexts are informed by the most reliable data possible.
Understanding Absolute Data
Understanding absolute data is crucial in the realm of data science and research. As the landscape of digital information evolves, the distinction between absolute and relative data becomes more significant. Researchers, educators, and professionals must grasp these concepts to enhance their analytical approaches and improve the integrity of their findings.
Absolute data serves as a foundational element in many scientific inquiries. It provides a benchmark that is not influenced by external variables, allowing for unbiased assessments and comparisons. This quality is particularly important when accuracy is paramount, such as in experiments that require precise measurements or in fields like environmental science and healthcare. Without a solid understanding of absolute data, researchers may overlook critical factors that could significantly affect their results.
The benefits of comprehending absolute data extend beyond accuracy. Understanding this concept helps in making informed decisions. Researchers can better design studies, interpret results, and apply findings in a real-world context. Given its critical implications, exploring the definition and origins of absolute data is fundamental for those engaged in scientific work.
Definition of Absolute Data
Absolute data refers to information that is factual and derived from direct observation or measurement without any modifications or interpretations. This data type stands in contrast to relative data, which often requires comparison to other values or data points. Absolute data provides a definitive measure or count, thus representing a clear and undeniable fact.
For example, the chemical composition of a substance can be represented as absolute data. If a scientist measures the concentration of a particular element in a solution, that measurement is absolute as it is based on direct analysis. Unlike relative data, which might say something like, "This is twice as concentrated as another solution,” absolute data eliminates uncertainty and allows for concrete conclusions.
Origins and Development
The concept of absolute data has evolved alongside advancements in measurement and analytical techniques. Historically, early studies relied on subjective observations, which often led to inconsistencies and inaccuracies. As scientific methods matured, especially during the Renaissance and the Enlightenment, the importance of precise measurement became evident. Techniques such as controlled experimentation put emphasis on obtaining absolute measures.
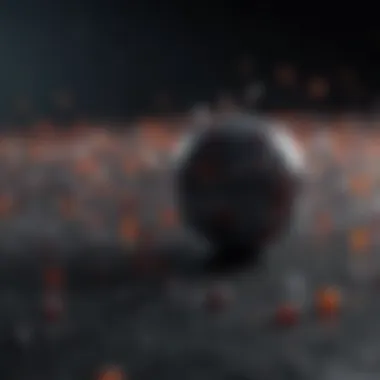
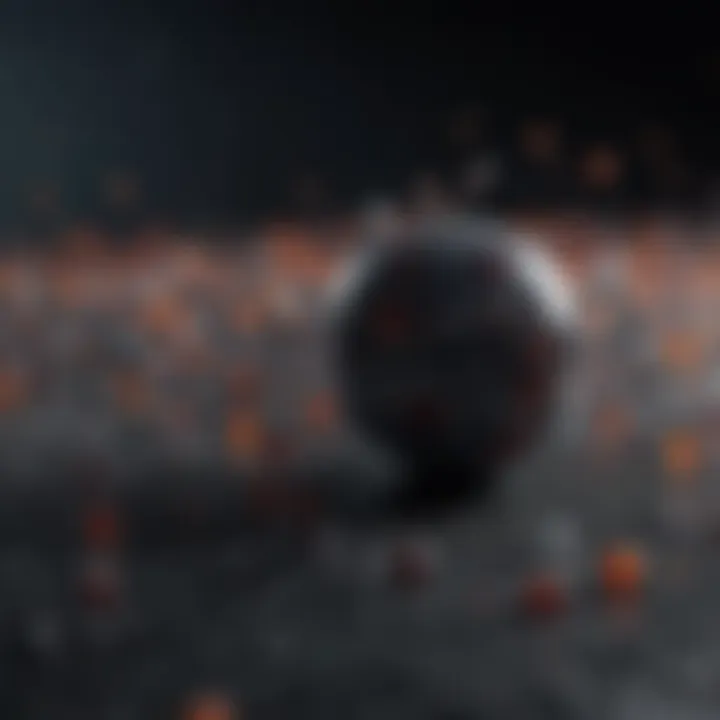
Through the 19th and 20th centuries, technological innovations played a pivotal role in refining the process of data collection. Instruments such as spectrometers and statistical analysis tools allowed scientists to gather and interpret absolute data with greater precision. The rise of data science in the digital age has further propelled the significance of absolute data, as vast amounts of information can now be analyzed consistently and accurately. The development of software programs that adhere to strict data validation procedures ensures that absolute data remains paramount in contemporary research practices.
In the next sections, we will delve deeper into its importance, methodologies for collecting absolute data, and its applications across various fields. Understanding these aspects will provide valuable insights into why absolute data is indispensable.
The Importance of Absolute Data
Understanding the importance of absolute data is essential in today's data-driven environment. Absolute data provides concrete, unconditional facts that lead to improved decision-making and greater certainty in conclusions drawn from research. These qualities are critical across a range of sectors, including science, finance, and technology. This section sheds light on these aspects, focusing on absolute data's implications for scientific inquiry and its role in ensuring accuracy and reliability.
Implications for Scientific Research
Absolute data serves as the foundation for scientific research. The integrity and validity of any study hinge on the quality of the data collected. In contexts where precision is crucial, like clinical trials or environmental assessments, reliance on absolute data helps researchers avoid biases that relative data may introduce.
Researchers benefit from using absolute data in a few key ways:
- Enhanced Precision: Absolute data offers a fixed point of reference. It minimizes variables that can skew results, making it easier to replicate studies and validate findings.
- Clear Comparisons: Scientific investigation thrives on comparisons. Using absolute metrics allows for clearer delineations among groups or conditions, which is pivotal in hypothesis testing.
- Robust Framework for Analysis: With absolute data, researchers can utilize advanced statistical methods more effectively. This supports more reliable interpretations of results, contributing to sound conclusions and recommendations.
Role in Data Accuracy and Reliability
The role of absolute data in achieving accuracy and reliability cannot be overstated. Absolute data provides a level of certainty that other forms of data may not offer. This certainty is indispensable for professionals who rely on precise information for critical decision-making processes.
Several factors highlight how absolute data enhances both accuracy and reliability:
- Elimination of Ambiguity: By focusing on fixed metrics, researchers can avoid the pitfalls of subjective interpretations. This leads to more straightforward, unbiased results.
- Consistency across Studies: When absolute data is employed, it establishes a consistent framework that other studies can reference. This consistency aids in the construction of a larger body of knowledge, as findings become more integrable.
- Informed Policymaking: Accurate data leads to well-informed decisions in public policy, health, and environment management. It provides a trustworthy basis on which policymakers can enact changes or allocate resources effectively.
"Absolute data empowers researchers and decision-makers by abolishing uncertainty in context where lives and resources are at stake."
Methodologies for Collecting Absolute Data
The methodologies for collecting absolute data are foundational in ensuring the quality, accuracy, and reliability of research outcomes. Understanding these methods streamlines the process of data acquisition and ensures that researchers are basing their conclusions on solid evidence. In the context of absolute data, methodologies can be broadly divided into quantitative approaches and qualitative techniques. Each type offers unique benefits and considerations, which will be detailed below.
Quantitative Approaches
Quantitative approaches are critical when dealing with absolute data. These methods rely on numerical data and statistical analysis to draw conclusions. The primary goal is to quantify the problem or phenomena being studied, allowing researchers to identify patterns, correlations, and causal relations. Methods such as surveys, experiments, and observational studies fall under this category.
For instance, using algorithmic models or statistical software can help analyze data collected from controlled environments. These approaches typically yield data that can be measured and compared directly. This reduces ambiguity concerning findings.
Benefits of Quantitative Approaches:
- Strength in drawing statistical inferences
- Ability to process large volumes of data efficiently
- Potential to generalize findings across larger populations
However, these methods come with specific considerations. Researchers must ensure proper sampling techniques to avoid bias. The variability in the data must also be understood to provide meaningful insights.
Qualitative Techniques
Qualitative techniques provide a different lens for collecting absolute data. These methods focus on understanding phenomena through observation, interviews, and content analysis. While qualitative data may not be easily quantifiable, it captures the meaning, context, and characteristics that numbers alone cannot express. This approach helps contextualize absolute data in real-world settings.
Interviews, focus groups, and ethnographies are a few examples of qualitative methods. Such techniques can reveal nuances that inform more comprehensive data interpretations.
Benefits of Qualitative Techniques:
- Deep insight into participant experiences and perspectives
- Flexibility in data collection, adapting to the evolving context
- Ability to explore complex issues in detailed ways
Yet, it is vital to recognize that qualitative data can introduce subjectivity, affecting how findings are interpreted. Researchers should strive to enhance rigor through techniques like triangulation, ensuring multiple data sources support conclusions.
In summary, the methodologies for collecting absolute data serve as the backbone of any solid research framework. Combining both quantitative and qualitative methods can enhance reliability and provide a more holistic view of the topics investigated. The character of the data collection methods influences the effectiveness of deriving absolute insights, making this aspect critical in the realm of scientific research.
Comparative Analysis: Absolute vs. Relative Data
The comparative analysis between absolute and relative data is essential in understanding their roles in data collection and interpretation. Each type of data serves distinct purposes in research and analysis, offering various benefits and challenges. Discerning the characteristics of absolute and relative data can significantly improve analytical accuracy and the relevance of findings. This section explores what relative data signifies and highlights key differences between the two forms of data.
Understanding Relative Data
Relative data refers to information that is interpreted in relation to other data points. It often conveys a type of context about the circumstances or conditions surrounding a specific measurement. Relative data can help in comparing values, identifying trends, and providing a more nuanced understanding of processes. For example, percentages, ratios, and indices are commonly used forms of relative data. They allow analysts to grasp the relations among variables rather than the raw integers themselves.
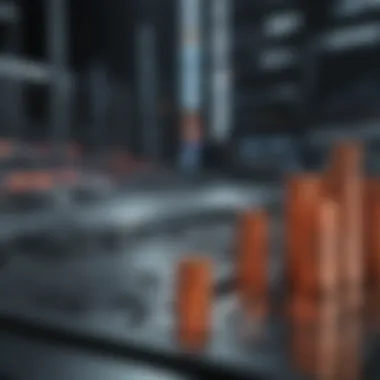
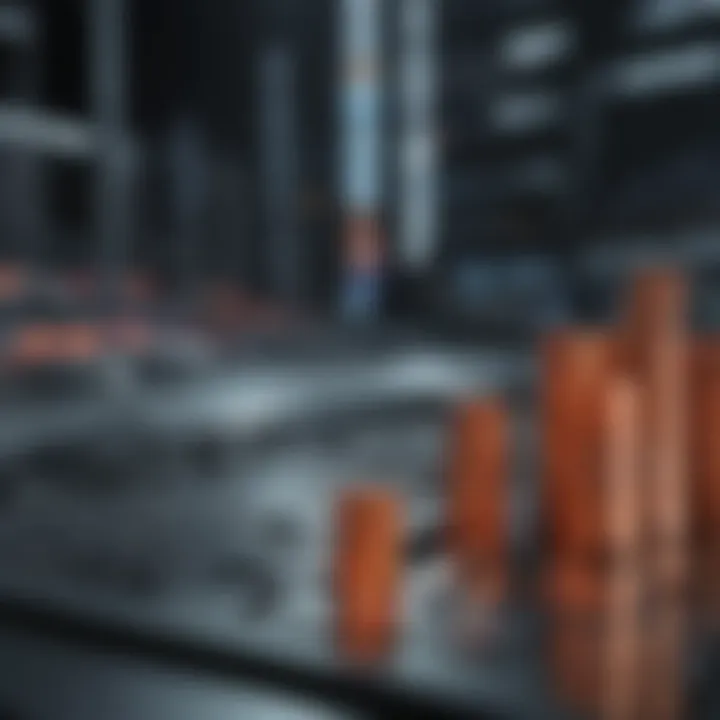
In many cases, relative data is more accessible and easier to interpret for various audiences. It enables a clearer understanding of how one variable affects another or how a specific outcome relates to a broader context. However, relative data may obscure the inherent value of absolute data, especially when precise measurements are necessary for accuracy in scientific research.
Key Differences
Delineating the differences between absolute and relative data is critical for effective use and interpretation in various fields. Here are some key distinctions that should be considered:
- Nature of Measurement
- Application
- Interpreting Findings
- Use Cases
- Absolute data provides a definitive measure, such as the exact quantity or value of a specific variable. For instance, the number of cells in a sample or the temperature reading reported in degrees Celsius.
- Relative data gives context by expressing a measurement concerning another. This could be in the form of a percentage increase or decrease over time.
- Absolute data is indispensable where specificity is paramount, such as in laboratory settings and quantitative analysis.
- Relative data often facilitates comparison across datasets, making it valuable in social sciences and market research.
- With absolute data, the interpretation is straightforward and relies solely on the recorded values.
- For relative data, interpretation can be complex since it involves analyzing how one data point fares compared to another.
- In biological research, absolute data might be used to measure precise organisms in an ecosystem, while relative data may show the proportion of species present, thus framing their ecological balance.
Understanding these differences allows researchers to choose the appropriate type of data that aligns with their study objectives. Using absolute data ensures precision in certain applications, while relative data effectively illustrates context and relationships. Balancing both types of data enriches research findings and enhances overall comprehension.
Applications of Absolute Data
The applications of absolute data are multifaceted, spanning across various fields. This section delves into how absolute data influences research and decision-making processes in ways that are both profound and practical. Understanding its applications is not just about recognizing its value but also about appreciating its transformative potential in enhancing the rigor of scientific inquiry.
Case Studies in Biological Research
In the realm of biological research, absolute data plays a pivotal role. Biological studies often demand precise measurements of parameters such as gene expression levels, enzyme activity, or cellular responses. For instance, in genomic studies, absolute data allows for direct quantification of nucleic acids, providing clarity that relative measures cannot. A case study that exemplifies this is the use of absolute quantification in polymerase chain reaction (PCR) techniques. This methodology enables researchers to determine the exact copy number of a target DNA sequence in a sample, facilitating accurate comparisons across different conditions or treatments.
Moreover, absolute data enhances reliability in clinical trials where drug efficacy is evaluated based on specific biomarkers. By providing objective measures, it aids in reducing variability and supports the replication of findings. Thus, the integration of absolute data in biological studies not only fortifies the results but also enhances the reproducibility of research outcomes, a cornerstone of scientific validity.
Use in Environmental Sciences
Environmental sciences benefit greatly from the application of absolute data. In fields such as ecology, conservation, and climatology, precise environmental measurements are crucial. For example, when assessing the impact of pollutants on marine life, researchers use absolute measurements of water quality parameters such as pH, dissolved oxygen, and nutrient concentrations. Relating these absolute figures to biological responses allows for clearer conclusions regarding ecosystem health.
Additionally, absolute data facilitates the modeling of climate change effects. Scientific models rely on precise historical climate data, such as average temperatures and precipitation levels, to predict future scenarios. This reliance illustrates that without accurate absolute data, forecasting would become less reliable. Consequently, the clarity provided by absolute data is essential for effective policymaking and environmental management, ensuring that actions are based on a solid foundation of factual evidence.
"Absolute data is not merely numbers; it is the backbone upon which credible research is constructed. The integrity of findings rests on the quality of the measurements made."
The thoughtful application of absolute data across these domains underscores its transformative role, making it indispensable for advancing both theoretical understanding and practical applications in research.
Challenges and Limitations of Absolute Data
The exploration of absolute data is essential in understanding its capabilities and restrictions within the research landscape. While absolute data serves as a cornerstone for accuracy and reliability, it is not without its own set of challenges and limitations.
Data Collection Constraints
Collecting absolute data can pose various challenges. One major issue is related to the availability of resources. In many cases, obtaining precise absolute measurements requires advanced equipment or highly trained personnel, which may not always be accessible. Furthermore, when datasets are collated over wide geographical areas, differences in conditions can lead to inconsistencies in the data collected. This needs to be acknowledged and accounted for during analysis.
Technical limitations are also prevalent. Instruments used for data collection may not always provide absolute values, particularly in fields like environmental science. For example, sensors used to measure atmospheric concentrations can be sensitive to calibration shifts and environmental influences. This can introduce uncertainty, which affects the quality of absolute data.
Additionally, time constraints can lead to rushed or incomplete data collection processes. When researchers are pressed for time, the rigor needed to ensure data integrity may suffer. This concern often highlights the trade-off between the scale of data collection and the quality of the measurements obtained.
Interpretation Challenges
Once absolute data is collected, interpreting it can present its own challenges. One primary concern is the context in which the data exists. Absolute values must be understood within the framework of the broader system they are part of. Without proper contextual knowledge, conclusions drawn from the data may lead to misleading insights. For instance, absolute temperature readings must be analyzed keeping in mind local climatic conditions.
Moreover, the sheer volume of data collected can create complexities in interpretation. Large datasets can obscure significant nuances, making it difficult to extract meaningful insights. Additionally, different methodologies and statistical approaches used to analyze absolute data can yield varying conclusions. Consequently, researchers need to be vigilant in selecting appropriate statistical methods that ensure valid interpretations.
"Absolute data possess immense value, but careful consideration in both collection and interpretation is vital to avoid pitfalls of inaccurate conclusions."
Lastly, bias can infiltrate the interpretation stages. Researchers' expectations or preferences might unconsciously influence how data is perceived. This cognitive bias can lead to selective interpretation, thus distorting the true essence of the absolute data. Vigilance against such biases is critical to uphold the integrity of research outcomes.
As absolute data continues to be a focus in various disciplines, addressing these challenges will be crucial to harnessing its full potential.
Ethical Considerations in the Use of Absolute Data
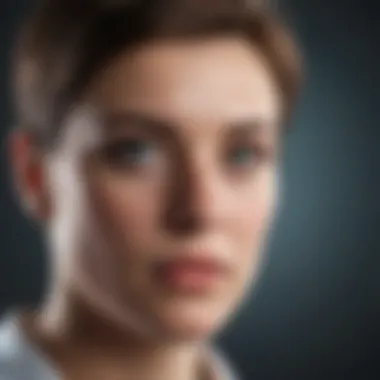
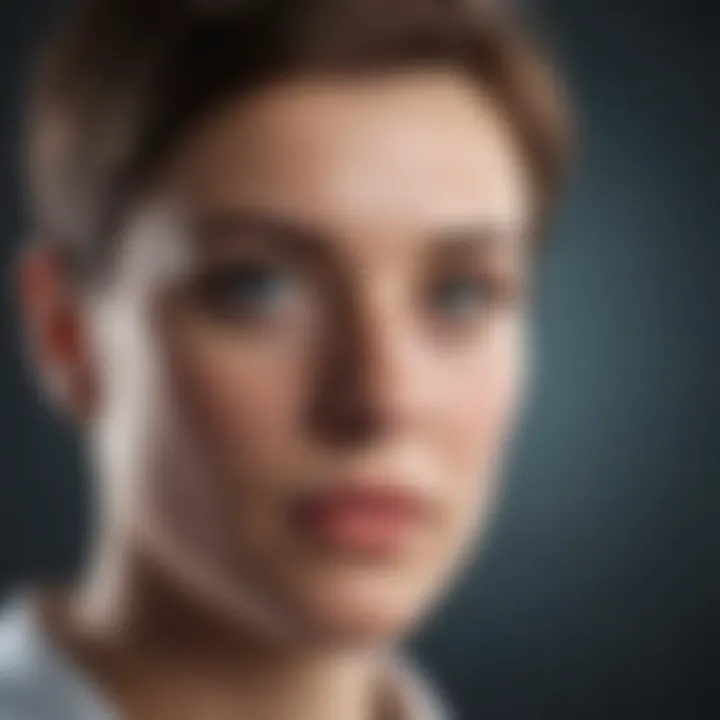
The exploration of absolute data brings forth numerous ethical considerations that are essential in today’s landscape. Researchers and data practitioners must grapple with these concerns as they navigate the complexities of data usage. Ethical practices in absolute data ultimately impact privacy, data integrity, and the broader implications of data misuse. The significance of these ethical considerations is twofold: it promotes responsible data usage and fosters trust in data-driven research and decision-making.
Privacy Issues
Privacy is a paramount concern when dealing with absolute data. As researchers collect and analyze data, the personal information of individuals often becomes part of the input. Whether it is health data, demographic information, or behavioral patterns, the risk of exposing sensitive details increases.
Among the critical aspects of privacy issues are:
- Informed Consent: Ensuring that individuals understand how their data will be used is vital. Participants need clarity about the scope of data collection and potential applications of their information.
- Data Anonymization: While collecting absolute data, anonymizing personal identifiers can help protect individual identities. This process involves stripping identifiable elements from datasets, making it challenging to link data back to specific individuals.
- Access Control: Limiting access to collected data is essential in maintaining privacy. Only authorized personnel should be able to handle sensitive information, reducing the risk of breaches.
Even with these precautions in place, there remains the potential for unintentional privacy violations. This emphasizes the need for ongoing vigilance and a proactive approach to privacy in absolute data management.
Data Integrity and Misuse
Data integrity refers to the accuracy and reliability of data throughout its lifecycle. Incomplete or manipulated data can lead to significant consequences in research outcomes, policy decisions, and stakeholder trust. The integrity of absolute data is crucial, primarily because decisions based on flawed information can have far-reaching effects.
Key considerations regarding data integrity and misuse include:
- Validation of Data Sources: It is imperative to ensure that data originates from reputable sources. Researchers should conduct thorough evaluations of their data collection methods and sources to validate the quality of absolute data utilized.
- Ethical Data Handling: Practicing ethical principles in data management can prevent misuse. This includes avoiding data fabrication, maintaining transparency about data limitations, and providing accurate reporting on findings.
- Consequences of Misuse: When data is manipulated for personal gain or to mislead stakeholders, it undermines the credibility of research and erodes public trust. Addressing this challenge requires robust ethical guidelines and accountability mechanisms that enforce high standards.
"The integrity of data hinges on the principles of honesty and transparency, which are catalysts for trust among users and stakeholders."
In summary, ethical considerations in absolute data involve a delicate balance between utilizing data for research while safeguarding personal privacy and ensuring data integrity. These principles not only protect individuals but also enhance the credibility and reliability of research findings, creating a more informed and trustworthy environment for scientific inquiry.
Future Directions in Absolute Data Research
The area of absolute data is evolving rapidly, driven by advancements in technology and growing recognition of its significance across various domains. Future directions in this field promise to enhance the robustness of research methodologies and expand the usability of absolute data. By focusing on technological advancements and exploring expanding applications, researchers can develop more effective strategies for harnessing absolute data in ways that improve decision-making and integrity in research.
Technological Advancements
Technological innovations are paving the way for improvements in how absolute data is collected, analyzed, and interpreted. One of the most impactful developments is the integration of artificial intelligence and machine learning into data collection protocols. These technologies can automate processes, improve accuracy, and reduce human errors. Moreover, they can analyze vast datasets swiftly, revealing hidden trends and correlations that may not be apparent through traditional methods.
Advancements in sensor technology also play a crucial role. Sophisticated sensors now enable real-time data collection in challenging environments, such as deep oceans or outer space, which previously posed significant difficulties for researchers. This ability not only improves the scope of absolute data but also enhances reliability, as data can be obtained more consistently.
Benefits of Technological Advancements:
- Increased automation in data collection reduces errors.
- Enhanced real-time data processing offers immediate insights.
- Improved sensor capabilities broaden research possibilities.
Expanding Applications
The potential applications of absolute data are continually expanding, influenced by emerging fields and interdisciplinary approaches. In healthcare, for example, the integration of absolute data can lead to more personalized medicine. By combining absolute data on genetic makeup with environmental factors, healthcare professionals can tailor treatments to individual needs, enhancing effectiveness.
In environmental sciences, absolute data is crucial for modeling climate change impacts. Researchers can gather precise measurements of greenhouse gas emissions, water quality, and biodiversity, facilitating a better understanding of ecological changes and supporting policy discussions.
Areas such as urban planning and smart cities also benefit from absolute data. By utilizing detailed measurements of urban emissions and traffic flows, planners can develop more sustainable cities and optimize resources effectively.
"Absolute data serves as a foundational pillar for making informed decisions in various fields. Its continued evolution is crucial for science and policy-making."
Examples of Expanding Applications:
- Personalized healthcare solutions based on genetic and environmental data.
- Climate change models utilizing precise environmental metrics.
- Urban planning strategies informed by comprehensive urban data.
Ending and Implications
The conclusion of this article highlights the essential role that absolute data plays in various domains, especially within scientific research and analytics. Understanding absolute data is critical for advancing knowledge and developing reliable data-driven decisions. As we have discussed, absolute data enables researchers and professionals to make precise measurements and analysis, thus fostering a greater degree of accuracy and reliability in findings.
The implications of adopting absolute data are broad. It not only enhances the integrity of research but also influences how results are interpreted and applied. In practical applications, it aids scientists in drawing valid conclusions while mitigating the risk of misinterpretation. It promotes a more solid foundation for evidence-based decision-making within organizations.
Additionally, as we look toward the future, the integration of absolute data will likely become more significant. Embracing advancements in technology can further refine the methodologies of capturing and analyzing this data, thereby reinforcing its relevance.
Thus, the adoption of absolute data and understanding its implications creates a robust platform for future research, innovation, and high-quality analysis, establishing a direct impact on sectors that rely on accurate data.
Summation of Key Points
- Absolute data provides a reliable foundation for scientific inquiry.
- Enables accurate measurement and analysis of phenomena.
- Critical for making informed, evidence-based decisions.
- Technological advancements will enhance methodologies for data collection.
Final Thoughts on Absolute Data's Role
In summary, absolute data holds a pivotal position in the framework of modern research and analytics. Its significance cannot be understated; it is not merely about gathering data but ensuring that what is collected is dependable and can be utilized effectively across various sectors. The ethical considerations surrounding absolute data must also be carefully navigated, ensuring privacy and integrity.
Future research should continue to explore absolute data's potential applications. As we harness technological advances, the role of absolute data will certainly evolve, offering new possibilities and refining existing methodologies. Thus, its contribution to academic and practical fields will likely expand, making it an enduring focal point for research and professional practices.