Applications of Machine Learning in Various Fields
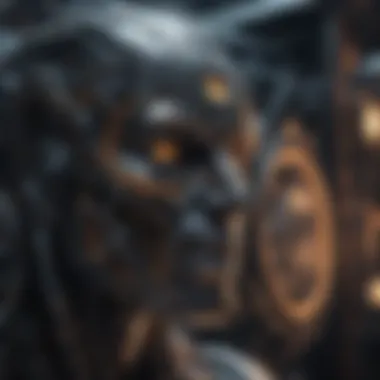
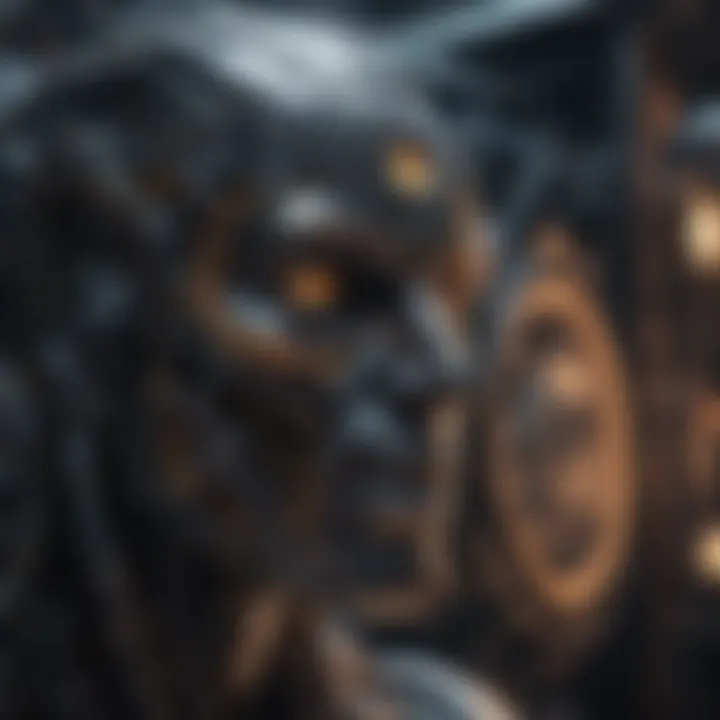
Intro
Machine learning, a branch of artificial intelligence, has rapidly evolved over recent years, becoming an integral part of a multitude of industries. This technology isn’t simply a buzzword; it’s reshaping how we interact with data, make decisions, and approach problems in our daily lives. From predicting patient outcomes in healthcare to driving investment decisions in finance, machine learning models are stepping in where traditional methods often fall short.
Consider this: When your smartphone suggests the next word in a text or when an e-commerce site recommends products tailored just for you, machine learning is silently at work behind the scenes. It learns from patterns in data, refining its algorithms to improve accuracy over time. However, the discussion surrounding this technology goes beyond mere functions; it raises critical issues of ethics and practical applications that merit a deeper exploration.
Research Overview
Summary of Key Findings
The breadth of machine learning applications is nothing short of astonishing. Key areas explored include:
- Healthcare: Improving patient care by predicting disease progression or personalizing treatments.
- Finance: Automating and optimizing trading strategies based on vast datasets and real-time analysis.
- Technology: Enhancing user experience through intelligent interfaces and better data management.
Each field presents unique challenges, yet the underlying principles of machine learning—learning from data, making predictions, and adapting over time—remain consistent.
Importance of the Research
Understanding machine learning's impact today is not just for tech experts. It’s a necessity for students, researchers, educators, and professionals across various domains. The growing reliance on machine learning technology makes it imperative to grasp its implications, benefits, and limitations. This knowledge can empower individuals and organizations to harness its potential effectively while navigating the ethical concerns that come along with it.
Methodology
Study Design
The exploration of machine learning applications is informed by a multifaceted study design that includes both qualitative and quantitative research. Data is gathered from case studies, industry reports, and academic research papers that demonstrate the effectiveness and challenges of implementing these algorithms in real-world scenarios.
Data Collection Techniques
Various techniques are employed to gather data on machine learning's effects:
- Literature Review: Analyzing a wide range of scholarly articles and technical papers.
- Surveys: Collecting opinions and experiences from practitioners in different fields.
- Case Studies: Reviewing successful implementations of machine learning in companies to draw insights.
Through this comprehensive methodology, the article aims to provide valuable takes on how machine learning is utilized across sectors and what practitioners can expect moving forward.
Prelims to Machine Learning
Machine learning stands at the crossroads of computer science, statistics, and practical applications that touch nearly every sector of our lives. As an essential part of artificial intelligence, it enables systems to learn from data and improve over time without explicit programming. This capability to adapt and evolve in response to new information is what makes machine learning a game changer in today's technology-driven world.
Despite the technical complexity often associated with the term, machine learning can be understood in simpler terms. It’s about harnessing data to make predictions, explore patterns, and often automate processes that would otherwise require human cognition. Understanding these foundational concepts is vital, especially as we dive deeper into specific applications and implications of the technology.
The myriad applications of machine learning have proliferated across diverse industries, ranging from healthcare, where algorithms assist in diagnostics, to the financial sector, enhancing risk assessment. Grasping the breadth of these uses is crucial for any student or practitioner wishing to navigate the current landscape. Moreover, as we engage with machine learning, we invite critical conversations around data ethics, the integrity of algorithms, and the evolving relationship between humanity and technology.
Furthermore, this section aims to highlight some key elements and benefits:
- Adaptability: Machine learning algorithms improve as they process more data, enhancing both precision and relevance.
- Automation: Routine tasks can be automated, allowing human effort to focus on more complex issues.
- Decision Support: They provide substantial data insights, facilitating better decision-making across various domains.
Acknowledge that while enthusiasm for machine learning can be high, it's equally important to explore its historical context. Understanding its evolution sheds light on how we arrived at current methodologies and frameworks, helping us gauge where we might head in the future.
Defining Machine Learning
To define machine learning, one may start with its core premise: the use of algorithms and statistical models that allow computers to perform tasks without explicit instructions. Instead of programming specific actions, a machine learning model uses data to identify patterns. For instance, in a classification task, a model learns from historical data to distinguish between different types of outcomes—like categorizing emails as either spam or not.
By employing techniques such as supervised learning, unsupervised learning, and reinforcement learning, the system learns through experience. Supervised learning relies on labeled datasets, while unsupervised learning looks for hidden structures in unlabeled data. Reinforcement learning, on the other hand, involves training an agent to make sequences of decisions by rewarding it for beneficial actions.
Historical Context
The roots of machine learning trace back to the early days of computer science. It’s fascinating to look at how the theories evolved. In the 1950s, the concept of artificial intelligence started blooming, with pioneers like Alan Turing proposing foundational ideas. Turing’s work on machine learning concepts laid the groundwork for machines to learn from their mistakes. Fast forward to the 1980s, when researchers began developing more sophisticated algorithms capable of learning from intricate datasets.
The introduction of the internet in the 1990s and 2000s was a turning point. The explosion of data availability transformed machine learning approaches, making it easier for practitioners to implement and test algorithms. Thus, concepts that were once theoretical saw practical application in fields like finance and healthcare.
Today, machine learning's historical journey serves as a testament to human ingenuity and its ability to adapt. Recognizing this context enriches our understanding of not merely the what but the why behind the technology's rapid evolution.
Applications in Technology
In today’s fast-paced world, the applications of machine learning (ML) in technology stand out as pivotal enablers in various sectors. This section sheds light on the transformative role of machine learning, illustrating how its integration delivers efficiency, enhances decision-making, and ultimately drives innovation. The adoption of machine learning technologies leads to increased accuracy and productivity while minimizing costs across industries. From optimizing performance metrics to unlocking new avenues for growth, machine learning’s multifaceted nature revoluttionizes how businesses operate.
Artificial Intelligence Integration
Machine learning serves as a backbone for artificial intelligence, fostering the development of intelligent systems that can learn, adapt, and make decisions independently. The integration of AI technologies powered by machine learning enables automatic responses in real-time, enhancing user experiences and operational efficiencies. For instance, virtual assistants like Google Assistant and Siri are capable of understanding natural language and providing tailored responses based on users' queries.
This interplay not only streamlines processes but also allows organizations to harness vast amounts of data, turning what was once complex into manageable insights. Such capabilities render machine learning indispensable in the modern technological landscape. In sectors ranging from customer service to logistics, AI-integrated solutions have become synonymous with increased operational agility and smarter resource allocation.
Industry-Specific Applications
Manufacturing
In the manufacturing sector, machine learning algorithms facilitate predictive maintenance, a critical aspect that reduces operational downtime and cuts costs. By analyzing sensor data from machinery, manufacturers can anticipate equipment failures before they occur. This approach not only minimizes loss of productivity but also extends the lifespan of physical assets, showcasing a significant return on investment.
The efficiency found in manufacturing settings stems from ML’s unique ability to analyze trends over time. The incorporation of autonomous robots for assembly lines has revolutionized production processes, delivering goods at remarkable speeds while ensuring higher quality control.
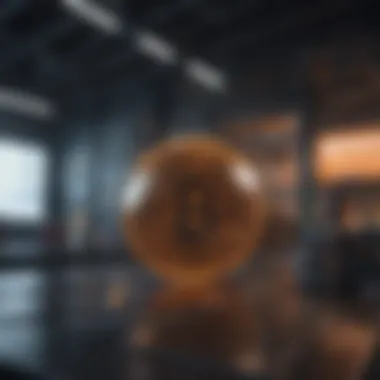
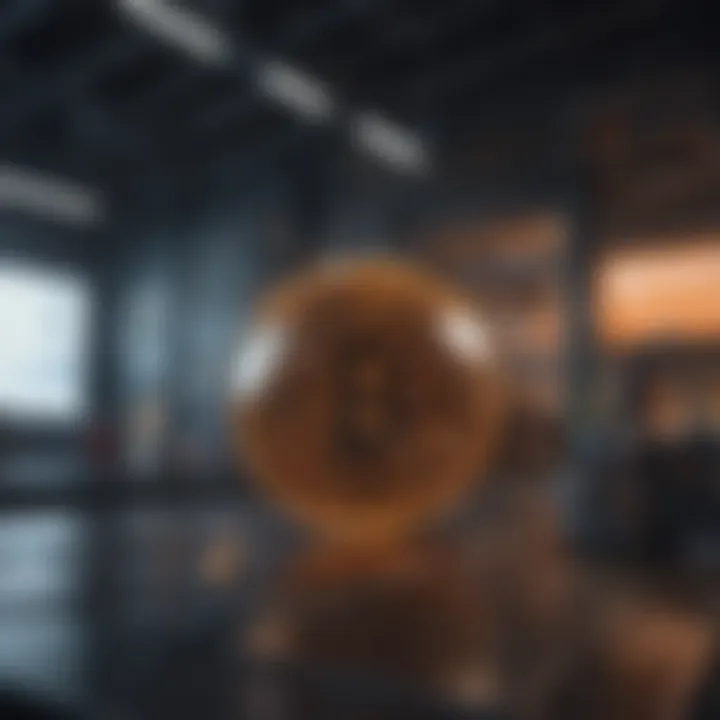
Retail
Machine learning’s role in retail has changed the game entirely. From personalized shopping experiences to enhanced inventory management, the technology provides valuable insights that drive sales strategies. Retail giants like Amazon utilize sophisticated algorithms to analyze customer behavior, allowing for recommendations tailored to individual preferences. This deep understanding can significantly improve customer satisfaction and loyalty.
However, as beneficial as these features are, they also require retailers to navigate challenges around data privacy. The balance between utilizing data and respecting consumer rights is a key consideration in the retail sector. Overall, ML applications in this space demonstrate both opportunity and responsibility.
Telecommunications
The telecommunications industry leverages machine learning to optimize network performance and enhance customer service. By analyzing call data, operators can improve service quality and identify fraudulent activities with greater precision. Additionally, machine learning aids in the development of chatbots and automated responses that improve customer interaction, reducing wait times and increasing satisfaction.
Telecommunication companies are often faced with vast datasets accumulated from millions of customers. The ability to process this information using machine learning not only provides a competitive edge but also enables more informed strategic planning. Yet, challenges such as the rapid evolution of technology and changing consumer expectations require continuous adaptation and innovation.
"The integration of machine learning into various technologies is not just about efficiency; it is about fundamentally reshaping how industries interact with information and adapt to changes."
In summary, the applications of machine learning across different sectors highlight its crucial role in facilitating transformative changes within technology. By fostering innovations and enhancing operational efficiencies, machine learning becomes an indispensable tool for businesses aiming for growth in an ever-evolving landscape.
Machine Learning in Healthcare
The healthcare sector stands on the brink of a revolutionary shift, and much of this transformation can be attributed to machine learning. From improving diagnostics to tailoring treatment plans, machine learning harnesses vast amounts of data to deliver solutions with stunning precision and efficiency. The benefits are not just limited to simple tasks but extend far beyond into critical areas affecting patient outcomes and operational efficiency.
Machine learning applications in healthcare bring several key benefits:
- Enhanced Decision-Making: It can help medical professionals draw upon detailed data analytics to make informed decisions, which often leads to better patient care.
- Resource Optimization: Hospitals and clinics can enhance their resource allocation, minimizing waste while maximizing service delivery.
- Predictive Modeling: By identifying trends and patterns in health data, machine learning can make accurate predictions about disease outbreaks or patient deterioration.
In the face of all these advantages, considerations such as data privacy and ethical use of technology must be taken seriously. Let's dive deeper into specific applications within the field: predictive analytics, personalized medicine, and medical imaging.
Predictive Analytics
Predictive analytics in healthcare is like having a crystal ball, forecasting everything from patient needs to potential health risks. Algorithms analyze historical data to predict future events, ultimately improving preventative healthcare measures.
For instance, hospitals can analyze emergency department visit data to predict peak times and prepare accordingly, thus ensuring timely treatment. Such predictive capacity aids in preventing escalated health issues by identifying at-risk patients early on and intervening before dire situations emerge.
- Key Benefits of Predictive Analytics:
- Reduced readmission rates through early intervention.
- Improved management of chronic diseases by monitoring patients continuously.
- Enhanced patient outcomes via tailored interventions based on predictions.
Personalized Medicine
Personalized medicine is where the gems of machine learning truly shine. It’s not merely about treating diseases but knowing precisely how to treat each individual. By analyzing genetic information, lifestyle, and environmental factors, machine learning models can recommend personalized treatment plans aimed at optimizing therapeutic outcomes for each patient.
For example, an algorithm may analyze a tumor's genetic markers and suggest a specific chemotherapy regimen that is likely to be more effective. This approach not only improves the chances of success but also reduces the chances of side effects, ensuring patient care remains at the forefront.
- Key Aspects of Personalized Medicine:
- Tailored therapies minimize trial-and-error methods in treatment.
- Increased patient adherence to treatment protocols because they see tangible benefits.
- Continuous learning from ongoing data improves future recommendations.
Medical Imaging
The realm of medical imaging has also undergone a metamorphosis fueled by machine learning. Traditional imaging methods, while invaluable, are reliant on human interpretation, which can vary greatly between practitioners. Machine learning enhances this by applying algorithms capable of identifying patterns within imaging data that may be overlooked.
For instance, algorithms are trained on vast datasets of X-rays, MRIs, and CT scans to identify anomalies with incredible accuracy. This not only expedites the diagnostic process but also addresses interpretation inconsistencies among healthcare providers.
- Key Advantages of Machine Learning in Medical Imaging:
- Accelerated diagnostic processes lead to quicker patient management.
- Fewer misdiagnoses and improved identification of early-stage diseases.
- Continuous training of AI models as more data becomes available allows systems to improve over time.
"The real power of machine learning in healthcare lies not just in better algorithms, but in the extraordinary potential to enhance human judgment, leading to optimal patient care."
In summary, machine learning presents an incredible spectrum of applications in healthcare that promise better outcomes, faster treatments, and personalized care. As we continue to explore the various dimensions of this evolving field, it remains crucial to balance the benefits with ethical considerations to ensure that technology serves humanity in the most responsible manner.
Financial Sector Transformations
The financial sector is undergoing a significant metamorphosis, primarily fueled by the incorporation of machine learning. The integration of such advanced technology is reshaping operations, improving decision-making, and enhancing customer experiences across various financial institutions. In this section, we will peel back the layers of three critical applications of machine learning within finance: fraud detection algorithms, algorithmic trading, and risk analysis models.
Fraud Detection Algorithms
Fraud detection represents one of the most vital applications of machine learning in finance. The financial world is rife with opportunities for deception, making it crucial for institutions to detect and prevent fraudulent transactions in real-time. Traditional methods often fall short, relying heavily on manual processes and static rules that can be easily circumvented by savvy fraudsters.
Machine learning introduces dynamic algorithms that learn from past transaction data to identify unusual patterns and behaviors. For instance, a credit card company can train a model on thousands of transaction vectors—amounts, location, time, and vendor details—to create a baseline of what a legitimate transaction looks like. When a transaction deviates significantly from this baseline, an alert can be triggered for further investigation.
These algorithms not only enhance accuracy but also significantly reduce false positives. This means fewer legitimate transactions are flagged, leading to better customer satisfaction. As the system evolves with emerging data, its capability to recognize fraud patterns becomes sharper over time.
"In the fight against fraud, using machine learning is like having a detective who never sleeps, always analyzing, learning, and adapting to new methods of deceit."
Algorithmic Trading
The realm of algorithmic trading has seen a seismic shift with the embrace of machine learning. This practice involves using complex mathematical models to make trading decisions at lightning speed. Investors and trading firms are turning to machine learning to sift through vast datasets, identify market trends, and execute trades with uncanny precision and timing.
Here, machine learning models can analyze historical price trends, global news, and even social media sentiment in order to predict future price movements. For example, a hedge fund might utilize a combination of supervised and unsupervised learning techniques to evaluate the correlation between economic indicators and market fluctuations. As a result, these models can learn and refine their predictions continuously, enhancing trading strategies.
The competitive edge gained through algorithmic trading is not just about speed; it's also about making informed decisions with data that a human trader, overwhelmed by information, might easily miss.
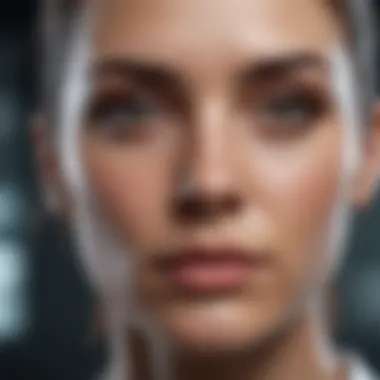
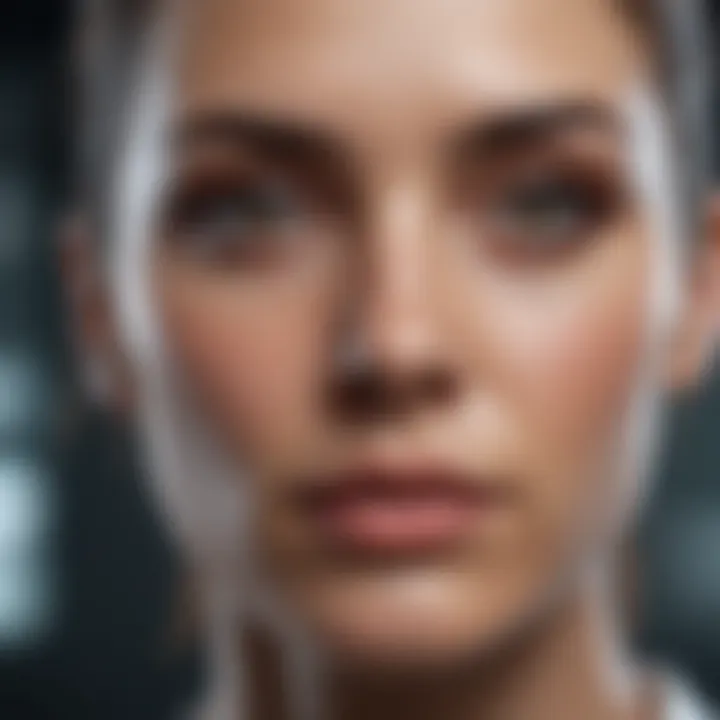
- Improved efficiency: Trades can be executed faster and with minimal human intervention.
- Data-driven insights: Enhanced analyses lead to better investment decisions.
- Scalability: Algorithmic systems can manage multiple portfolios simultaneously.
Risk Analysis Models
Risk management is another domain where machine learning’s impact reverberates within finance. Understanding and mitigating risks are fundamental components of any financial transaction. Traditional risk assessment models often rely on historical data and simplistic assumptions, which may leave room for vulnerabilities in liquidity, credit, or market risks.
Machine learning models, however, consider a wider array of variables and complex interactions. They can evaluate vast datasets in real-time, identifying patterns that indicate risk more effectively. A bank, for example, might deploy a machine learning model to assess a client's creditworthiness by examining not just credit scores, but also transaction history, spending habits, and broader economic indicators.
This nuanced approach allows financial institutions to create a more comprehensive risk landscape and tailor their offerings accordingly.
- Adaptive learning: The models continuously learn from new data points.
- Enhanced precision: Risks previously unnoticed are flagged for immediate attention.
- Customization: Insights enable tailored financial products for diverse client needs.
Machine Learning in Natural Language Processing
Understanding the integration of machine learning in natural language processing (NLP) is vital to grasping the broader picture of how these two fields intertwine to enhance communication and information processing. NLP serves as a bridge between human languages and machine understanding, making it an essential area for developing intelligent systems capable of human-like interaction. As people increasingly rely on technology for communication, the implications of advancements in NLP become significant. The benefits extend across various sectors, from customer service automation to enhancing accessibility for people with disabilities.
Speech Recognition
Speech recognition technology stands as a cornerstone of natural language processing. It enables machines to convert spoken language into text. The real magic happens through algorithms that analyze audio signals and can distinguish words, dialects, and special phrases. For instance, in personal virtual assistants like Apple’s Siri or Google Assistant, speech recognition allows users to command and interact with their devices hands-free.
One striking example is how this technology benefits those with mobility challenges, allowing them to communicate effortlessly through voice commands. Such applications highlight the human aspect of machine learning in NLP, demonstrating its potential beyond traditional computing tasks. Yet, challenges persist, particularly in dealing with accents and background noise, which can skew results. Manufacturers are continuously refining these algorithms to improve accuracy and responsiveness.
Sentiment Analysis
Sentiment analysis, another key element of machine learning in NLP, focuses on understanding and interpreting emotions behind texts. From social media platforms to customer feedback systems, businesses leverage sentiment analysis to gauge public opinion about their brands. By analyzing phrases and context, systems can determine whether the sentiment expressed is positive, negative, or neutral.
The significance of sentiment analysis is evident in marketing strategies. Companies exploit insights gleaned from this analysis to adjust campaigns, tailoring them to resonate more effectively with their target audience. It’s interesting how such systems can detect nuances and even sarcasm, evolving with the language trends users adopt. For example, platforms such as Twitter utilize this analysis to track real-time events, uncovering how the public feels about breaking news.
"Understanding the emotional undertones captured through sentiment analysis can lead to actionable insights that shape not only marketing strategies but product development and customer engagement approaches as well."
The Role in Research and Development
When we talk about the role of machine learning in research and development, it's like shining a flashlight in a dark room filled with possibilities. The advances this technology fosters are not merely incremental; they are transformative, pushing boundaries and unlocking new avenues of inquiry.
To put it plainly, machine learning acts as an invaluable ally for researchers, providing tools that can sift through mountains of data that human beings simply cannot manage on their own. In a world where data is pouring out by the gigabyte, leveraging machine learning techniques becomes crucial. These methods sharpen our ability to analyze complex datasets, enabling us to extract meaningful patterns and insights which might otherwise go unnoticed.
For a clearer view, let’s break down some of the specific benefits that machine learning brings to the table:
- Enhanced Data Processing: By employing algorithms that can learn from data, researchers save time and can focus on strategic decision-making rather than getting bogged down in the minutiae of data cleaning and preprocessing.
- Pattern Recognition: Machine learning models excel at identifying trends that may not be visible to human eyes. This can lead to breakthroughs across various fields from social sciences to molecular biology.
- Predictive Modeling: Using historical data to predict future trends allows researchers to test and validate hypotheses without extensive manual calculations.
With these advantages in mind, let’s dive deeper into two significant aspects of machine learning within R&D: data analysis techniques and simulation and modeling.
Data Analysis Techniques
When it comes to data analysis, machine learning provides a robust framework for interpreting complex data sets. One of the standout features of machine learning approaches is the capability to automate the process of data exploration. This automation enables researchers to discover correlations and anomalies that they might have overlooked.
Some commonly applied techniques include:
- Regression Analysis: This method helps in understanding relationships between variables. It can be particularly useful in fields like economics, where one seeks to understand how various indicators affect economic outcomes.
- Clustering: This technique groups data points into clusters based on similarities. For example, in genomics, it can identify groups of genes with common expression patterns, leading to new insights in biomedicine.
- Classification Algorithms: These are pivotal in categorizing data into specific classes. In natural language processing, for instance, methods like support vector machines or decision trees help in sentiment analysis.
As researchers adopt these techniques, they find that the quality of their insights improves significantly, enabling advances that are both rapid and actionable.
Simulation and Modeling
Moving on to simulation and modeling, this aspect of machine learning allows for creating digital representations of real-world processes. Think of it as building a miniature model of reality that you can manipulate and study without the constraints of physical experimentation.
Simulation plays a critical role in fields such as:
- Environmental Science: Analyzing climate data to model and predict weather patterns.
- Pharmaceuticals: Using simulations to test drug interactions and efficacy before clinical trials.
- Engineering: Creating simulations for material behavior under various stress tests, which can save time and resources in product development.
Through these simulations, researchers can experiment with variables in a controlled environment, revealing outcomes and improving designs based on comprehensive data. This iterative process ultimately enhances the quality of innovation.
"Machine learning transports research from a guesswork game to a data-driven discipline."
In summary, the integration of machine learning techniques into research and development is not just beneficial; it’s essential. The capabilities these technologies hold open countless doors for manipulation and interpretation of complex data, leading to insight and understanding that defines the cutting edge of modern research.
Challenges and Limitations
When discussing machine learning, it's vital to turn the spotlight on challenges and limitations that accompany its deployment. The excitement surrounding machine learning often tends to overshadow these concerns. Yet, understanding these obstacles is fundamental not only for developers and organizations but also for society at large. Being out in front of technological advancements means acknowledging that every rose has its thorns. The potential pitfalls can affect how we design, implement, and adapt these systems.
Data Privacy Concerns
One of the monumental issues in the world of machine learning is data privacy. As systems and algorithms evolve, the amount of personal data they require often grows as well. This raises a couple of eyebrows. Users naturally wonder, "How secure is my data?" and "Where is it being stored?"
Moreover, the recent high-profile data breaches have left a scar on public trust. When datasets used for training contain sensitive information—such as financial records or health data—it makes the stakes even higher. If such information falls into the wrong hands, folks can suffer serious consequences. This isn’t just about being cautious; it's about being responsible.
Some crucial points regarding data privacy include:
- Regulatory Compliance: Organizations must navigate a complex landscape of rules like GDPR or CCPA. Not adhering to these regulations can lead to hefty fines.
- User Consent: Securing informed consent from users is not merely a formality anymore. It's an ethical necessity. Users should know what data is collected and how it will be used.
- Anonymization Techniques: While anonymization methods exist, they sometimes don't fully protect against re-identification, particularly when combined with other datasets.
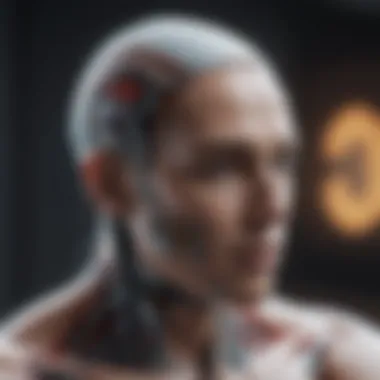
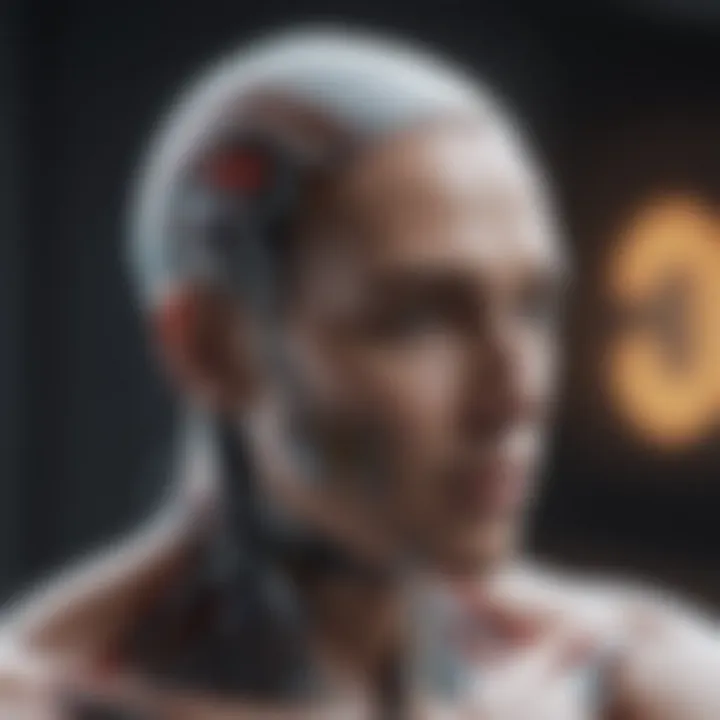
To quote a study from the University of California,
"As machine learning applications proliferate, so too do the risks associated with data misuse, making the need for stringent privacy measures both urgent and critical."
Bias in Machine Learning Algorithms
Another critical concern is the bias inherent in machine learning algorithms. Algorithms learn from the data they are fed, and if that data reflects human societal biases, it paves the way for skewed outcomes. When biases creep into algorithms, they can reinforce stereotypes or lead to systemic discrimination, which is the last thing anyone wants.
- Sources of Bias: Bias can originate from various sources, including:
- Impact of Bias: For example, biased algorithms may result in unfair outcomes in hiring practices or lending decisions, reinforcing existing inequalities.
- Mitigation Strategies: Some potential solutions include:
- Historical data that reflects past prejudices.
- Incomplete or unrepresentative training data.
- Regular algorithm audits to assess fairness and transparency.
- Diverse teams in the development process can minimize blind spots during implementation.
Addressing bias isn't just a nicety; it's a necessity. As algorithms increasingly guide decisions in critical areas such as law enforcement and loan approvals, the implications of bias can have far-reaching consequences. To turn a blind eye is to risk perpetuating rather than solving societal issues.
In summary, it is crucial to approach the realm of machine learning with a lens of caution. The promise of machine learning is robust, but understanding the challenges and limitations ensures we can harness its potential responsibly.
Ethical Considerations
As machine learning continues to mold our future, the ethical landscape surrounding its applications grows more complex and significant. In this section, we will navigate the essential elements of accountability, transparency, and the impact this technology has on employment. Understanding these dimensions is vital for anyone engaged in, or affected by, the development and deployment of machine learning systems.
Accountability and Transparency
Accountability in machine learning involves being responsible for the outcomes of automated systems. Algorithms can inadvertently perpetuate biases or lead to harmful outcomes if not carefully monitored. Thus, systems should be structured in a way that tracks both decision-making processes and subsequent results. This means establishing mechanisms that allow stakeholders to seek redress or explanations when adverse decisions are made based on these algorithms.
Transparency in machine learning is about shedding light on how models operate and make decisions. For example, consider a loan application process that uses machine learning to evaluate creditworthiness. If the algorithm declines an application without a clear rationale, the interested party may feel unjustly treated. By making algorithms interpretable, users can understand the reasoning behind decisions, which builds trust in technology.
- Key components for enhancing accountability and transparency include:
- Clear documentation of the models used and their training data
- Ongoing audits to monitor for biases and accuracy
- Easily accessible information for end-users
Emerging practices like explainable AI aim to address these issues, providing clearer insights into how models work without oversimplifying their complexities.
"In a world where algorithms steer much of our daily lives, accountability and transparency can't just be afterthoughts; they need to be foundational principles."
Impact on Employment
The advent of machine learning stirs considerable discussions about its influence on the job market. On one hand, automation could make various roles obsolete, particularly in sectors like manufacturing and services. For instance, machine learning algorithms can perform tasks faster and often with greater accuracy than humans, which could lead to fewer job opportunities for certain skill sets.
On the other hand, it's crucial to recognize that machine learning also creates new roles. Jobs centered on managing, designing, and overseeing these systems are rapidly emerging.
- Factors to consider regarding employment include:
- The upskilling of workers in tech frameworks
- Job displacement in routine tasks
- The emergence of new job categories in AI training, ethics, and system management
As machine learning expands, a balance is needed between harnessing its efficiency and safeguarding livelihoods. This nuanced perspective sheds light on the realities of how technology either disrupts or enhances the workforce. Tackling these ethical considerations thoughtfully is essential to ensure a future where technology serves humanity’s best interests.
Future Prospects of Machine Learning
The landscape of machine learning is constantly evolving, with each breakthrough revealing new avenues for exploration. As we peer into the future, the significance of understanding the prospective advancements in machine learning becomes clear. With innovations unfurling at a rapid pace, it’s critical to grasp how these advancements may reshape industries, impact society, and influence our daily lives. This section delves into the emergent technologies anticipated to arise and the broader societal implications they'll carry.
Emerging Technologies
Tech giants and startups alike are pouring resources into research that stands to redefine our interactions with technology. Here are some noteworthy trends and innovations that are likely to emerge:
- AutoML: Automation in machine learning might simplify model creation and tuning, making it accessible to non-experts and increasing its adoption across various sectors.
- AI and Edge Computing: By processing data nearer to the source, edge computing could dramatically enhance a machine learning system's responsiveness. This will allow for real-time decision-making in sectors such as manufacturing and healthcare.
- Federated Learning: This approach keeps data decentralized, enhancing privacy while still allowing for the training of robust models. As privacy concerns continue to loom, federated learning may become essential to data-centric AI design.
- Explainable AI (XAI): As machine learning gets more entrenched in decision-making frameworks, there’ll be a pressing need for clarity. Explainable AI aims to provide insights into how machine learning models make decisions, fostering trust amongst users.
"Machine Learning is no longer just a trend; it is becoming the backbone of innovative solutions across the globe."
These emerging technologies, backed by ongoing research, are not only advancing the capabilities of machine learning but are also setting the stage for its integration into everyday applications.
Societal Implications
The ripple effects of machine learning advancements extend far beyond the confines of technology. They carry profound societal implications, warranting careful consideration. Here are a few critical aspects to ponder:
- Job Displacement and Creation: While it's a fact that machine learning can automate various tasks, it also promises to create new job categories. The challenge lies in equipping the workforce with the skills needed to adapt to these changes.
- Inequality of Access: As machine learning becomes a cornerstone of many sectors, there's a risk that its benefits could disproportionately favor those already in privileged positions. Ensuring equitable access to these technologies is a challenge that stakeholders must address urgently.
- Dependency on Technology: With increased reliance on machine-driven decisions, there might be an emerging sense of dependency, leading society to question decisions made by autonomous systems.
- Ethical Governance: As we forge ahead with machine learning applications, there’s a pressing need for frameworks that govern its use. Ensuring accountability and fairness in AI systems is paramount for healthy societal function.
Epilogue
The conclusion serves as a pivotal moment in any comprehensive examination of machine learning and its varied applications. It wraps up extensive discussions, creating a cohesive understanding of the insights gained throughout the article. In this section, essential elements such as the impressive capabilities of machine learning across sectors, including technology, finance, and healthcare, are summarized. This reinforces the transformative potential of this field.
Summary of Key Takeaways
To map out the key insights gathered, the essence of machine learning can be encapsulated as follows:
- Diverse Applications: From enhancing product recommendations in retail to boosting diagnostic methods in healthcare, machine learning finds myriad uses.
- Innovative Mechanisms: Understanding how algorithms learn from vast datasets is crucial for grasping their applications.
- Ethical Implications: The rise of machine learning raises important questions regarding transparency and employment, which must not be overlooked.
- Challenges: Recognizing data privacy concerns and bias in algorithms highlights the roadblocks teams must address.
- Future Trends: Staying informed about what the future holds for emerging technologies helps professionals prepare for upcoming changes.
"Machine Learning is not merely a tool; it’s a doorway into new realms of possibility that redefines boundaries across industries."
The Path Forward
Looking ahead, the trajectory of machine learning seems promising yet complex. As researchers, educators, and practitioners tap into the potential of this technology, it’s equally important to tread thoughtfully. Key considerations heading forward include:
- Continuing Education: As the landscape evolves, ongoing training and education will be necessary to keep pace with innovations.
- Interdisciplinary Collaboration: Encouraging collaboration between technologists, ethicists, and industry professionals can yield robust solutions to the challenges faced.
- Regulatory Frameworks: Establishing guidelines and standards will ensure ethical practices in developing and implementing machine learning applications, balancing innovation with responsibility.
- Public Engagement: Cultivating open discussions about machine learning, its benefits, and drawbacks will raise awareness and help mitigate public fears surrounding its use.
In summary, the journey into the practical applications of machine learning will not only pivot on its ability to innovate but also on the preparedness of society to embrace and guide its growth harmoniously.