Cohort Studies: Insights, Examples, and Impact
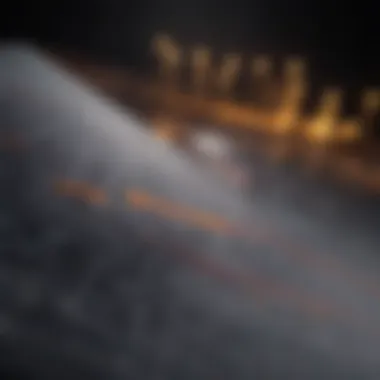
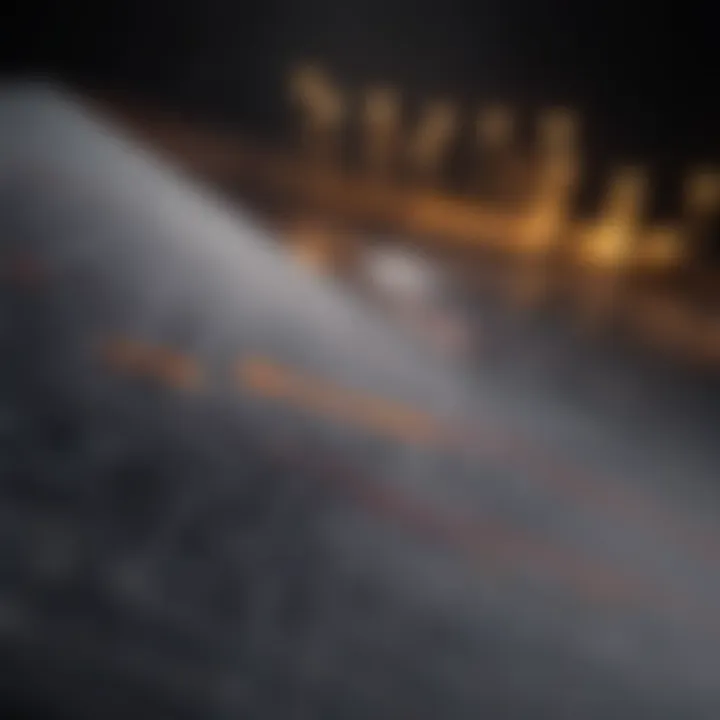
Intro
Cohort studies are a fundamental aspect of epidemiological research, enabling researchers to investigate the relationships between specific exposures and their potential outcomes over a given period. These studies are designed to follow groups of individuals who share common characteristics. They allow the evaluation of how risks and health outcomes evolve, providing extensive insights into public health issues.
The diverse applications of cohort studies range from understanding chronic diseases like diabetes to exploring the impact of lifestyle choices on mental health. Their relevance has become even more pronounced in settings where establishing a cause-and-effect relationship is critical. By delineating the timeline of exposure and outcome, cohort studies can yield valuable data.
Through this article, we will navigate the intricate landscape of cohort studies by examining key components and methodologies, along with their implications for public health policies. The examination of real-world examples will illustrate both the efficacy and the limitations inherent in this research approach. Ultimately, this discourse aims to enrich the understanding of cohort studies within the broader framework of epidemiological research.
Research Overview
Cohort studies carry out a vital role in the field of epidemiology. They offer a structured way to analyze how exposures, such as smoking, diet, or environmental factors, influence health outcomes over time. This section encapsulates the essence of cohort research.
Summary of Key Findings
Evidence from cohort studies has uncovered numerous associations which highlight the complex interactions between lifestyle and health. For instance, a prominent cohort study demonstrated that long-term exposure to air pollution correlates with increased respiratory diseases. Another notable finding from a large cohort study indicated that moderate exercise significantly reduces the risk of cardiovascular diseases. Such findings not only bolster existing literature but also guide clinical practices and policymaking.
Importance of the Research
Understanding cohort studies is essential for several reasons:
- They provide long-term data that can track changes in health outcomes.
- Findings from these studies can influence preventative measures in healthcare.
- Cohort studies inform public health recommendations and policies, aiming for higher rates of disease prevention.
These studies serve as a bridge between theoretical knowledge and practical application, underscoring the value of a robust research design in producing meaningful public health outcomes.
Methodology
This segment delves into the structural aspects of cohort studies, emphasizing the design choices and data collection methods that underpin their success.
Study Design
Cohort studies are categorized mainly into two types: prospective and retrospective.
- Prospective Cohort Studies: Researchers follow a group of individuals over time, collecting data on exposures before any outcome occurs.
- Retrospective Cohort Studies: This approach involves looking back at existing records to identify exposures and outcomes that have already occurred.
The choice of study design depends significantly on research questions, available resources, and time constraints. Each has its strengths and weaknesses, with prospective studies generally being more robust in establishing temporal relationships.
Data Collection Techniques
Data collection in cohort studies is a meticulous process. Varying techniques can be employed:
- Surveys and Questionnaires: These tools gather subjective data regarding individuals' health behaviors and exposures.
- Medical Records: Researchers often review medical histories to obtain objective data about outcomes.
- Biological Samples: Blood tests or other biological data can help in understanding physiological changes linked to exposures.
The integration of different data collection techniques enhances the validity of findings, ensuring a comprehensive picture of health outcomes.
By examining the methodological frameworks of cohort studies, we gain insight into the rigorous processes that drive epidemiological research.
Preface to Cohort Studies
Cohort studies are integral in the landscape of epidemiological research. They provide significant insights into the relationships between exposures and various outcomes over time. Understanding how these studies function is crucial, especially for researchers, policymakers, and healthcare providers. These studies offer a framework that allows for the investigation of health trends and the efficacy of interventions. They serve as a basis for developing public health strategies and clinical guidelines.
Cohort studies are distinct due to their longitudinal design, often following a group of individuals who share common characteristics. This design allows researchers to observe changes and outcomes in health over time. The advantages of cohort studies include the ability to establish temporal relationships and to quantify risks associated with certain exposures. At a basic level, knowing these key elements can guide various stakeholders in making data-driven decisions that potentially impact large populations.
Definition and Importance
A cohort study is defined as a research strategy that follows a group of individuals who share a common characteristic, usually over an extended period. This approach allows researchers to monitor the individuals for the development of specific outcomes, such as diseases or health conditions. The unique aspects of cohort studies lie in their ability to provide real-time data on the effects of exposures, whether they be environmental, behavioral, or biological, on health outcomes.
The importance of cohort studies cannot be overstated. They play a pivotal role in identifying risk factors and potential causes of diseases. Moreover, the findings from cohort studies can be influential in public health policies and strategies aimed at disease prevention. In essence, they are foundational tools in epidemiology, offering insight crucial for understanding population health dynamics.
Background of Epidemiological Research
Epidemiological research has evolved significantly over the years. Traditionally, studies relied more heavily on observational designs without the robust frameworks seen in modern cohort studies. The development of cohort studies marks a turning point, enabling researchers to investigate associations methodically and systematically.
The origins of cohort studies can be traced back to the early 20th century when public health officials began to recognize the importance of studying populations over time. High-profile studies, such as the Framingham Heart Study, set the stage for future research by demonstrating how lifestyle factors could influence heart disease. This evolution reflects an increasing awareness of the need to link observations with quantifiable outcomes.
As health issues become more complex, the ability to follow populations over time helps address new questions that arise from emerging health trends. Cohort studies continue to be a vital instrument in epidemiology, aiding in translating observations into actionable health policies.
Cohort studies are instrumental for deriving causal inference in health outcomes, providing clarity on the long-term effects of exposures.
Understanding the evolution of cohort studies gives insight into their value in research today. As challenges in public health increase, the methods and findings from cohort studies can guide effective interventions and inform future studies.
Types of Cohort Studies
Understanding the different types of cohort studies is essential for appreciating their unique contributions to research in epidemiology. These studies can be broadly classified into two categories: prospective and retrospective studies. Both types have distinct features, benefits, and limitations, which warrant careful consideration based on the research objectives as well as the practical constraints related to the study.
Prospective Cohort Studies
Prospective cohort studies are designed to follow participants forward in time. In this type of study, researchers identify a group of individuals, known as the cohort, who share certain characteristics or experiences. The cohort is then observed over a set period to assess how various exposures affect health outcomes.
Key Elements:
- Identification of Participants: Participants are selected based on specific inclusion and exclusion criteria to ensure the cohort is as homogeneous as possible. This aids in reducing bias.
- Follow-Up: As time progresses, researchers collect data regarding health outcomes and potential risk factors. This may involve regular check-ins, surveys, or medical examinations.
- Data Analysis: The analysis often involves the comparison of health outcomes in those exposed to certain risk factors versus those who were not exposed. This makes it clearer to understand causal relationships.
Benefits:
- Clear Temporal Sequence: Observing participants over time allows for a clear understanding of how exposures precede outcomes.
- Reduced Recall Bias: Since data is collected at the time of exposure, reliance on participant recall is minimal.
- Flexibility: Researchers can focus on multiple outcomes as well as many different exposures.
However, prospective studies require significant resources, time, and commitment from participants, which can also lead to challenges related to retention and sample size.
Retrospective Cohort Studies
Retrospective cohort studies, on the other hand, look backward in time. Researchers use existing records to identify a cohort that has already been formed based on specific criteria. The goal is to determine how prior exposures affect current health outcomes.
Key Elements:
- Use of Historical Data: Data is drawn from medical records, databases, or previous surveys. This provides a wealth of information without the delays associated with prospective studies.
- Outcome Assessment: Researchers evaluate outcomes that have already occurred, often using information from the health records of participants.
Benefits:
- Efficiency: These studies can be conducted more quickly than prospective studies, as data collection relies on already existing records.
- Cost-Effective: They usually require fewer resources, which can make them more feasible in instances where funding is limited.
- Useful for Rare Outcomes: Retrospective studies can be particularly valuable in situations where outcomes are rare, leveraging historical data to identify the cohort exposed to risk factors.
However, these studies come with pitfalls, such as reliance on the accuracy and completeness of existing records, which can introduce bias into the findings.
"Whether prospective or retrospective, the type of cohort study selected must align with the research questions, available resources, and the type of data needed for analysis."
Designing a Cohort Study
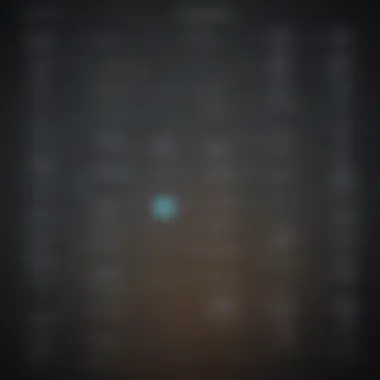
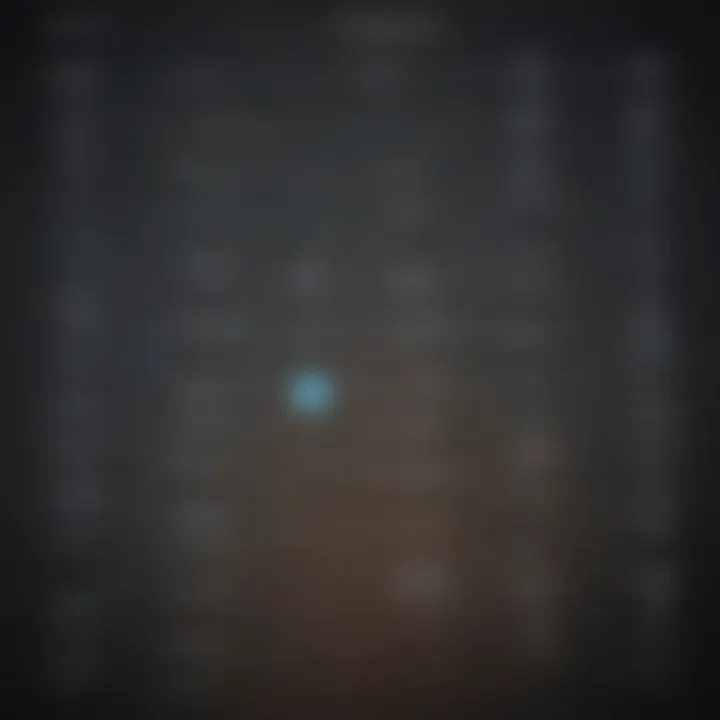
In the realm of cohort studies, the design phase is critical. A well-designed cohort study allows for accurate findings that can influence public health and policy. It involves several key elements that ensure the study's validity, reliability, and relevance. Proper consideration of these aspects allows researchers to draw meaningful conclusions about the relationship between exposures and outcomes over time.
Defining the Cohort
Defining the cohort is the first step in the design process. A cohort consists of individuals who share a common characteristic, such as age, health status, or participation in a specific activity. Clear identification is necessary to ensure the cohort represents the population being studied. This may involve:
- Demographic Factors: Age, sex, ethnicity, and socioeconomic status can all influence health outcomes.
- Health-Related Criteria: Conditions such as smoking status, existing health issues, or exposure to various environmental factors must be defined.
Defining the cohort accurately not only determines who will be included but also helps to narrow down the focus of the study, allowing for a more effective analysis.
Selection Criteria
Selection criteria play a vital role in designing a cohort study. They set the boundaries for who qualifies to be part of the cohort, impacting the overall generalizability of the findings. Key consideration should focus on:
- Inclusion Criteria: These are the characteristics participants must have to be included in the study. Examples could include specific age ranges, health conditions, or exposure to a particular risk factor.
- Exclusion Criteria: These criteria determine who is removed from the cohort to avoid confounding variables. This might include individuals with conditions that may interfere with the study’s outcomes.
Defining clear selection criteria ensures that the cohort effectively represents the target population while enabling researchers to isolate the effects being studied.
"A thoughtfully defined cohort enhances the power and accuracy of a cohort study's outcomes."
Data Collection Methods
Data collection methods are critical in cohort studies, as they form the foundation for gathering the necessary information on participants over time. The efficacy of a cohort study heavily relies on how accurately and consistently data are collected. Appropriate methods not only enhance the reliability of the findings but also influence the overall integrity of the research. Importantly, the choice of data collection methods affects the level of bias and the potential for confounding variables to skew results. Understanding different approaches helps in selecting the best fit for the specific research questions being addressed.
Surveys and Questionnaires
Surveys and questionnaires represent a direct method for obtaining self-reported information from participants. When designed carefully, these tools can provide rich data on behaviors, perceptions, and experiences related to health conditions. The advantages of this method are numerous.
- Cost-Effective: Compared to other methods like interviews, surveys can be more economical, allowing for data collection from larger populations.
- Standardization: Well-structured questionnaires can provide uniformity in responses, which is invaluable for statistical analyses.
- Anonymity: Surveys can be conducted anonymously, which may encourage honesty, especially regarding sensitive topics.
However, there are some challenges to consider. Surveys might suffer from low response rates or participant bias, where respondents may not accurately reflect their true behaviors. It's essential to pre-test these instruments to refine questions and formats. Using validated measures can also enhance the quality of the collected data.
To ensure effective data collection, researchers should invest time in designing surveys that are clear, concise, and relevant to the research objectives.
Medical Records Review
The review of medical records is an important method for collecting data in cohort studies, particularly for studying health outcomes over time. This method allows researchers to access longitudinal health data that often extends over several years. There are specific benefits associated with medical records review:
- Rich Data Source: Medical records can provide information on various health metrics, diagnoses, treatments, and responses to interventions without relying on participant memory.
- Longitudinal Tracking: Ongoing health information captured in medical records enables researchers to establish temporal associations between exposures and outcomes more effectively.
- Reduced Bias: Using clinical data can mitigate self-reporting bias, as medical records often reflect objective clinical findings.
Nevertheless, challenges exist. Accessing medical records typically involves navigating complex privacy laws and ethical considerations. Additionally, variability in record-keeping practices across institutions can affect the consistency and completeness of the data.
In summary, both surveys and medical records reviews play integral roles in data collection for cohort studies. Each method has its advantages and drawbacks, necessitating careful consideration of the research goals and target population to achieve optimal data gathering.
Statistical Analysis in Cohort Studies
Statistical analysis plays a crucial role in cohort studies. It helps researchers interpret data and draw meaningful conclusions regarding relationships between exposures and outcomes over time. The analysis can provide insights that impact public health policy, clinical recommendations, and further research directions.
Cohort studies generate vast amounts of data, leading to the necessity for comprehensive statistical techniques. These techniques serve several purposes:
- Understanding relationships: Statistical methods help in determining correlations between variables.
- Assessing risks: They can evaluate the risks associated with certain exposures.
- Enhancing validity: Proper analysis improves the validity of the study findings.
In the context of cohort studies, statistical analysis can be divided primarily into two categories: descriptive statistics and inferential statistics. Each category serves specific functions in interpreting data and understanding study implications.
Descriptive Statistics
Descriptive statistics summarize and organize data in a meaningful way. It provides a clear picture of the study participants and the characteristics being analyzed.
Key components of descriptive statistics include:
- Measures of central tendency: These are numbers that represent the center of a data set, such as the mean, median, and mode. They provide insight into typical values within the cohort.
- Measures of variability: These figures, including range, variance, and standard deviation, indicate how much variation exists in the data. They help in understanding how different or similar participants are regarding the exposure and outcomes.
- Frequency distributions: This technique shows how often certain values occur, which can reveal patterns in the data.
Descriptive statistics are essential as they lay the groundwork for further analysis. They not only describe the data but also highlight important aspects that might warrant more sophisticated evaluations.
Inferential Statistics
Inferential statistics involve making predictions or inferences about a population based on sample data. This branch of statistics is vital in cohort studies as it aids in generalizing findings beyond the observed sample.
Key elements of inferential statistics include:
- Hypothesis testing: This process evaluates assumptions about the population. Researchers can determine whether findings from the cohort sample can be inferred to a broader context.
- Confidence intervals: These provide a range of values that likely include the true population parameter. They offer a way to quantify the uncertainty that comes with sample estimates.
- P-values: These values help in determining the significance of results. A low p-value suggests that the observed results are unlikely to have occurred under the null hypothesis, providing evidence for a relationship between exposure and outcome.
In epidemiological research, statistical methods transform raw data into insights that can shape public health interventions and clinical practices.
Evaluating Cohort Study Outcomes
Evaluating outcomes in cohort studies is essential to understanding the implications of patient exposures and treatments over time. This evaluation helps uncover relationships between risk factors and health outcomes, thereby laying the groundwork for evidence-based practices. With a clear focus on both primary and secondary outcomes, researchers can gain nuanced insights that are vital for effective public health strategies and clinical practices.
Primary and Secondary Outcomes
Primary outcomes in a cohort study are the main results that investigators want to evaluate. They are clearly defined before the study starts, which aids in objective data collection and analysis. For example, in a study investigating the long-term effects of smoking on lung health, the primary outcome could be the incidence of lung cancer among participants. This outcome is crucial as it addresses the fundamental question of the study.
On the other hand, secondary outcomes provide additional information that may enhance the understanding of the primary results. These can include variables like the incidence of chronic obstructive pulmonary disease or rates of hospitalization due to respiratory issues. Including secondary outcomes allows researchers to explore other related health issues that arise from the primary exposure, enriching the analysis and implications of the study findings.
- Importance of Defining Outcomes
- Balancing Outcomes
- Clearly defined primary and secondary outcomes ensure the study remains focused and relevant.
- They facilitate effective statistical analysis which contributes to the reliability of results.
- A well-balanced study includes both primary and secondary outcomes as it provides a more comprehensive look at the potential effects of exposures.
- Researchers must carefully consider the relevance of each outcome to the main research question.
Causal Inference
Causal inference is a critical element in cohort studies, as it allows researchers to draw conclusions about the relationships between exposures and outcomes. Understanding whether a specific exposure leads to a particular outcome informs risk assessments and health policies.
In cohort studies, causal inference often relies on statistical methods. By controlling for confounding variables, researchers aim to isolate the effect of the examined exposure on the outcome. This can mean adjusting for factors such as age, sex, socioeconomic status, and other relevant characteristics that could influence results.
Causal inference not only helps in understanding the relationships in data but also plays a significant role in translating findings into practical health interventions.
- Methods for Causal Inference
- Challenges in Causal Inference
- Various statistical tests, including regression analysis, help assess the strength and significance of associations between variables.
- Techniques like propensity score matching can help account for confounding variables.
- Establishing causality is complex and not always straightforward, as correlation does not imply causation.
- Researchers must weigh the evidence carefully and consider alternative explanations for the observed associations.
In summary, evaluating the outcomes of cohort studies involves a detailed examination of primary and secondary results and the application of causal inference. These elements are integral to deriving meaningful conclusions that can influence public health decisions and clinical practices.
Examples of Notable Cohort Studies

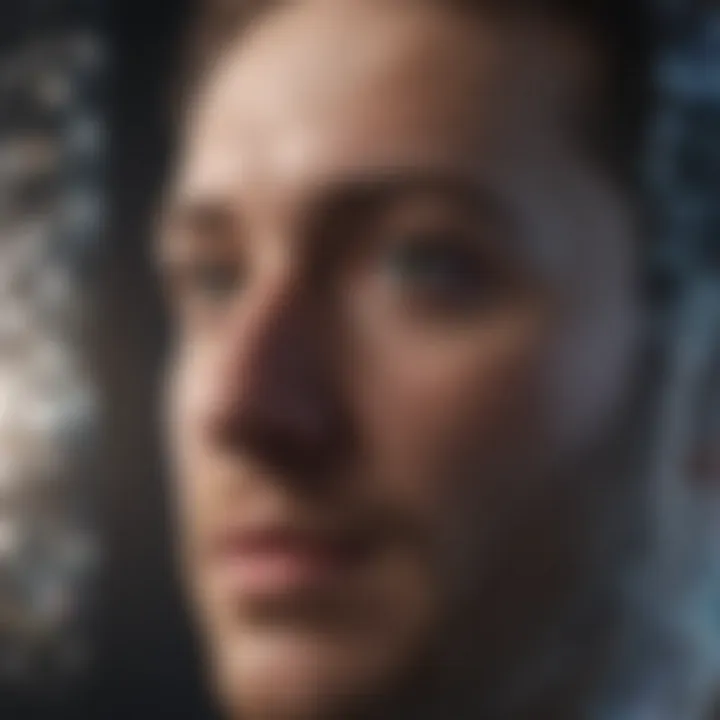
Cohort studies are essential for understanding patterns and correlations that impact public health, medical practices, and policy-making. They provide a framework for observing health outcomes across diverse populations over extended periods. Highlighting notable cohort studies illustrates their significant contributions to medical knowledge and public health policies. This section focuses on three landmark studies: the Framingham Heart Study, the Nurses' Health Study, and the UK Biobank.
Framingham Heart Study
Initiated in 1948, the Framingham Heart Study has become a cornerstone in cardiovascular epidemiology. It originally began with 5,209 participants from Framingham, Massachusetts. Researchers aimed to identify the common factors that contribute to cardiovascular diseases. Over the decades, the study has expanded to include multiple generations of participants, now encompassing thousands of subjects.
The findings from this study were groundbreaking. Notable contributions include the identification of risk factors such as high blood pressure, high cholesterol, smoking, obesity, and physical inactivity. The study has led to the formulation of various preventive strategies that are widely adopted in clinical guidelines today.
Key Considerations:
- Long-term data collection ensures the validity of health correlations.
- It serves as a model for cohort studies in other fields of health research.
“The Framingham Heart Study has revolutionized our understanding of cardiovascular health, illustrating the importance of longitudinal data.”
Nurses' Health Study
Launched in 1976, the Nurses' Health Study recruited 121,700 registered nurses to explore the impact of lifestyle on women's health. This study has been pivotal in understanding chronic diseases, including cancer, diabetes, and heart disease. Researchers collect data through biennial questionnaires addressing diet, physical activity, medication use, and other factors.
One significant achievement of the Nurses' Health Study is its contribution to our understanding of diet and health. Breakthrough research indicates a link between high trans-fat intake and increased risk of coronary heart disease, influencing dietary guidelines.
Implications:
- The study highlights the role of women in health research, often underrepresented in clinical trials.
- Findings shape policies regarding women's health across various dimensions, from reproductive health to chronic diseases.
UK Biobank
The UK Biobank represents one of the largest and most comprehensive cohort studies to date, recruiting around 500,000 participants across the UK from 2006 to 2010. This study aims to investigate the connections between genetic, environmental, and lifestyle factors on health outcomes. Participants provided detailed health data through questionnaires, physical measurements, and biological samples.
The UK Biobank's extensive database enables researchers to explore a wide range of health issues, including dementia, cancer, and cardiovascular diseases. This large-scale data collection strategy allows for the identification of subtle correlations that smaller studies may miss.
Benefits:
- Facilitates research on a scale that can address various health concerns.
- Encourages collaboration among researchers worldwide, fostering innovation in epidemiological studies.
In summary, notable cohort studies provide a wealth of knowledge that informs public health policy and clinical practices. Their intricate designs and substantial participant engagement allow for rigorous analysis of health outcomes, solidifying their importance in advancing medical science.
Challenges in Conducting Cohort Studies
Cohort studies are invaluable in uncovering relationships between various exposures and health outcomes. However, executing these studies is not without its challenges. Understanding these challenges is crucial for researchers, as they can impact the validity and reliability of study findings. The challenges discussed here include loss to follow-up and confounding variables, both of which are significant concerns in maintaining the integrity of cohort study results.
Loss to Follow-Up
Loss to follow-up is a common issue in cohort studies that can lead to biased results. It occurs when participants drop out of the study, either voluntarily or due to circumstances beyond their control. This dropout can affect the representativeness of the cohort and, consequently, the generalizability of the results.
The implications of loss to follow-up can be severe. If certain individuals are more likely to leave the study, this may skew results. For instance, if healthier participants remain in the study while those with poorer health drop out, the findings may inaccurately reflect the exposure's effects. To mitigate this issue, researchers must employ rigorous tracking and follow-up methods. Regular communication and engagement with participants can help reduce dropout rates and ensure more reliable data.
Confounding Variables
Confounding variables present another critical challenge in conducting cohort studies. A confounding variable is an external factor that may influence both the exposure and the outcome of interest. This can lead to spurious associations or obscure true relationships between exposures and outcomes.
For example, in a cohort study examining the impact of physical activity on heart health, age could act as a confounder. Older individuals might both exercise less and have a higher risk of heart disease, leading to misleading conclusions if age is not adequately controlled in the analysis.
To address confounding variables, researchers can use statistical techniques, such as stratification or multivariable regression models. These methods allow researchers to adjust for the impact of confounders, providing a clearer picture of the relationship being studied.
Ethical Considerations in Cohort Studies
Cohort studies play an essential role in understanding complex health issues and shaping health policies. However, the ethical implications of these studies cannot be overlooked. Ethical considerations ensure that the rights and welfare of participants are upheld throughout the research process. This discussion highlights two critical components: informed consent and data privacy. Understanding these aspects enriches the integrity of cohort studies and enhances the strength of their findings.
Informed Consent
Informed consent is a foundational ethical principle in research involving human subjects. This process involves providing potential participants with clear and comprehensive information about the study. The objective is to help individuals make knowledgeable decisions regarding their participation. In cohort studies, obtaining informed consent is particularly significant due to the long-term nature of these studies. Participants may be involved for many years, making it essential that they fully understand what is expected of them.
Important elements of informed consent include:
- Clear explanation of the study's purpose and procedures.
- Details about potential risks and benefits.
- Assurance of the right to withdraw from the study at any time without penalties.
- Information on how their data will be used and shared.
In essence, informed consent fosters a trusting relationship between researchers and participants. It is not merely a signed document; it is an ongoing dialogue. Researchers must emphasize transparency and respect participants' autonomy, ensuring that individuals are willing partners in the research process.
Data Privacy
Data privacy is another vital consideration in cohort studies. As researchers collect sensitive health information from participants, they must prioritize confidentiality and security. Data breaches can have serious consequences, including reputational harm, loss of trust, and legal implications.
Factors to consider regarding data privacy in cohort studies include:
- Anonymization: Researchers should remove identifiable information when analyzing data to protect participant identities.
- Secure data storage: Data should be stored in secure systems with restricted access to minimize the risk of unauthorized exposure.
- Policy compliance: Researchers need to comply with regulations, such as HIPAA in the United States, to safeguard personal health information.
In summary, ethical considerations in cohort studies are critical for maintaining participants' rights and trust. Both informed consent and data privacy ensure the protection of individuals while promoting the integrity of research findings. This ethical framework not only enhances the validity of the studies but also fosters a culture of respect and responsibility in research.
Implications of Cohort Studies for Policy Making
Cohort studies hold a significant place in shaping health policy and public intervention strategies. Their ability to track health outcomes in relation to various exposures makes them an invaluable asset for policymakers. When researchers gather data over time, they provide insights that help in understanding long-term effects of lifestyle choices, environmental factors, and healthcare practices. This can inform decisions that ultimately promote public health and well-being. Therefore, the implications of cohort studies are far-reaching, affecting both local and global health policies.
Application in Public Health
Cohort studies are instrumental in public health research. By observing a group over an extended period, these studies can highlight potential risk factors for various diseases. This information can guide public health initiatives targeted toward disease prevention. For example, data from the Nurses' Health Study has influenced dietary guidelines and smoking cessation programs, showing how diet and lifestyle impact long-term health outcomes.
Moreover, cohort studies can reveal discrepancies in health outcomes across different populations. This can help identify vulnerable groups that may require tailored interventions. Public health authorities can allocate resources more effectively based on the evidence gathered from these studies, ultimately improving health outcomes.
Key Benefits:
- Identifying Risk Factors: Cohort studies track changes and outcomes, making it easier to identify exposures associated with health risks.
- Informed Decision-Making: Policymakers can rely on robust data, ensuring that health interventions are evidence-based.
- Resource Allocation: Insights from cohort studies can drive more effective allocation of public health resources.
Influence on Clinical Guidelines
Cohort studies significantly influence clinical guidelines by providing empirical evidence regarding the effectiveness of treatments, preventive measures, and lifestyle recommendations. When studies show a clear correlation between certain behaviors and health outcomes, guidelines can be updated to reflect these insights. For instance, findings from the Framingham Heart Study led to a deeper understanding of cardiovascular disease risk factors, shaping guidelines on cholesterol management and hypertension.
This evidence-based approach is crucial for enhancing clinical practices. Healthcare professionals depend on these guidelines when making treatment decisions. A more informed approach can lead to better patient outcomes, demonstrating how vital cohort studies are in clinical settings.
Examples of Impact:
- Hypertension Management: Evidence that controlling blood pressure can decrease stroke risk has reshaped treatment protocols.
- Dietary Recommendations: The link between high sugar intake and diabetes helped refine dietary guidelines to mitigate this growing epidemic.
In summary, cohort studies have profound implications for policy-making and clinical guidelines. They not only provide substantial evidence to influence public health decisions but also enhance clinical practices, ultimately aiming for improved health outcomes across populations.
Comparative Effectiveness of Different Study Designs
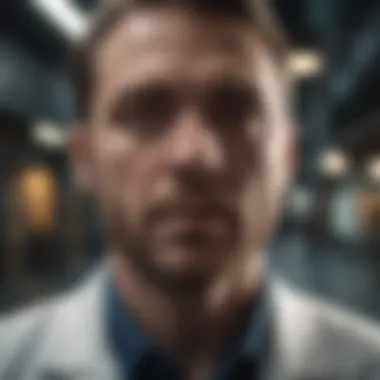
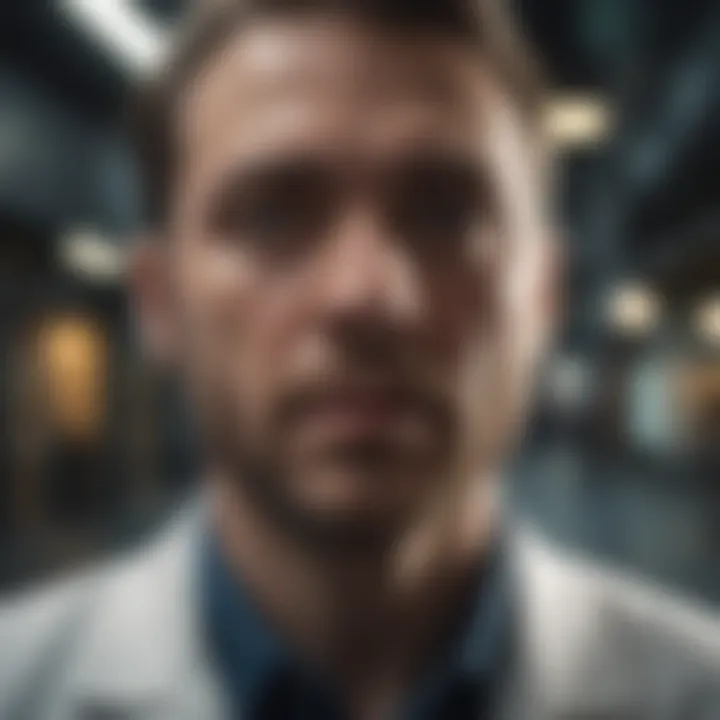
The choice of study design in epidemiological research plays a vital role in understanding causal relationships between exposures and outcomes. This section focuses on the comparative effectiveness of different study designs, specifically cohort studies, randomized controlled trials (RCTs), and case-control studies. Each of these designs has its own strengths and limitations, making it crucial for researchers to determine the most suitable method depending on their specific research questions, contexts, and available resources.
Cohort Studies vs. Randomized Controlled Trials
Cohort studies and randomized controlled trials are two prominent research methodologies in the field of epidemiology. Both have their inherent advantages and limitations.
Cohort Studies involve the observation of a group of individuals over time, differentiating between those who have been exposed to a certain risk factor and those who have not. This design is particularly beneficial for studying long-term outcomes and rare associations, as it captures data that evolves over time. One significant advantage is that researchers can assess multiple outcomes from a single exposure, often resulting in richer data.
In contrast, Randomized Controlled Trials are characterized by the random assignment of participants to either a treatment or control group. This design is widely regarded as the gold standard in clinical research for its ability to minimize confounding variables. RCTs allow for a high level of control over the experimental conditions and often produce results that are more easily generalizable.
Nevertheless, conducting RCTs can be resource-intensive and time-consuming, and they may not always be ethical or feasible, especially when it involves harmful exposures. Cohort studies stand out as a robust alternative, providing key insights in situations where RCTs cannot be implemented.
Cohort Studies vs. Case-Control Studies
The debate between cohort studies and case-control studies presents different approaches and insights. Case-Control Studies are retrospective in nature, selecting individuals based on the presence (cases) or absence (controls) of an outcome, and then looking back to determine exposure status. This design is particularly effective for studying rare diseases or outcomes, as it allows for the efficient gathering of historical exposure information.
However, a major limitation of case-control studies is the reliance on recall and historical data, which can introduce bias. Information on exposure may not be accurately remembered or may vary between cases and controls, leading to discrepancies that affect study validity.
On the other hand, cohort studies allow for a prospective evaluation of exposure prior to the outcome occurring. This can lead to more reliable data collection and potentially clearer causal relationships. The ability to track participants over time establishes a timeline of events, helping to clarify whether the exposure preceded the outcome, thus enabling stronger causal inference.
"The selection of the appropriate study design is a critical decision that profoundly affects the outcomes of research. Cohort studies often yield invaluable insights, especially in contexts where RCTs are not applicable."
Future Directions in Cohort Study Research
The future of cohort study research holds significant promise for advancing our understanding of health and disease. As technology evolves, researchers can expect to harness new tools and methodologies to enhance the quality and efficiency of cohort studies. This section delves into two key elements that are shaping the horizon of cohort studies: integrating technology in data collection and the utilization of big data.
Integrating Technology in Data Collection
The incorporation of technology in data collection is transforming cohort studies. With the advent of mobile devices, wearable technology, and online survey platforms, researchers can now collect data in real-time, improving accuracy and participant engagement. These technologies allow for better monitoring of health behaviors and exposures, which can lead to more dynamic and responsive study designs.
Moreover, technological advancements such as electronic health records (EHR) integration enable seamless access to participant data. This reduces the burden of manual data entry and minimizes errors, thereby enhancing the reliability of the research findings.
- Wearable devices can track health metrics such as heart rate and activity levels.
- Mobile applications facilitate regular data input from participants, promoting higher retention rates.
The flexibility offered by technology can also aid in recruiting diverse populations. Digital platforms can reach wider audiences, breaking geographical barriers that traditionally limited participation.
Big Data and Cohort Studies
Big data is another crucial element reshaping cohort study research. The ability to analyze massive datasets allows researchers to identify patterns and correlations that might not be apparent in smaller samples. With the right analytical tools, cohort studies can leverage big data to explore a range of outcomes across multiple demographics, thereby improving generalizability.
The integration of big data encourages a more nuanced analysis, often leading to the discovery of unexpected associations. This can provide insights into the long-term effects of exposures, enhance understanding of disease etiology, and inform predictive modeling.
"Big data analytics in cohort studies allows for unprecedented insights and can significantly influence public health strategies."
Using big data also raises important considerations regarding data management and privacy. Researchers must navigate the complexities of ethical data use, ensuring participant confidentiality while maximizing research potential.
In summary, the future directions of cohort study research are poised to take advantage of technological advancements and big data. These developments promise not only to enhance research methodologies but also to yield profound insights into health and disease, ultimately informing public health and clinical practices.
Real-World Applications of Cohort Study Findings
Cohort studies are essential tools in epidemiology, bridging the gap between research and practical applications. They generate findings that are not only academically intriguing but also relevant to public health practices and policies. The significance of cohort studies lies in the insights they provide, which directly inform health interventions and disease prevention strategies. This section discusses how these findings translate into real-world actions, emphasizing the necessity to understand and apply them effectively.
Health Interventions
Cohort studies often lead to the development and enhancement of health interventions. By observing how specific exposures affect health outcomes over time, researchers can identify which interventions are most effective in improving health conditions. For instance, studies that track the long-term health impacts of smoking have led to significant anti-smoking campaigns and legislation.
Some of the key benefits of using cohort studies in health interventions include:
- Evidence-Based Strategies: Data from cohort studies provide a solid foundation for designing interventions. They help identify at-risk populations and effective methods to reach them.
- Targeted Resource Allocation: Understanding the demographics affected by certain exposures allows for better allocation of public health resources. For example, targeting programs towards populations with higher incidences of chronic diseases can enhance overall health outcomes.
- Monitoring Trends Over Time: Cohort studies can track the effectiveness of interventions over the years, providing valuable feedback for continuous improvement.
"Cohort studies illuminate the complex interplay between exposures and health outcomes, guiding interventions that save lives."
These applications demonstrate that the implications of cohort studies extend far beyond their initial findings. They play a crucial role in informing public health guidelines and addressing health inequalities.
Disease Prevention Strategies
Disease prevention is another critical area where findings from cohort studies have profound effects. Understanding the relationship between risk factors and health outcomes allows public health officials to create effective prevention strategies. For example, cohort studies have highlighted the links between dietary habits and the development of obesity, enabling the formulation of nutritional guidelines aimed at reducing obesity rates.
Important considerations in translating cohort study findings into disease prevention strategies include:
- Primary Prevention: This involves interventions aimed at preventing the onset of diseases. For example, studies demonstrating the increased risk of diabetes due to sedentary lifestyle have paved the way for initiatives promoting physical activity among different age groups.
- Secondary Prevention: Cohort studies can identify early biomarkers for diseases, leading to screening programs. Detecting diseases like cancer early through regular screenings has shown to reduce mortality rates.
- Public Awareness Campaigns: Insights from cohort studies contribute to designing campaigns that inform the public about health risks, encouraging preventive behaviors.
By leveraging data from cohort studies, policymakers can implement targeted strategies that effectively curb the incidence of diseases. This results not only in better individual health outcomes but also in improved public health overall.
Limitations of Cohort Studies
Cohort studies offer significant insights in epidemiology and public health research. However, driving from their strengths, they also carry a set of intrinsic limitations. Understanding these limitations is crucial for researchers and scholars to assess the validity and applicability of their findings accurately. This section highlights some of the most pertinent limitations: generalizability issues and temporal ambiguity.
Generalizability Issues
One key limitation of cohort studies is their potential lack of generalizability. Generalizability refers to the extent to which findings from a study can be applied to broader populations outside of the studied group. Often, cohorts are selected based on specific criteria, which can lead to a homogenous population.
This behavior can arise during the recruitment process. For instance, studies examining chronic diseases might enroll participants from specific clinics or demographic groups. Thus, findings may not reflect the experiences of other populations, especially in diverse societies. As a result, conclusions drawn may misrepresent risk factors or health outcomes.
Moreover, the setting in which a cohort study is conducted also influences its generalizability. For example, a cohort study conducted in an urban environment may yield different results when replicated in a rural area. Therefore, researchers must be cautious in extrapolating results across varied groups and ensure appropriate context is considered when applying their findings in practice.
"The inadequacy of generalizability can lead to misguided public health strategies if results are generalised without thorough consideration of relevant differences among populations."
Temporal Ambiguity
Temporal ambiguity constitutes another significant limitation of cohort studies. It relates to the challenge of establishing a clear cause-and-effect relationship between an exposure and an outcome. In cohort studies, participants are followed over time, and outcomes are observed subsequent to exposure. However, determining the precise timing of exposure relative to the outcome can be complex.
For example, if a study investigates the linkage between diet and cardiovascular disease, it can be difficult to ascertain whether dietary habits preceded the onset of the disease or were influenced by it. This uncertainty complicates the ability to conclude whether the exposure is a causal factor or merely correlated with the outcome.
Temporal ambiguity can lead to conflicting interpretations of results. It requires researchers to be vigilant about accurately documenting both exposure and outcome timelines, which can often be an arduous task.
In summary, while cohort studies remain a valuable resource in epidemiological research, their limitations must be taken into account. Generalizability issues restrict the applicability of findings to larger populations, while temporal ambiguity complicates causal inferences, potentially misleading interpretations. Understanding these limitations allows scholars and practitioners to better evaluate the relevance and application of study results.
Ending
Cohort studies are a fundamental aspect of epidemiological research. They not only facilitate the understanding of disease patterns and health outcomes over time but also influence public health policies and practices. This article has provided a thorough examination of cohort studies, emphasizing their significance in research.
Revisiting Cohort Studies
Cohort studies have evolved as key tools in understanding health-related issues. They allow researchers to observe how different variables affect outcomes over time in a defined group. This longitudinal approach is critical, as it can help identify trends that are often missed in cross-sectional studies. The continuous tracking of participants provides rich data that can support causal inferences and help bridge gaps in knowledge. Acknowledging the strengths and limitations of cohort studies helps ensure better study design and interpretation of findings.
The Future of Research Methodologies
The future of cohort studies is likely to merge with advancements in technology and data science. As big data analytics continue to grow, cohort studies can leverage these tools for more precise data collection and analysis. Integrating digital health tools, such as wearables and mobile health applications, into cohort studies could provide real-time health data, enriching the study outcomes. Researchers need to embrace these innovations to enhance the rigor and relevance of their findings.
"The use of technology in research will not only change how we collect data but also how we visualize and interpret it."
In summary, cohort studies are invaluable for understanding health trends and informing policy. Their adaptability, especially with emerging technologies, underscores their potential in future research.