Exploring Ab Initio: Foundations and Applications
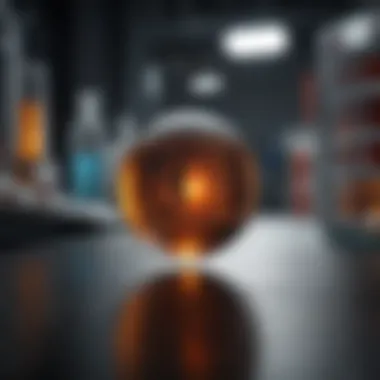
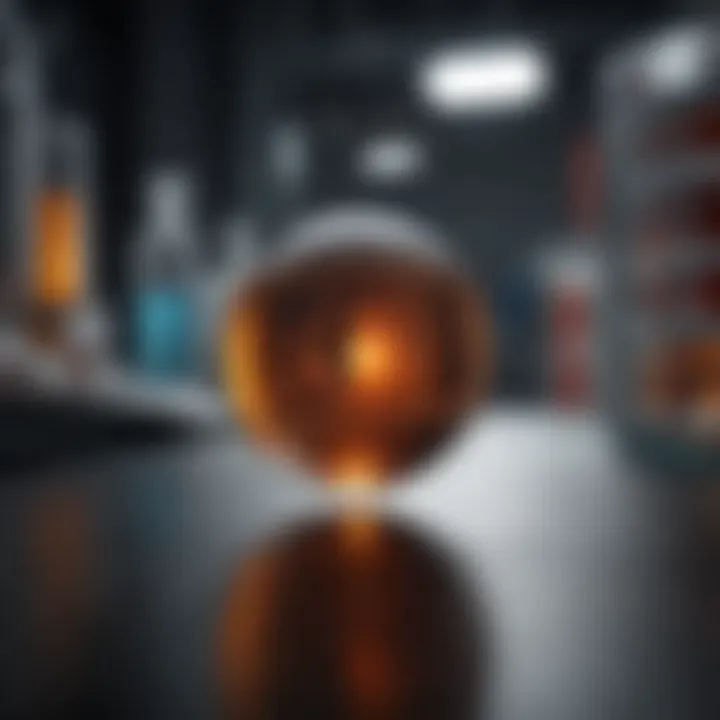
Intro
Ab initio methods are crucial in various scientific fields. The term itself originates from Latin, meaning "from the beginning". This concept implies an approach where theories and calculations are derived from foundational principles without relying on experimental data. This article aims to demystify the abundance of applications of ab initio methods in disciplines such as computational chemistry, artificial intelligence, and physics.
Exploring these methods not only enhances theoretical understanding but also drives innovations in technology. As we dive in, we will discuss how these approaches are reshaping numerous fields through a solid foundation of fundamental principles.
Research Overview
Summary of Key Findings
Research in ab initio techniques reveals significant insights across various domains:
- In computational chemistry, ab initio methods produce accurate molecular structures and reaction pathways, significantly impacting drug discovery and materials science.
- In physics, using ab initio approaches allows for predicting properties of new materials, vital for developing next-generation electronic devices.
- In artificial intelligence, these methods enhance machine learning algorithms by providing meticulously derived data sets, facilitating better learning models.
Importance of the Research
Understanding ab initio methods is key in pushing the boundaries of scientific discovery. The relevance spans multiple disciplines:
- They encourage new discoveries by offering detailed examinations of systems that are tough to study experimentally.
- By employing these methods, researchers can develop predictive models that lead to practical applications, boosting innovation and efficiency in technology development.
Methodology
Study Design
The exploration of ab initio methods follows a structured analysis of both the theoretical and practical frameworks. This involves:
- Detailed evaluations of existing literature that discusses various mathematical models and computational techniques.
- Case studies showcasing successful implementations of these methods across different fields.
Data Collection Techniques
Data collection relies predominantly on:
- Reviewing peer-reviewed publications, which provide insights into recent advancements in ab initio methodologies.
- Analyzing real-world case studies where ab initio approaches have been successfully applied, demonstrating practical impacts and innovations.
"The power of ab initio techniques lies in their ability to forecast outcomes based on known principles, transforming theoretical predictions into tangible advancements."
In summary, this collection of methods not only provides a strong basis for scientific inquiry but also heralds advancements in research. Productive applications of these techniques can lead to significant breakthroughs across various domains.
Understanding Ab Initio
The concept of ab initio serves as a cornerstone in various scientific fields, encompassing the principles of theoretical understanding and predictive modeling. Its significance lies in the ability to derive conclusions from first principles rather than relying on empirical data. This principle is essential in disciplines like computational chemistry and physics, where complex interactions within systems are analyzed. By embracing ab initio methods, researchers can gain deeper insights, foster innovation, and develop reliable technologies.
Definition and Origin
Ab initio is a Latin phrase that translates to "from the beginning." In scientific contexts, it refers to methods that are rooted in fundamental theories, such as quantum mechanics. Rather than basing conclusions on existing data, ab initio approaches build their conclusions directly from the underlying physical laws and principles. This makes them distinct from empirical methods, which often depend on observation and experience.
The origin of ab initio methods can be traced back to early developments in theoretical physics and chemistry, where initial efforts aimed at understanding molecular and atomic behaviors led to the establishment of quantum mechanics. As researchers aimed to solve complex systems, they realized the potential of analyzing them from fundamental principles. This shift has catalyzed advancements in modeling and simulations, allowing scientists to predict molecular structures and behaviors with greater accuracy.
Philosophical Perspectives
From a philosophical standpoint, ab initio approaches challenge some conventional views in science. Traditional empirical methods rely heavily on observation and data accumulation, while ab initio emphasizes the theoretical underpinnings of phenomena. This raises important questions about the nature of knowledge itself: Can understanding be achieved without direct observation?
Ab initio models advocate for a more fundamental understanding of systems, leading to the notion that theories must be grounded in well-defined principles. These philosophical undercurrents emphasize rationality and logic, which can enhance the robustness of scientific reasoning.
Moreover, considering ab initio from a philosophical angle introduces discussions about knowledge creation. It suggests that deeper insights about the universe are attainable without solely depending on past experiences. This perspective can inspire further inquiry into the nature of reality and our engagements with it.
Historical Context
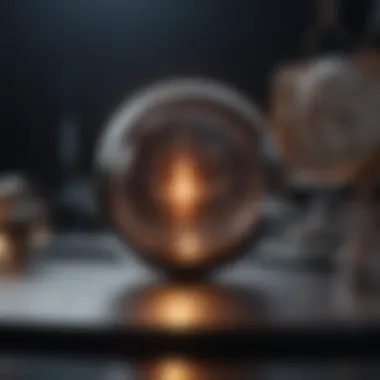
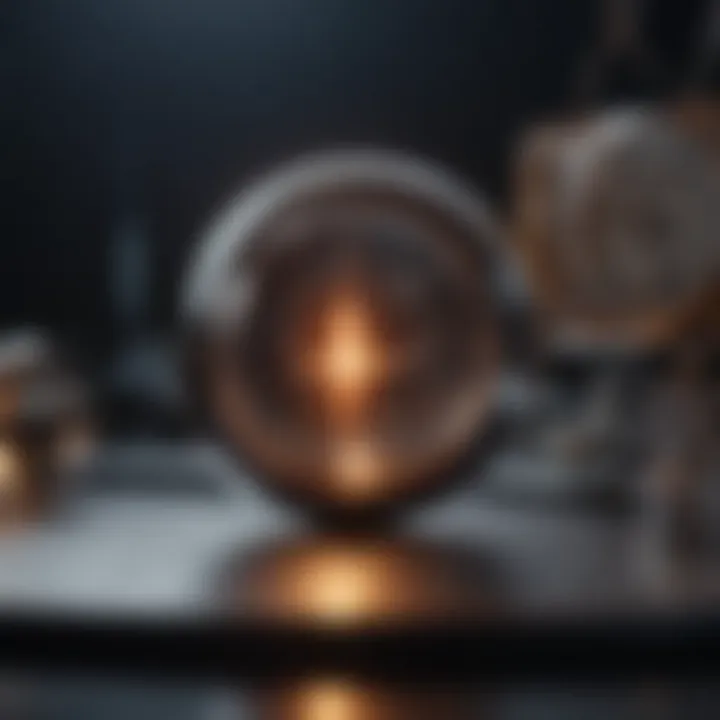
The historical evolution of ab initio methods begins in the early 20th century with the formulation of quantum mechanics. Pioneers such as Max Planck and Niels Bohr laid the groundwork for understanding atomic behavior, which shaped the use of theoretical models. By the mid-20th century, researchers like Walter Heitler and Fritz London pioneered the development of molecular quantum mechanics, illustrating how ab initio methods could predict chemical bonding.
As computing technology advanced, the application of ab initio methods expanded significantly. The introduction of algorithms and software capable of handling complex calculations allowed for the exploration of larger systems with unprecedented detail. Institutions like Gaussian, Inc. advanced the field even more by developing software that enabled widespread access to computational methods in chemistry.
Thus, ab initio has a rich legacy intertwined with scientific progress, as it continues to evolve with advancements in technology. As we stand at the intersection of theory and computation, the historical context helps us appreciate the role that ab initio approaches play in shaping contemporary scientific discourse.
Ab Initio in Computational Chemistry
Ab initio methods in computational chemistry hold a pivotal role in simulating and understanding molecular systems. These techniques are grounded in quantum mechanics and allow scientists to predict molecular properties and behaviors from fundamental principles without empirical data. This approach provides clear advantages over empirical methods, enabling researchers to explore chemical phenomena in a more comprehensive manner. By employing ab initio methods, chemists can obtain high-accuracy results that are essential for advancing various fields such as drug discovery, materials science, and nanotechnology.
Methodological Approaches
Ab initio methods vary widely in their theoretical frameworks. These include Hartree-Fock theory, Density Functional Theory (DFT), and post-Hartree-Fock methods like Configuration Interaction (CI) and Coupled Cluster (CC). Each of these methodologies has its unique merits and is suited for different types of problems.
- Hartree-Fock Method: This is one of the earliest approximations to solve the Schrödinger equation. It simplifies the many-body problem by considering single-electron wave functions and using mean field theory to estimate the electron correlation.
- Density Functional Theory (DFT): DFT has gained popularity due to its balance between efficiency and accuracy. It focuses on electron density rather than wave functions and provides a feasible way to calculate properties of systems with many particles.
- Post-Hartree-Fock Methods: When higher accuracy is required, methods like Configuration Interaction and Coupled Cluster are employed. These approaches take into account electron correlations more effectively, thus improving the predictive capability for chemical properties.
Each of these methodologies draws on complex mathematical frameworks, and choosing the right approach depends on the specific requirements of the molecular system under study.
Role in Molecular Modeling
Molecular modeling is another critical application of ab initio methods in chemistry. Through these techniques, researchers can construct models that replicate the geometries, energies, and behaviors of molecular systems. This is evident in areas such as drug design, where understanding the interactions between a drug molecule and its biological targets is crucial for effective therapy.
Key Benefits of Ab Initio Molecular Modeling:
- Accuracy: Provides high-precision results grounded in physical laws.
- Predictive Power: Enables forecasts of molecular behavior in untested scenarios.
- Insights into Reaction Mechanisms: Offers detailed views of transition states and energy barriers, enhancing the understanding of chemical reactions.
This modeling is often complemented with visualization tools that allow researchers to present complex interactions in an understandable form, thus facilitating increased insight into molecular functions.
Case Studies of Successful Applications
Analyzing practical applications of ab initio techniques can illuminate their importance in contemporary research. For instance, ab initio calculations played a critical role in the development of new materials with tailored properties. In nanotechnology, researchers have predicted the behavior of nanoparticles under various environmental conditions through these methods.
Another notable application is in pharmacology, where ab initio methods have been used to optimize drug candidates by predicting their binding affinities with target proteins. Studies have shown that molecules designed through these techniques lead to effective pharmaceutical agents with fewer side effects. Overall, the use of ab initio methods in computational chemistry significantly enhances both theoretical understanding and practical application across multiple domains.
Ab Initio Techniques in Physics
Ab initio techniques in physics play a crucial role in understanding fundamental interactions and behaviors at the atomic and molecular levels. This approach relies on first principles, granting insights that empirical methods may overlook. By utilizing the principles of quantum mechanics, researchers can predict physical properties without the need for experimental data. This capability is especially important in fields where experimental methods are challenging or impossible.
Quantum Mechanical Foundations
The cornerstone of ab initio techniques in physics is rooted in quantum mechanics. By applying the Schrödinger equation, these methods model systems based on their electronic structure. The calculation starts with an approximation of the wave function, which describes the quantum state of a system. These techniques often include methods like Hartree-Fock and density functional theory (DFT), each providing a different level of accuracy and computational demand.
- Hartree-Fock: This method simplifies the interaction between electrons, allowing for a basic but comprehensive understanding of electron correlation.
- Density Functional Theory (DFT): It is widely used for more complex systems and focuses on the electron density rather than the wave function. This shift allows for efficient computations in larger systems while still capturing essential electron interactions.
Understanding the quantum mechanical foundations equips physicists to explore material behaviors and chemical properties more accurately.
Applications in Material Science
Ab initio techniques significantly advance material science, especially in the development and analysis of new materials. These techniques help in tailoring material properties for specific applications through precise calculations of electronic structures and interactions.
Some notable applications include:
- Predicting new materials: Ab initio methods assist in discovering materials with unique properties, contributing to advancements in nanotechnology and superconductors.
- Analyzing defects in materials: Techniques can reveal how defects alter material properties, informing engineering decisions for improved durability and functionality.
- Understanding phase transitions: By studying how materials change state under varying conditions, researchers can develop more efficient materials for energy conversion and storage.
Challenges and Limitations
Despite their power, ab initio techniques face several challenges and limitations.
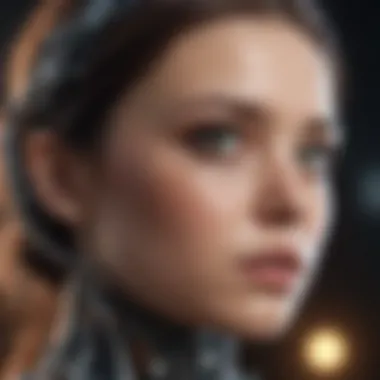
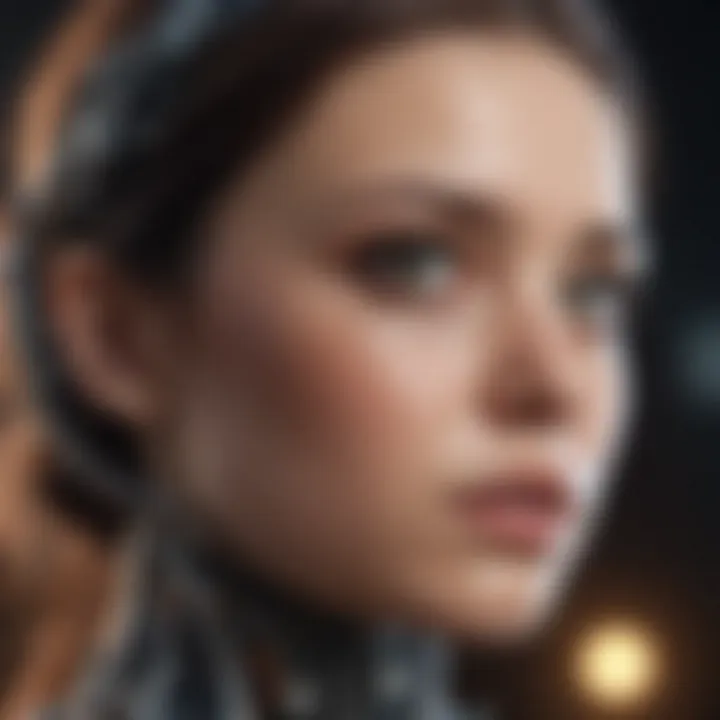
- Computational Cost: The complexity of quantum mechanical calculations often results in high computational demands. Larger systems can be prohibitively expensive in terms of time and resources to calculate.
- Accuracy vs. Efficiency: There is often a trade-off between the accuracy of the results and the computational efficiency.
- Model Limitations: Some methods may not fully capture electron correlation, which can lead to inaccuracies in predictions.
Despite these challenges, ongoing advancements in computational technology and algorithms continue to enhance the applicability of ab initio methods in physics, paving the way for future discoveries.
Ab Initio in Artificial Intelligence
The integration of ab initio methods into artificial intelligence signifies a profound shift in how we approach model building and data interpretation. These principles are crucial as they provide a framework for creating AI systems that are not merely reliant on empirical data but are instead grounded in fundamental truths and principles of their respective domains. Ab initio approaches enable researchers to formulate algorithms that simulate real-world scenarios from a foundational level, resulting in more accurate and reliable models. This section discusses the principles of ab initio AI models, their impact on machine learning algorithms, and the future prospects for innovations.
Principles of Ab Initio AI Models
Ab initio AI models are constructed upon first principles, aiming to understand and replicate the basic mechanisms of the system being studied. Such models often incorporate essential theories and scientific laws relevant to the specific application. For instance, a model designed for predicting protein structures would base its algorithms on the laws of chemistry and physics governing molecular interactions. By doing so, it bypasses the potential pitfalls of data overfitting and model bias that can occur when merely relying on training data alone.
Key principles include:
- Fundamental Theories: Relying on established theories rather than existing datasets.
- Predictive Power: Utilizing core scientific knowledge to drive predictions and insights.
- Interdisciplinary Collaboration: Engaging experts from various fields to enhance model accuracy and relevance.
Each of these principles serves to reinforce the credibility and applicability of AI solutions across diverse fields such as healthcare, finance, and material science.
Impacts on Machine Learning Algorithms
The influence of ab initio principles on machine learning can be both profound and multifaceted. By embedding fundamental knowledge into the design of algorithms, researchers create systems that can generalize better across unseen data, reducing the chances of overfitting that purely empirical training might face.
The following impacts are noteworthy:
- Improved Accuracy: Models built on solid foundations tend to yield higher predictive accuracy.
- Reduced Dependency on Large Datasets: Ab initio models can function effectively with smaller datasets, which is particularly beneficial in domains where data collection is costly or impractical.
- Enhanced Interpretability: The grounding in fundamental principles often makes the models’ decision-making processes easier to understand, thus building trust with users and stakeholders.
By focusing on these elements, ab initio models can lead to more enlightened applications in areas such as predictive analytics and complex system simulations.
Future Prospects and Innovations
Looking to the future, the marriage of ab initio methods and artificial intelligence is likely to yield significant innovations. As computational capabilities improve and the integration of AI systems becomes deeper, new horizons are expected to open. Potential advancements include:
- Real-time Predictive Models: AI systems that can adjust in real-time based on new inputs, guided by first principles.
- Cross-Disciplinary Applications: Ab initio AI models could be used in environmental science to simulate climate changes, employing fundamental laws of physics and chemistry.
- Ethical AI Development: Ensuring that AI models are built upon robust principles may foster greater ethical considerations and accountability in AI deployments.
Comparative Analysis with Empirical Methods
Comparative analysis between ab initio methods and empirical methods is crucial in understanding the strengths and limitations of both approaches. Ab initio techniques are grounded in fundamental physical laws, while empirical methods rely on experimental data and heuristics. This section discusses these fundamental differences, evaluates strengths and weaknesses, and explores integrated approaches.
Fundamental Differences
Ab initio methods are built from the ground up using quantum mechanics. They do not depend on experimental data, making them universally applicable to various systems. This contrasts with empirical methods that often require specific data to yield results. For instance, empirical calculations may be tailored to particular materials or conditions, while ab initio calculates outcomes based directly on particle interactions and fundamental principles.
A notable example is the application of Hartree-Fock methods in quantum chemistry, where calculations derive directly from many-body wavefunctions. In contrast, molecular dynamics might use effective potentials determined empirically from experiments. The reliance on fundamental laws in ab initio methods often results in higher computational costs but promises accuracy and broad applicability.
Strengths and Weaknesses
Each method holds distinct advantages and drawbacks.
Strengths of Ab Initio Methods:
- Accuracy: Results are grounded in theory, yielding reliable predictions for a broad range of systems.
- Universality: Applicable across multiple scientific domains, enhancing interdisciplinary research.
- Predictive Power: Can model new systems without prior experimental data.
Weaknesses of Ab Initio Methods:
- Computational Intensity: High resource requirements can limit scalability for complex systems.
- Time Consumption: Long runtimes for calculations may hinder real-time applications.
Strengths of Empirical Methods:
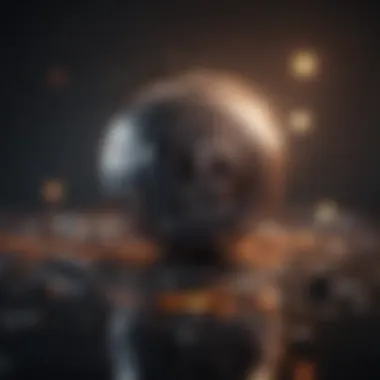
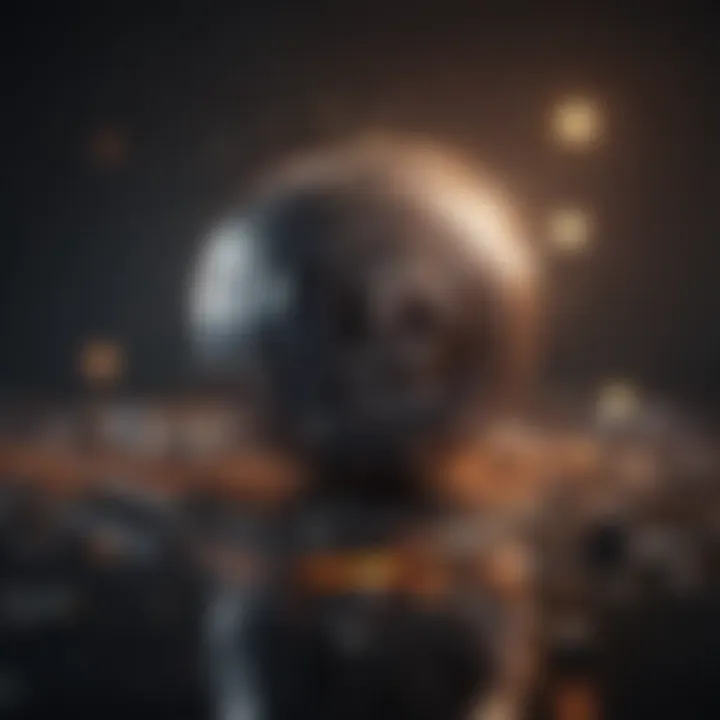
- Speed: Generally faster computations enable quick exploration.
- Resource Efficiency: Lower computational resources make them accessible for many research contexts.
Weaknesses of Empirical Methods:
- Limited Applicability: Dependence on existing data limits use in novel systems.
- Potential for Bias: Results may reflect assumptions and simplifications that could mislead interpretations.
Integrated Approaches
Integrated approaches combine the strengths of both ab initio and empirical methods. By leveraging empirical data to fine-tune ab initio results, researchers can achieve faster computations without sacrificing accuracy. This hybrid methodology enables predictive modeling while capitalizing on existing data frameworks.
For example, in materials science, researchers may utilize ab initio calculations to develop initial models, followed by empirical methods for refinement. This synergy not only enhances precision but also expedites research in practical applications.
An integrated approach allows scientists to navigate complex systems efficiently, balancing the detailed insight obtained from ab initio methods with the speed of empirical assessments.
Implications of Ab Initio Research
The examination of ab initio research carries significant implications across various fields. This approach demands a foundational understanding of systems and processes, aiming to predict outcomes from first principles without empirical data. Such an orientation not only enhances theoretical frameworks but also provides practical benefits.
By anchoring findings in fundamental scientific principles, ab initio research fosters a comprehensive interpretation of phenomena. Researchers can develop models and simulations that lead to a deeper grasp of complex systems. Additionally, ab initio methods can illuminate areas where empirical approaches might fall short.
Impact on Scientific Understanding
Ab initio approaches profoundly affect scientific understanding by prioritizing foundational elements. Because these methods derive from established laws of physics and chemistry, they yield insights that extend beyond mere correlation. The equations of motion or interaction potentials are based on theoretical constructs rather than observational data alone.
- Predictive Power: These methods can provide high levels of accuracy in predicting molecular behavior, which is essential in areas like drug formulation.
- Unified Theories: They promote the development of unified theories across disciplines since they rest on common principles.
- Validation of Empirical Data: Ab initio research often validates or refines empirical data, offering a benchmark for experimental results.
Ab initio studies encourage a shift from empirical validation to theoretical grounding, enriching the scientific discourse.
Technological Advancements
The advancements facilitated by ab initio research are noteworthy. Industries ranging from pharmaceuticals to materials science increasingly rely on ab initio methods to engineer innovative products and processes. The capability of these approaches to yield precise predictions can enhance design procedures and optimize performance, leading to more efficient technologies.
- Drug Design: With the power to simulate molecular interactions, many drug discovery processes have transitioned to ab initio methods, significantly speeding up the time to market.
- Material Innovation: The discovery and design of new materials, including nanomaterials and superconductors, owe much to ab initio calculations.
- Computational Tools: Software such as Gaussian and VASP incorporates advanced ab initio methods, creating user-friendly interfaces for practitioners.
The impact of ab initio techniques on technological evolution can hardly be overstated. By harnessing the foundational knowledge of systems, industries push boundaries in innovation.
Ethical Considerations
While the implications of ab initio research are largely positive, ethical considerations necessitate careful examination. The predictive capabilities come with responsibilities and potential consequences. As researchers embrace ab initio methods, they must consider the ethical ramifications of their applications.
- Responsible Use of Technology: The use of computational predictions should align with ethical standards to prevent misuse in fields like biotechnology or defense.
- Transparency: Researchers must maintain transparency in their methodologies and findings, ensuring reproducibility and access to information.
- Environmental Impact: The design of new materials should consider sustainability and the long-term environmental implications.
Consequently, fostering an ethical framework around the use of ab initio research remains crucial. By prioritizing integrity, scientists can mitigate risks while maximizing societal benefits.
"The virtues of ab initio methods go hand in hand with responsibilities that must guide their application in modern research."
End
The conclusion serves as an essential component of this article, drawing together the various threads discussed throughout. It encapsulates key findings and emphasizes the significance of ab initio methods across diverse scientific fields. By reflecting on how these foundational approaches contribute to our understanding of complex systems, the conclusion underscores the value of continued exploration in this domain.
Summary of Key Insights
In reviewing the insights gained from this article, several core themes emerge:
- Foundational Principles: Ab initio methods, grounded in principles of quantum mechanics, provide a robust framework for exploring various phenomena in chemistry and physics.
- Diverse Applications: The versatility of ab initio techniques extends to fields such as artificial intelligence and materials science, showcasing their role in enhancing predictive accuracy and innovation.
- Refinement of Scientific Approaches: Through integrating ab initio with empirical methods, researchers can achieve a more holistic understanding of systems and generate more meaningful results.
- Ethical and Technological Considerations: As the implications of ab initio research unfold, it is crucial to address ethical considerations alongside the technological advancements this research spearheads.
These insights collectively illuminate the profound impact of ab initio methodologies in contemporary scientific inquiry.
Future Directions for Research
Looking ahead, several paths for future research in ab initio methods become apparent:
- Enhanced Computational Methods: Continued development of algorithms and computational techniques is essential for enabling ab initio approaches to tackle larger and more complex systems.
- Interdisciplinary Applications: Exploring the interplay between ab initio methods and other disciplines, such as biology and neuroscience, may yield novel insights and applications.
- Autonomous Research Systems: The integration of ab initio methodologies within machine learning frameworks could lead to more automated and efficient research processes.
- Addressing Limitations: As the field progresses, actively addressing the current limitations of ab initio methods will promote their efficacy and broaden their applicability.
By pursuing these directions, researchers can unlock new potentials within the realm of ab initio methods, furthering our understanding of the intricate systems that govern natural phenomena.