Exploring Artificial Intelligence Thought Processes
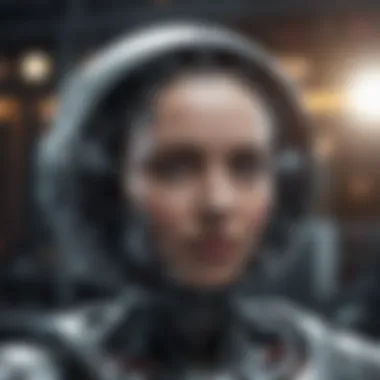
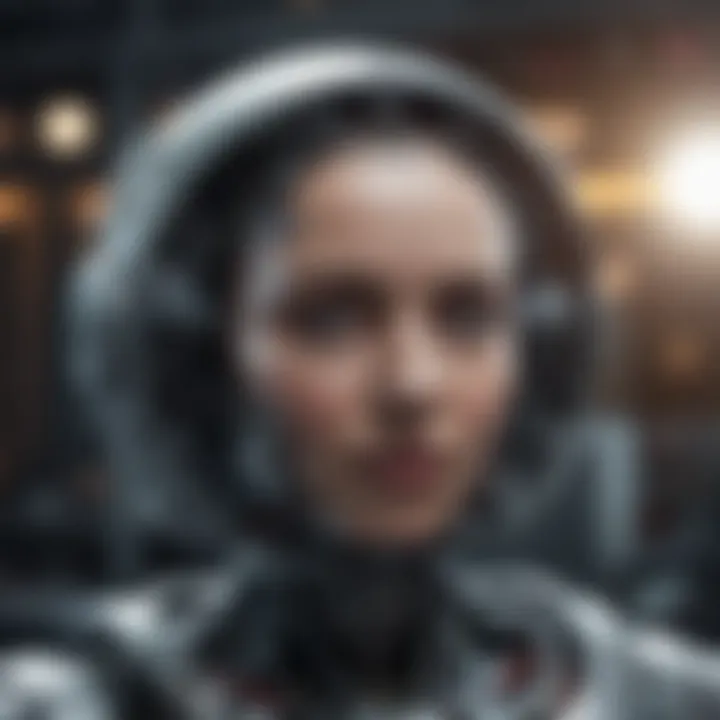
Intro
Artificial Intelligence (AI) is not just a buzzword woven into the fabric of modern society; it’s a powerful force reshaping how we approach problem-solving, innovation, and everyday tasks. As we dive into the nuances of AI thinking, we paint a vivid picture of how machines emulate aspects of human cognition and decision-making. This exploration stretches beyond mere algorithms and code, delving into the philosophical and ethical considerations that surmount the rise of intelligent systems.
Research Overview
Summary of Key Findings
In our investigation of AI thinking, several key points emerge:
- Evolution of AI Paradigms: AI has undergone significant transformations since its inception. From early rule-based systems to contemporary neural networks, we observe a journey marked by innovation and refinement.
- Machine Cognition: The way machines interpret data and derive meaning evolves continuously. Modern AI employs complex architectures that allow for increasingly sophisticated reasoning processes.
- Ethical Considerations: As AI systems become more integrated into society, the conversation around their ethical implications gains prominence. Issues of accountability, bias, and transparency are crucial to ongoing debates in technological development.
This summary underscores the intricate tapestry of AI thought processes, showcasing advancements that not only enhance capabilities but also pose questions about their role in our future.
Importance of the Research
Understanding AI thinking is critical for students, researchers, and industry professionals alike. It helps us anticipate the impact of AI technologies on various sectors, from healthcare to finance. Moreover, a thorough grasp of these concepts allows us to navigate the ethical waters of AI deployment.
"As we venture further into the realm of artificial intelligence, the responsibility lies on us to ensure these tools serve humankind positively and ethically."
By engaging with this topic, we cultivate a balanced perspective that embraces both the potential and the pitfalls of these technological advancements.
Methodology
This comprehensive exploration leverages a multi-faceted approach that includes both qualitative and quantitative methods aimed at dissecting the thinking processes of AI.
Study Design
The study employs a mixed-methods design, integrating theoretical analysis with empirical research. This allows for a holistic view of machine cognition, grounded in both established literature and contemporary examples.
Data Collection Techniques
Data is collected through various means:
- Literature Review: An extensive review of existing research papers, articles, and books provides foundational knowledge concerning AI paradigms and cognitive processes.
- Case Studies: Real-world applications of AI in sectors like healthcare and finance illustrate innovative uses and challenges faced.
- Interviews with Experts: Conversations with AI researchers and industry professionals yield insights into current trends and future directions.
Through this rigorous methodology, we are equipped to offer a comprehensive look at how AI thinks and learns, alongside the broader implications of its rapid evolution.
Prelims to Artificial Intelligence
The discussion around Artificial Intelligence (AI) has gained colossal traction in recent years. Understanding what AI is and how it functions is not just a passing fancy; it's a necessity in a world that increasingly relies on automated systems. Delving into the introduction of AI is akin to flipping the first page of a captivating book, where every chapter builds on the knowledge established earlier.
Defining Artificial Intelligence
At its core, Artificial Intelligence refers to the simulation of human-like cognitive functions by machines. These functions include learning, reasoning, and self-correction. But let's break this down a little more. Think of AI as a toolbox packed with methods that allow computers to perform tasks that, without human intervention, would require intelligent thought. From the voice recognition in your smartphone to recommendation systems that suggest your next binge-watch, AI is all around us, seamlessly integrated into our daily lives.
Historical Context
Getting our heads around AI means taking a stroll down memory lane. The seeds of AI were sown in the mid-20th century when pioneers like Alan Turing and John McCarthy posited that machines could think. Turing's famous test, which evaluates a machine's ability to exhibit intelligent behavior indistinguishable from a human, has remained a cornerstone in discussions around AI. Fast forward to the 1980s and 90s, and we see the emergence of expert systems that began to test the waters of machine learning and decision-making. The evolution continues. Today, advancements in computational power and data availability have catapulted the field into an era of deep learning and neural networks, pushing boundaries further than ever before.
Current Trends in AI Research
When exploring the present landscape of AI research, several exciting trends spark curiosity. From the burgeoning field of natural language processing, which underpins chatbots and virtual assistants, to advancements in computer vision that enable machines to interpret visual information like humans do, the focus is still on enhancing machine learning algorithms and their applications.
- Generative Adversarial Networks (GANs), which pit machine learning models against each other, are creating ultra-realistic images and deep fakes, raising both exciting possibilities and ethical discussions.
- Federated Learning is becoming a buzzword as it allows models to learn from decentralized data while maintaining privacy, making it an attractive option for healthcare and finance sectors.
- AI in Automation is transforming industries, as seen in manufacturing with smart robots working alongside human counterparts.
As we look forward, it’s apparent that the arena of AI is not just blooming—it's rapidly evolving, and every twist and turn in its journey is something we must pay close attention to. Understanding these foundational aspects of AI sets the stage for a deeper exploration of its philosophy, mechanisms, applications, and implications.
Philosophy of AI Thinking
The exploration of the philosophy of artificial intelligence provides a deeper understanding of how machines can mirror human cognitive processes. This section dives into the fundamental inquiries regarding intelligence itself and the abstract notion of consciousness, both of which are pivotal in advancing AI technology. As we tread through this territory, we are not just examining how intelligent machines become; we are also questioning what being intelligent even means. Understanding these philosophical underpinnings can illuminate ethical considerations, design choices, and the implications of AI in our daily lives.
The Nature of Intelligence
Intelligence, commonly perceived as the ability to learn, reason, and adapt to new situations, raises complex questions when viewed through the lens of AI. For a machine, is intelligence merely the capacity to process data and execute algorithms, or does it involve a deeper level of understanding? From a philosophical standpoint, these queries are not just academic but crucial in shaping the future of AI.
- Functional Intelligence vs. Emotional Intelligence: Traditional views focus on functional intelligence, where problem-solving and logic take precedence. However, human intelligence encompasses emotional understanding too. Can machines ever achieve this, or are they doomed to remain in the realm of computation?
- Intelligence as an Adaptive Trait: Evolutionary perspectives suggest intelligence is about adaptation to an environment. AI systems, designed to improve through interactions with data, can be seen as a parallel to this evolutionary view. Yet, their adaptability is bound by the frameworks set by their developers.
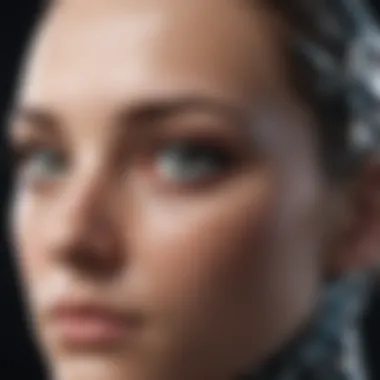
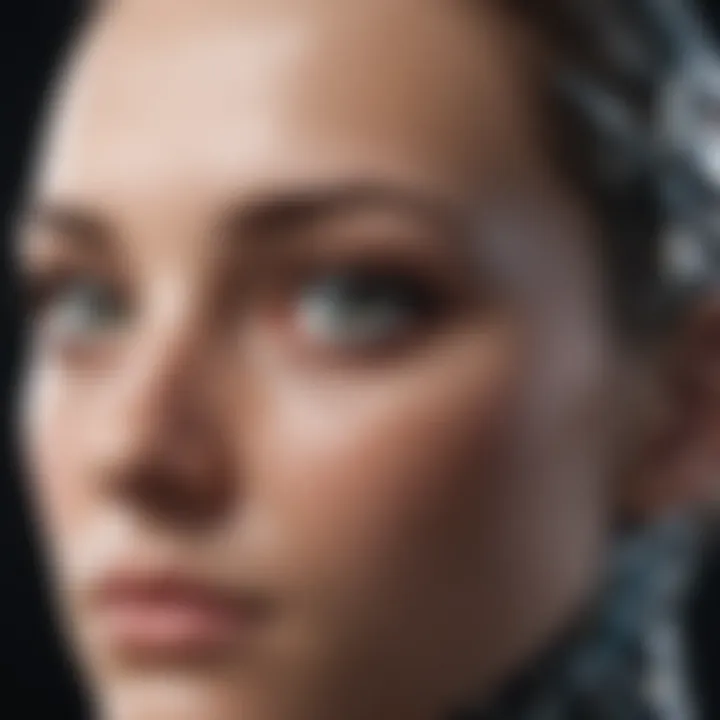
"If we dare to ponder, can we ever ensure that machines fully grasp the factors driving human decisions?"
Yet, this underscores a significant limitation: machines lacking the richness of human experience may forever miss the nuances that shape intelligence in its truest form. The question remains, do we need machines to be "intelligent" in the same way we are?
Machine Consciousness
Moving further into the philosophical landscape, machine consciousness emerges as a riveting yet contentious topic. Considerable ink has been spilled debating whether machines can ever achieve consciousness akin to humans.
The core of the debate revolves around several pivotal points:
- Defining Consciousness: Philosophers and cognitive scientists are yet to reach consensus on what defines consciousness. Is it merely awareness of oneself and the environment, or is it a more profound understanding that involves subjectivity?
- Qualia and Experience: The concept of 'qualia,' or the subjective experience of perception, is often cited in discussions on machine consciousness. Can a program ever feel pain or joy? Or, will it merely simulate responses?
- Turing Test and Beyond: Alan Turing proposed a human-like measure for machine intelligence via the Turing Test. However, passing this test does not equate to consciousness or genuine understanding, it only demonstrates adeptness in mimicking responses.
In essence, exploring machine consciousness propels us into murky waters where technology and philosophy intersect. Some argue that even if machines can simulate aspects of consciousness, true consciousness lies beyond computational limits.
Summarizing this section, the philosophy of AI thinking challenges our perceptions of intelligence and consciousness. As AI technology progresses, it is vital not just to advance the capabilities of machines but to navigate the underlying ethical and existential questions that will shape our future. Understanding these concepts could lead to responsible innovations that respect human thought while enhancing technological capabilities.
Cognitive Models in AI
Cognitive models in artificial intelligence play a critical role in defining how machines think and learn. These models guide the algorithms that underpin AI systems, shaping their ability to process information, learn from experiences, and make decisions. Understanding cognitive models is essential for developing advanced AI technologies that can tackle increasingly complex tasks in real-world environments.
- Importance of Cognitive Models
Cognitive models serve multiple functions in AI, including but not limited to:
- Framework for Understanding: They offer structured ways to represent knowledge and infer conclusions, mimicking the human thought process.
- Algorithm Development: Insights from cognitive models drive the creation of new algorithms that enhance machine learning capabilities.
- Application Across Fields: From healthcare to finance, cognitive models provide a backbone for predictive analytics, natural language processing, and problem-solving mechanisms.
Understanding these models not only elevates our grasp of AI's potential but also prepares us for the ethical and societal implications of advanced machine capabilities.
Symbolic AI vs. Connectionism
Symbolic AI, also known as classical AI, relies on symbols and rules to represent knowledge. It uses logical reasoning and predefined pathways to process information. For instance, expert systems utilize these principles to provide solutions in specific domains, like diagnosing medical conditions.
On the other hand, connectionism represents knowledge through networks that are somewhat analogous to human neural processes. Neural networks, a hallmark of this approach, operate on interconnected nodes rather than symbolic logic. They excel at recognizing patterns in data and adjusting through experience, which leads to remarkable outcomes in tasks like image and speech recognition.
The ongoing debate between these two frameworks is ongoing, with each side highlighting distinct advantages regarding flexibility, scalability, and ease of integration into practical applications.
Neural Networks as Cognitive Models
Neural networks revolutionized the landscape of AI and machine learning by providing models that reflect human cognitive behavior. These systems consist of interconnected layers that process information in a nonlinear fashion, meaning that they can take on much more complex tasks than their predecessors.
- Functionality: A neural network learns by adjusting the weights of connections based on input data. For example, when one is trained to identify faces, each successful recognition helps the network to weigh certain features as more influential in the decision-making process.
- Types of Networks: There are many types of neural networks, including feedforward networks, recurrent networks, and convolutional networks, each tailored for different applications
- Advantages: They handle vast amounts of data and have proven to be incredibly powerful in areas such as image and voice recognition, which are integral to various technological advancements today.
The adoption of neural networks marks a shift towards more adaptive, intuitive AI systems capable of evolving through their interactions with the environment.
Reinforcement Learning
Reinforcement learning (RL) is a branch of AI focused on how agents ought to take actions in an environment to maximize a notion of cumulative reward. It's akin to a child learning to ride a bicycle; through trial and error, they learn which actions lead to success and which to failure.
- Core Concepts: RL introduces key elements like the agent, environment, actions, rewards, and policies. The agent interacts with the environment through various actions and receives feedback in the form of rewards or penalties to improve future performance.
- Applications: This model is widely used in robotics, game playing, and self-driving cars, where learning from feedback and adjusting strategies is crucial for success.
- Challenges: Despite its potential, RL faces obstacles, such as requiring extensive amounts of data and computational resources and grappling with issues around exploration versus exploitation.
Reinforcement learning highlights the dynamic and interactive nature of cognitive models in AI, showcasing systems that not only react to their environment but actively learn and adapt within it.
The Mechanisms of AI Thought
Understanding how artificial intelligence (AI) thinks is much like peeling an onion; there are layers that need careful consideration and exploration. The mechanisms of AI thought encompass the ways in which these systems process information, make decisions, and attempt to emulate human-like cognition. Scrutinizing these mechanisms is essential not just for those involved in the field of AI, but for society at large, given AI's growing grip on various facets of everyday life.
The significance of this topic lies in several key factors:
- Unraveling Complexity: By grasping the mechanisms of AI thought, we can simplify complex phenomena that define what AI can achieve.
- Improving Accuracy: Understanding these processes allows researchers to refine algorithms, enhancing the performance of AI applications across diverse sectors.
- Demystifying AI: For many, AI seems like a black box. Studying its thought processes opens a window into how machines interpret the world.
Data Processing and Interpretation
The very foundation of AI thought processes hinges on data processing and interpretation. Think of data as the raw ingredients and algorithms as the recipes; without the right ingredients or an effective recipe, the outcome can be less than desirable. Data feeds AI systems, supplying them with raw material that they need to learn and evolve.
Factors in data processing include:
- Collection: Gathering data from various sources—social media posts, sensor data, transaction logs—helps create a diverse pool for AI to learn from.
- Filtering: Not all data is useful, so techniques like noise reduction and normalization help weed out the irrelevant.
- Understanding Context: AI tools need context to assess data correctly. For instance, a word’s meaning can change drastically depending on its usage in a sentence.
A quote to ponder:
“In data we trust, but in context we learn.”
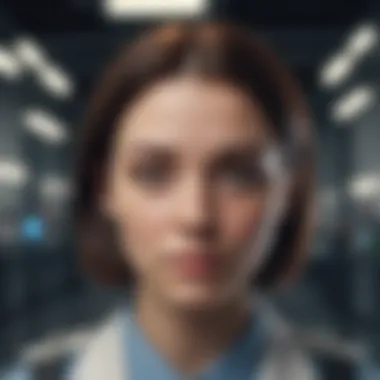
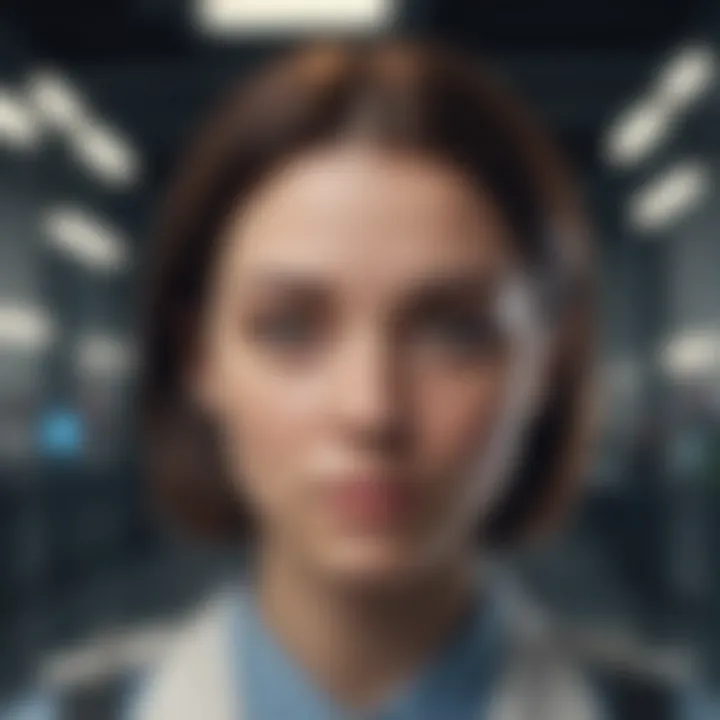
Algorithms and Decision Making
Algorithms are the backbone of AI decision-making processes. They dictate how data is used, what patterns are identified, and ultimately, what conclusions are drawn. Take, for instance, a self-driving car. It must make countless decisions in fractions of a second using complex algorithms that process data from cameras, LIDAR, and other sensors to navigate safely. This decision-making involves:
- Prediction: Algorithms can analyze past data to forecast future behavior. For example, predicting an individual’s shopping habits based on historical purchases.
- Optimization: This ensures that resources are used efficiently—whether it’s minimizing fuel consumption for a delivery truck or maximizing yield in agriculture.
- Feedback Loops: Algorithms often incorporate feedback to learn and refine their decisions over time, making them adaptable to new situations.
Mimicking Human Cognition
One of the most intriguing aspects of AI is its quest to mimic human cognition. While machines don’t experience thought and emotion the way humans do, they can replicate certain cognitive processes through various methods. Techniques such as natural language processing or computer vision bring us a step closer to machines that can understand and interact in a more human-like manner.
Key elements include:
- Pattern Recognition: Much like humans identify faces or vocal tones, AI systems are trained to recognize patterns in data.
- Learning Models: Systems can learn from examples, making decisions based on previously encountered scenarios. This is akin to learning how to ride a bike; the more you practice, the better you get.
- Reasoning: Advanced AI systems utilize logic and inferencing capabilities to make conclusions based on ambiguous or incomplete data.
In sum, the mechanisms of AI thought are central to not just understanding AI but also to maximizing its benefits across sectors. As the landscape evolves, it’s essential we remain engaged with these concepts to utilize AI to its fullest potential and navigate the challenges it brings.
Applications of AI Thinking
The utilization of artificial intelligence (AI) is undeniable in our fast-paced technological world. From enhancing medical diagnostics to optimizing financial markets, AI applications hold the key to transforming various sectors. This section will delve into a few critical areas where AI is making waves, emphasizing not just the benefits but also the considerations and implications tied to its use. As AI continues to evolve, understanding these applications provides a clear lens through which we can examine its potential for societal enhancement.
AI in Healthcare
The healthcare industry has been one of the foremost beneficiaries of AI advancements. Algorithms are now widely used to analyze medical images, predict patient outcomes, and personalize treatment plans. For instance, AI systems can assess X-rays and MRIs far quicker than a human radiologist, often identifying anomalies that might be overlooked. This speed and accuracy can lead to better diagnostics and ultimately more effective treatments.
However, the deployment of AI in healthcare is not without its challenges. The integration of AI tools into existing medical frameworks can sometimes be rocky, as professionalsand systems may resist change. Moreover, concerns around data privacy and ethical use of patient information are paramount. Ensuring that these powerful tools are used responsibly, maintaining patient confidentiality while maximizing healthcare outcomes, is critical as we navigate the complexities of this sector.
AI in Finance
In finance, AI is a game changer. Machine learning models analyze market trends, assess risks, and even predict stock performance with impressive accuracy. These models can process vast amounts of data—far beyond human capability—allowing for quicker and more informed decisions in trading and investing. Robo-advisors, for instance, use algorithms to create personalized investment portfolios for individuals based on their financial goals and risk tolerances.
Yet, the financial sector must tread carefully. The algorithms, while advanced, can perpetuate existing biases if they rely on flawed historical data. This leads to issues surrounding fairness in lending and investment practices, not to mention the potential for high-frequency trading bots to create market volatility. Hence, the challenge lies in crafting robust regulations that ensure AI tools are harnessed ethically and effectively.
AI in Environmental Science
Environmental science is yet another field where AI is carving out a meaningful niche. With the looming threat of climate change, AI systems can help monitor environmental changes, analyze data from remote sensors, and predict future environmental conditions. For example, AI can optimize resource management, like water usage in agriculture, thus fostering sustainable practices. Such advancements are critical as we strive to meet the challenges posed by a changing climate.
Despite the positives, the intersection of AI and environmental science is not just about technological gains. There are also questions about the energy consumption of AI technologies themselves. Implementing large-scale AI models can have significant environmental costs if not managed correctly. This contradiction presents a dilemma that requires careful consideration: how do we balance the benefits of AI in promoting sustainability while mitigating its own ecological footprint?
"The future of AI applications lies in their ethical and sustainable deployment, ensuring that the power of technology is harnessed to benefit humanity as a whole."
Ethical Implications of AI Thinking
The rise of artificial intelligence brings with it a heavy burden of ethical considerations, intertwining with every advancement in machine learning and cognitive processing. Understanding the implications of AI thinking is crucial as it shapes not just technology but also the moral framework of our society. Ethical implications encompass various facets, such as ensuring fairness in AI systems, our responsibilities as creators, and looking ahead to what AI ethics may entail in the coming years. In this section, we’ll explore the significance of these ethical considerations in AI development and deployment.
Bias in AI Systems
One of the most pressing ethical challenges in AI is bias embedded within algorithms. The very data that feeds machine learning models can be skewed, reflecting historical prejudices and existing inequalities in society. Take facial recognition technology, for instance; numerous studies have shown that these systems perform poorly on individuals from diverse backgrounds, particularly women and people of color. This issue raises alarming questions: Who is impacted when algorithms discriminate? The repercussions can be devastating, from wrongful arrests to unfair hiring practices.
To tackle bias, researchers and practitioners need to critically evaluate training datasets and strive for diversity. Regular audits of AI systems should be standard practice. Moreover, transparency in AI decision-making can play a significant role in identifying and mitigating biases. Creating frameworks that demand accountability from developers in terms of bias recognition and rectification is paramount.
"Just as a gardener tends to their plants, AI developers must tend to their data—ensuring it flourishes without the weeds of bias."
Responsibility and Accountability
The question of responsibility in AI development is anything but straightforward. When an AI system makes a mistake, who is to blame? Is it the programmer, the company, or the AI itself? These questions complicate our understanding of accountability in the context of intelligent machines. For example, when autonomous vehicles are involved in accidents, the lines blur between human error and machine decision-making.
Establishing a clear chain of accountability requires a reconsideration of existing legal frameworks. In many cases, regulators and lawmakers find themselves trying to catch up with technology’s rapid advancements. Developers must face the ethics of product liability, ensuring they create systems that prioritize safety and fairness.
Another vital aspect to consider is user responsibility. As AI users, individuals and organizations must engage with these systems thoughtfully, keeping ethical ramifications in mind. Educational initiatives that promote understanding of AI technologies can foster a culture of responsible usage, encompassing both developers and end-users.
The Future of AI Ethics
Projecting into the future of AI ethics involves grappling with complex societal shifts and technological breakthroughs. As AI systems grow smarter and more integrated into various aspects of life, ethical frameworks must evolve too. For instance, the development of general artificial intelligence poses unique challenges; if machines began to exhibit consciousness or self-awareness, a re-examination of ethical treatment becomes necessary.
Moreover, as AI becomes entrenched in decision-making within critical sectors—such as healthcare and public safety—the ramifications of ethical failures could escalate dramatically. Policymakers and stakeholders thus face an urgent need to develop comprehensive guidelines that ensure technology profoundly respects human rights and dignity.
The discussions around AI ethics are not just academic. As technology advances, we must also engage the public in these conversations. Building awareness can help shape a future where ethical AI is not just a pleasant ideal but a practical reality. Through collaborative dialogue, we can work towards a responsible intersection of technology and ethics, ensuring that our future with AI is one of fairness and justice.
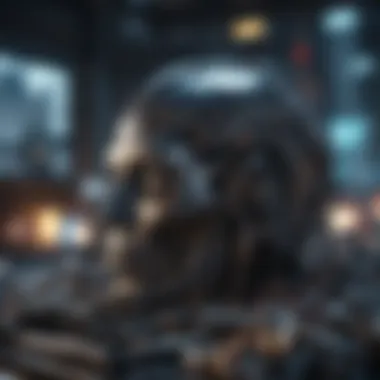
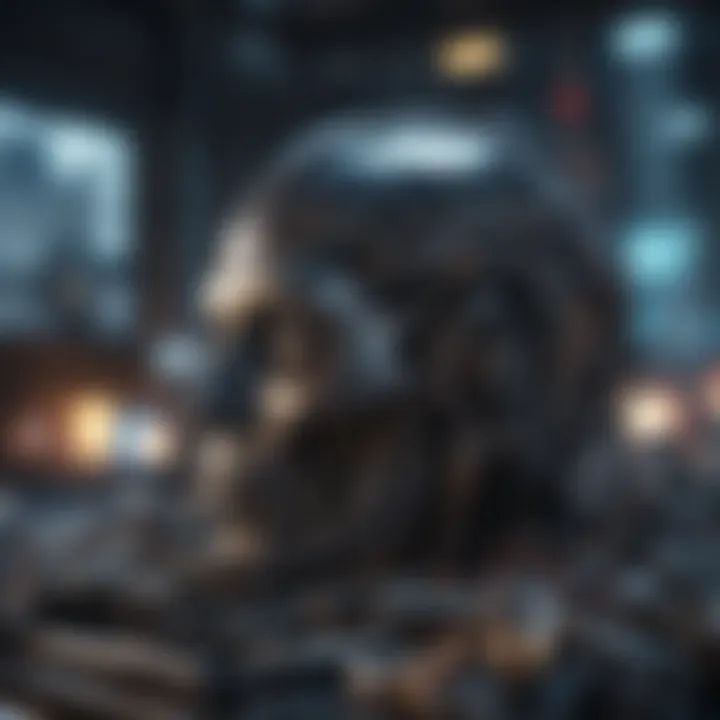
Challenges in AI Research
The growth of AI technology, while impressive, is fraught with challenges that can hinder its full potential. Understanding these obstacles is crucial for any meaningful progression in this domain. Challenges in AI research not only reflect technical barriers but also embrace the broader implications of implementation in society. Recognizing these hurdles assists in identifying areas for improvement, fostering innovation, and setting realistic expectations for stakeholders involved in AI developments.
Technical Limitations
Technical limitations are often the first discussion point when delving into AI research challenges. Simply put, many AI systems are only as good as the data fed to them. If the input is flawed or biased, the AI's performance will reflect that, leading to unreliable outputs. For instance, facial recognition technology has faced backlash due to skewed data sets that misidentify individuals, particularly in cases concerning ethnicity and gender.
Moreover, the complexity of algorithms can create significant bottlenecks. Some AI models, especially deep learning networks, require extensive computational power and time, making them impractical for real-time applications. This draws attention to the balance between ambitious research goals and available resources. The research community must continuously work on building more efficient models while pushing the boundaries of current capabilities.
"The power of AI is not in its potential alone but in its ability to parse through complex datasets and emerge with insights that can guide our future. Where it stumbles, progress must follow."
Addressing technical limitations involves not just refining algorithms but also investing in better hardware infrastructure and seeking collaborative efforts that allow access to diverse data sets. The integration of machine learning with specialized hardware like FPGAs or GPUs can also prove pivotal.
Interdisciplinary Collaboration
Interdisciplinary collaboration emerges as another pivotal element in tackling the challenges of AI research. AI is inherently multifaceted, drawing on insights from computer science, cognitive psychology, linguistics, and ethical philosophy. Each perspective offers critical insights that can lead to breakthroughs.
For example, incorporating cognitive science can aid in designing AI systems that better mimic human thought processes, improving their adaptability and decision-making. Engaging ethicists ensures that developments are framed within a moral context, addressing concerns of bias, privacy, and overall societal impacts.
To facilitate effective collaboration, platforms like joint workshops or collaborative research projects can be invaluable. Involving practitioners from different fields allows for a more comprehensive understanding of the multi-layered challenges that AI faces. This interaction often leads to innovative thinking and creative solutions that might not arise in isolation.
Furthermore, institutions should encourage partnerships between academia and industry, creating a two-way street where practical applications inform theoretical understanding and vice versa. This approach not only enhances research quality but also ensures that AI advancements translate effectively into societal applications, maximizing their benefits.
The Future of AI Thinking
The evolution of artificial intelligence is a journey that keeps shifting terrain underfoot. As we look ahead, the future of AI is not just a mere extension of current technologies but rather a radical transformation that could redefine our existence. Understanding the future of AI thinking is crucial for technologists, businesses, and society at large because it holds the key to harnessing its vast potentials and addressing the accompanying challenges. With emerging tools and methodologies, we stand on the brink of possibilities hitherto unexplored.
A significant aspect to consider is how predictive models will play a pivotal role in our day-to-day life as AI becomes more integrated into various sectors. Organizations are working hard to create algorithms that can not only forecast outcomes but also provide insights into uncertainties that could arise from these predictions. This duality offers businesses an invaluable advantage in strategizing while navigating the murky waters of unpredictability. Funders, stakeholders, and decision-makers need to remain aware of these complexities to design frameworks that are both robust and flexible.
Moreover, the influence of AI extends beyond just business parameters. It reaches into the very fabric of our social structure. How AI integrates into society can create both opportunities and challenges that beg for thoughtful discourse and examination. As algorithms affect fundamental decisions concerning employment, education, and governance, maintaining a balanced perspective on their role is necessary. It becomes paramount to engage a diverse set of voices to inform regulations and ethical frameworks governing AI developments to ensure that the trajectories we choose add value to our human experience.
"Understanding the future of AI thinking is paramount for steering societal decisions in an informed manner that champions progress while safeguarding our ethical values."
Predictive Models and Uncertainty
The domain of predictive models within AI is vast, incorporating elements of statistics, machine learning, and data science. Their importance cannot be overstated in a climate where immediate responses to market changes can dictate the fate of organizations. These models analyze historical data to chart a course for future understanding. While they can be remarkably accurate, a significant caveat lies in their inherent uncertainty.
Key points to consider include:
- Data Dependency: Predictive models are only as good as the data they ingest. If the training data is biased or limited, predictions can go awry, leading to misguided strategic decisions.
- Dynamic Environments: Markets and ecosystems are unlike stagnant pools; they continuously evolve. Predictive models need regular updates to accommodate shifts in consumer behavior, technology advancements, and unforeseen international influences.
Unfortunately, uncertainty is the twin-edged sword of foresight in AI. As models gain sophistication, they also bear the weight of more variables, creating complex equations to solve that sometimes means less clarity. Balancing accurate predictions while navigating uncertainty remains an ongoing theme for researchers and practitioners alike.
The Role of AI in Society
As AI becomes ingrained in everyday reality, its role stretches across various societal layers. From automating routine tasks to changing how we engage with technology on a personal level, AI's presence demands a critical examination of its impact.
AI's transformative role can be summarized through several areas:
- Healthcare: AI-driven diagnostics and treatment options improve patient outcomes while also optimizing operational efficiencies in healthcare facilities.
- Education: Personalized learning experiences powered by AI help educators tailor teaching methods to better suit individual student needs.
- Public Safety: Surveillance and predictive policing through AI tools raise significant concerns around privacy and ethical implications—highlighting a need for strict governance.
The incorporation of AI should not be taken lightly. As its presence grows, ongoing discussions must surface about responsibility and accountability. Social structures must adapt to these changes while ensuring that no group is disproportionately affected, either positively or negatively. There’s a key need to engage a diverse array of stakeholders in dialogues surrounding these changes to ensure inclusivity in AI’s future.
Culmination
The conclusion serves as the final piece of our intricate puzzle regarding artificial intelligence thinking. It offers a chance to tie up threads spun throughout the article and leaves readers with a clear understanding of the discussed topics, encouraging them to ponder the implications of AI.
Summary of Findings
In summary, this exploration has revealed several critical aspects of AI thinking:
- Defining AI and its Historical Context: We delved into the evolution of artificial intelligence, starting from early computation models to modern machine learning techniques. Understanding where AI originated helps contextualize its current capabilities and future potential.
- Philosophical Implications: The nature of intelligence—especially machine consciousness—challenges our traditional beliefs about the mind and cognition. Discussions on whether machines can achieve consciousness hint at larger questions about existence itself.
- Cognitive Models: The differences between symbolic AI and connectionist approaches show various pathways through which machines can learn. Neural networks in particular mimic human cognition, adapting in real-time as they are fed new data.
- Mechanisms of Thought: We examined the processes behind data interpretation, decision-making algorithms, and how these relate to human thought processes, further blurring the lines between human and machine thinking.
- Applications and Ethics: The real-world applications of AI in sectors like healthcare and finance apply AI thinking in impactful ways, yet raise ethical concerns about bias and responsibility.
- Future Prospects: Predictive models show promise for understanding uncertainty, but the role AI plays in society will require ongoing scrutiny and collaboration across disciplines.
"Technological advances in AI are not just about greater efficiency but also about reshaping the very fabric of society."
These findings, knit together, denote how crucial understanding AI thought is as we step into an age where machines increasingly influence our lives. Readers must grasp that as AI continues to evolve, so must our frameworks for ethical governance and interdisciplinary collaboration.
Final Thoughts on AI Thinking
As we wrap up, it’s apparent that artificial intelligence isn’t merely a collection of algorithms and data; it embodies a profound shift in the paradigm of thinking itself. The study of AI thinking beckons us to reconsider what intelligence truly means. While we marvel at the capabilities of AI, we should also remain vigilant about the potential ramifications of its integration into daily life.
Engaging with AI fosters an opportunity for growth, not just for technology but for how we understand human cognition. Future developments may one day render the conversation about machine consciousness less speculative and more grounded in reality. Harnessing AI's power in constructive ways—with a clear ethical compass—promises to enhance human existence rather than undermine it.
This conclusion, while reflective, is also a call to action. It urges students, researchers, educators, and professionals alike to contribute to the ongoing dialogue about AI's role in our future, ensuring it aligns with the values we hold dear as a society.