AI Diagnosis: Transforming Medical Evaluation
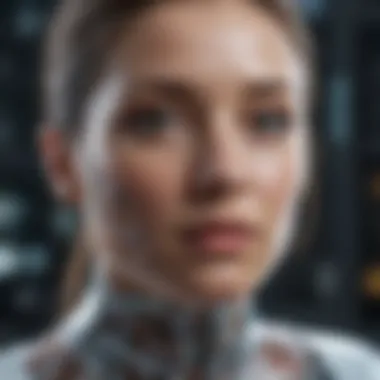
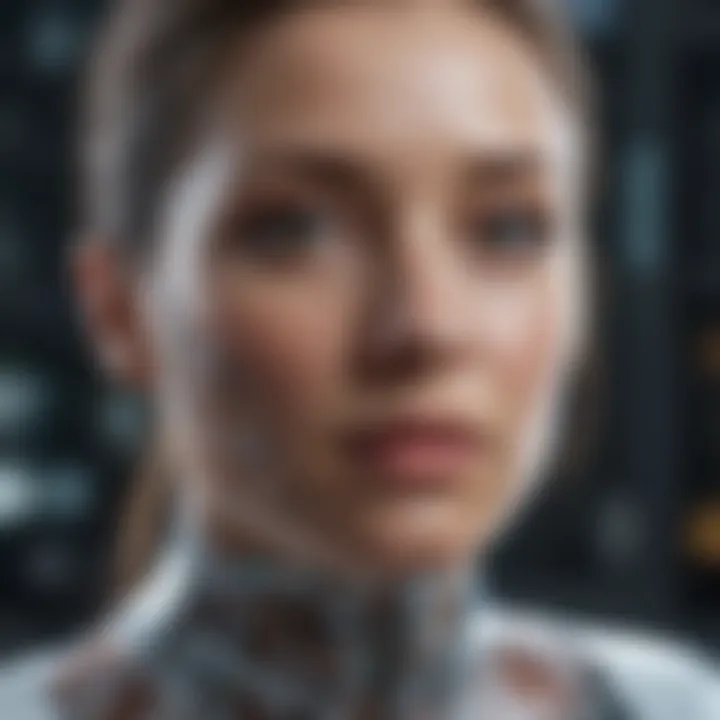
Intro
Artificial intelligence (AI) in medical diagnosis is increasingly prominent. This topic is critical for healthcare as it can greatly affect patient outcomes. In this discussion, we will explore how AI technologies are being integrated into diagnostic processes. The advancements in computer algorithms and machine learning have transformed traditional diagnostic methods. However, these changes bring both advantages and challenges.
AI diagnostic systems utilize vast amounts of data. They analyze patterns that may not be visible to the human eye. Technologies like deep learning have shown promise in detecting diseases from medical images such as X-rays and MRIs. Recognizing these patterns allows for earlier and potentially more accurate diagnoses. The implications for healthcare are substantial, as quicker diagnoses can lead to timely treatments and better patient care.
Challenges accompanying these innovations cannot be overlooked. The reliance on data raises questions about privacy, accuracy, and accountability. Moreover, there is ongoing debate about the ethical considerations surrounding AI in healthcare. In particular, how to ensure that AI systems do not perpetuate existing biases. Thoughtful consideration of these issues is paramount for the future of AI diagnosis.
Understanding AI Diagnosis
Understanding AI diagnosis is crucial in today’s healthcare landscape. As artificial intelligence technology develops, its integration into medical diagnosis transforms the way healthcare professionals approach patient care. The advent of AI enhances diagnostic capabilities, leading to improved patient outcomes. In this section, we will explore the definition of AI in healthcare and examine its historical context.
Definition of AI in Healthcare
Artificial intelligence in healthcare refers to the use of computational algorithms to assist in various medical activities, mainly diagnosis and treatment planning. AI systems analyze vast sources of data, including patient history, symptoms, and other medical inputs to make informed predictions.
The implementation of AI in healthcare aims to augment the capabilities of medical professionals. By processing large data sets with accuracy and speed, AI can uncover patterns in medical information that might be too complex for human analysis alone. Such technology has shown potential in supporting clinical decisions, ultimately improving the quality of care delivered to patients.
Key Elements of AI in Healthcare:
- Data Analysis: AI systems perform complex data analysis to identify trends and anomalies in patient data.
- Predictive Analytics: Algorithms utilize past medical data to predict potential health issues.
- Decision Support: AI provides healthcare professionals with recommendations based on analyzed data.
Historical Context and Development
The development of AI in healthcare is relatively recent but has its roots in foundational computer science research. The journey began in the 1950s when pioneers initiated research into how machines could mimic human reasoning. Over the decades, advancements in machine learning have paved the way for AI applications in healthcare.
In the early 2000s, the focus shifted towards leveraging big data for enhancing healthcare services. Electronic health records became more prevalent, allowing researchers to harness the power of data. AI gradually found its place in technology like diagnostic imaging and patient monitoring systems.
Several key milestones mark the evolution of AI in healthcare:
- Early Expert Systems: These were among the first AI applications that offered clinical decision support.
- Data Mining Techniques: As medical data grew, data mining techniques started to play a critical role in analysis.
- Deep Learning: The introduction of deep learning in recent years has significantly improved the accuracy of diagnostic imaging.
In summary, understanding AI diagnosis is vital for appreciating healthcare's progressive nature. The definitions and historical contexts provided offer foundational knowledge that shapes future discussions on this transformative technology.
Mechanisms of AI Systems in Diagnosis
The mechanisms behind AI systems in diagnosis represent the foundational technologies that enable artificial intelligence to function effectively in the medical field. Understanding these mechanisms is crucial because they determine how accurately and efficiently AI can analyze data and deliver diagnostic results. Key elements include the types of algorithms used, the processing capabilities for handling extensive datasets, and the integration of AI technologies with existing medical systems.
At the core of AI diagnostic systems are various computational techniques, each with specific strengths suited to diverse medical challenges. The utilization of these mechanisms not only enhances diagnostic accuracy but also streamlines processes that typically consume considerable time and resources.
Machine Learning Algorithms
Machine learning algorithms play a vital role in AI diagnostics. These systems analyze large datasets to identify patterns that humans might overlook. By using supervised learning, unsupervised learning, or reinforcement learning, these algorithms iteratively improve their accuracy as they process more data.
Some common machine learning methods employed in this context are:
- Support Vector Machines (SVM): These operate by finding the hyperplane that best separates different classes of data.
- Random Forests: This ensemble learning method uses multiple decision trees to enhance predictive analytics.
- K-means Clustering: An unsupervised method that groups similar data points, facilitating the identification of anomalies.
The advantages of machine learning in diagnostics include the ability to process vast amounts of data rapidly and the potential for continuous learning, which leads to better patient outcomes over time.
Deep Learning Approaches
Deep learning, a subset of machine learning, uses neural networks with many layers to analyze various data types, particularly images. This approach is especially significant in areas like radiology, where it can assess x-rays and MRIs far beyond human capability.
Deep learning has several benefits:
- Feature Extraction: The system automatically identifies and learns features from raw data without explicit programming to do so.
- High Accuracy Rates: When trained on large datasets, deep learning models can significantly outperform traditional methods in image recognition tasks.
- Scalability: These models can be adapted to handle additional data and tasks with minimal adjustments.
As demands for precise diagnostic tools increase, the role of deep learning in medical AI systems continues to grow.
Natural Language Processing
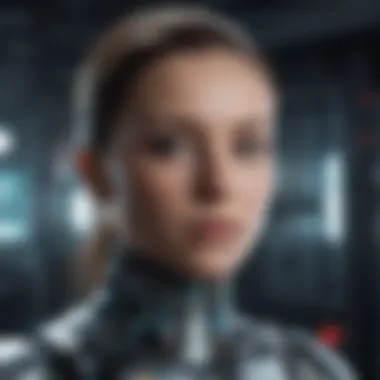
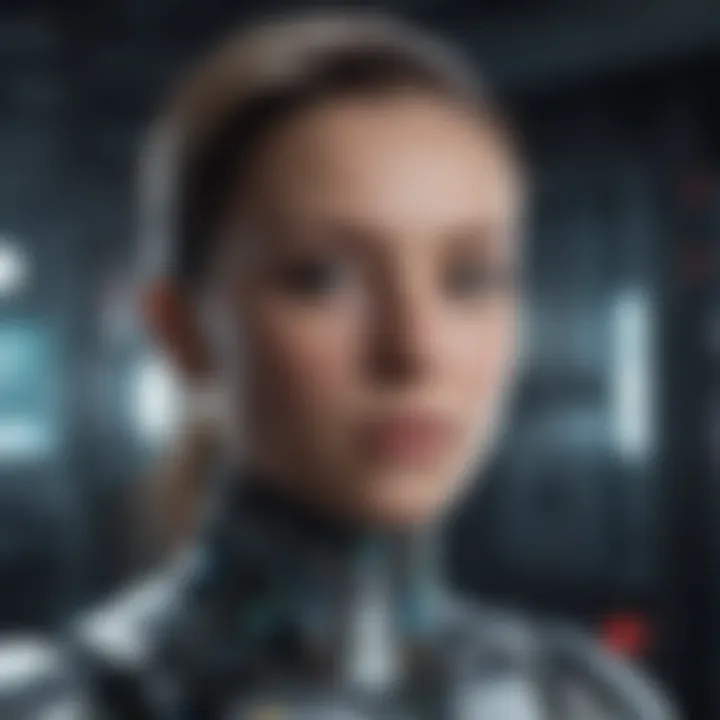
Natural language processing (NLP) is essential for understanding and analyzing unstructured data such as clinical notes and patient records. AI systems that incorporate NLP can extract valuable insights from various text formats, facilitating more comprehensive patient assessments.
Key applications of NLP in AI diagnosis include:
- Information Extraction: Identifying relevant information within medical texts to support diagnostics and treatment plans.
- Sentiment Analysis: Evaluating patient feedback from surveys and forums for insights on care quality.
- Clinical Decision Support: Offering evidence-based scenarios to healthcare providers based on the detailed analysis of past cases and medical literature.
The integration of NLP into AI systems allows for more holistic patient evaluations by combining structured data analysis with context gleaned from language processing.
"AI's integration into medical diagnosis can reshape traditional practice, enhancing accuracy and efficiency through advanced algorithms."
This brief overview of the mechanisms of AI in diagnosis highlights their critical relevance in today's healthcare landscape. Continuing improvements in these areas ensure that AI can offer increasingly reliable support to medical professionals and ultimately improve patient outcomes.
Applications of AI in Medical Diagnosis
The landscape of medical diagnosis is experiencing a significant shift due to the integration of artificial intelligence (AI). The applications of AI in medical diagnosis are not only enhancing the accuracy of identifying diseases but are also streamlining procedures in clinical settings. By utilizing advanced algorithms, machine learning, and data analytics, healthcare professionals can make more informed decisions and improve patient outcomes. This section focuses on three crucial domains: radiology and imaging diagnostics, pathology and histopathology, and predictive analytics in patient care. Each application offers unique benefits and addresses different challenges within the medical field.
Radiology and Imaging Diagnostics
Radiology is one of the most prominent fields where AI technologies have made substantial contributions. The ability of AI to process and analyze vast amounts of imaging data allows for improved detection and interpretation of abnormalities. Traditional methods often involve time-consuming analysis by radiologists, which can lead to delays in diagnoses. However, AI algorithms can analyze medical images such as X-rays, CT scans, and MRIs rapidly and with high precision.
Studies show that AI systems can achieve diagnostic accuracies that match or exceed human experts in certain situations. For instance, Google’s DeepMind has developed an AI system that can detect breast cancer from mammograms with a lower rate of false positives and false negatives compared to human radiologists. Moreover, these technologies continue to evolve, as machine learning models can improve their performance through exposure to more data.
"AI in radiology enhances diagnostic accuracy while also reducing the workload on healthcare professionals."
Pathology and Histopathology
In pathology, AI is revolutionizing the way diagnoses are made by improving the analysis of tissue samples. Pathologists traditionally rely on visual assessments, which are subjective and prone to human error. AI can assist in quantifying features that may be overlooked during manual reviews, leading to more accurate and consistent diagnoses.
AI systems can analyze histopathological slides by identifying patterns and anomalies indicative of diseases such as cancer. Technologies like image recognition and deep learning have shown great promise. For example, algorithms can detect signs of metastasis in tissue samples more efficiently. The potential for AI to reduce diagnostic errors and expedite the assessment process is significant in high-volume labs, ultimately benefiting patient treatment plans.
Predictive Analytics in Patient Care
Predictive analytics employs AI algorithms to analyze data trends and outcomes, allowing healthcare practitioners to anticipate patient needs. By evaluating historical data, AI can predict disease progression and future health risks. This is particularly valuable in chronic disease management, where monitoring ongoing patient health is vital.
For instance, predictive models can assess patient data to identify individuals at risk for conditions like diabetes or heart disease before symptoms develop. The proactive approach provided by predictive analytics ensures timely interventions, personalized treatment plans, and better resource allocation in healthcare.
Advantages of AI Diagnostic Systems
The integration of artificial intelligence into medical diagnosis brings significant advantages that enhance patient care and streamline healthcare processes. Understanding these benefits is essential for healthcare professionals, students, and researchers.
Increased Diagnostic Accuracy
AI systems, through advanced algorithms and large datasets, improve diagnostic accuracy remarkably. The ability of machine learning models to analyze complex data patterns allows for earlier detection and better identification of diseases. For instance, in imaging diagnostics, AI tools can assist radiologists by highlighting abnormalities that may be overlooked.
Improved diagnostic accuracy can lead to higher treatment success rates, minimizing the risk of misdiagnosis.
Healthcare providers can utilize AI diagnostic systems to make more informed decisions. This is particularly crucial in complex cases where multiple conditions may present concurrently, complicating traditional evaluation methods. By harnessing AI, the precision in diagnostics not only benefits individual patients but also overall public health outcomes.
Efficiency and Speed in Diagnoses
One of the primary merits of AI in diagnostics is its ability to increase the efficiency and speed of the diagnostic process. AI systems can analyze vast amounts of data much faster than a human can. Taking a closer look at time-sensitive conditions, such as stroke or heart attacks, the ability to provide immediate assessments can significantly impact patient outcomes.
In practical applications, AI tools can rapidly process lab results or imaging studies and deliver results to healthcare professionals. This reduces waiting times and accelerates the initiation of treatment when necessary.
The speed and efficiency provided by AI tools not only enhances operational workflow within healthcare settings but also improves the patient experience. The satisfaction of receiving quicker diagnoses can have a huge influence on patient trust and compliance, leading to better healthcare engagement.
Cost-effectiveness in Healthcare Delivery
Cost considerations are central in every aspect of healthcare. The advantages of AI in this field include cost-effectiveness. By improving diagnostic accuracy and efficiency, AI can lead to reduced costs associated with unnecessary tests and hospital readmissions.
AI systems can help in resource allocation by determining the most urgent cases requiring attention and streamlining workflows. A reduction in diagnostic errors translates into less spending on treatment for misdiagnosed conditions. In the long run, AI could help healthcare systems operate more efficiently, reducing the overall financial burden on both hospitals and patients.
Challenges and Limitations of AI Diagnosis
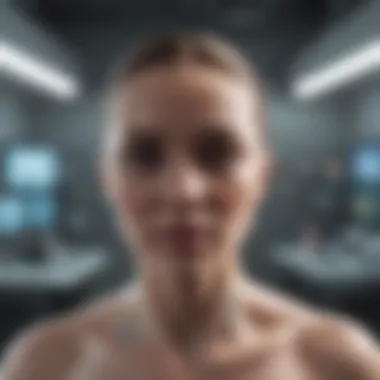
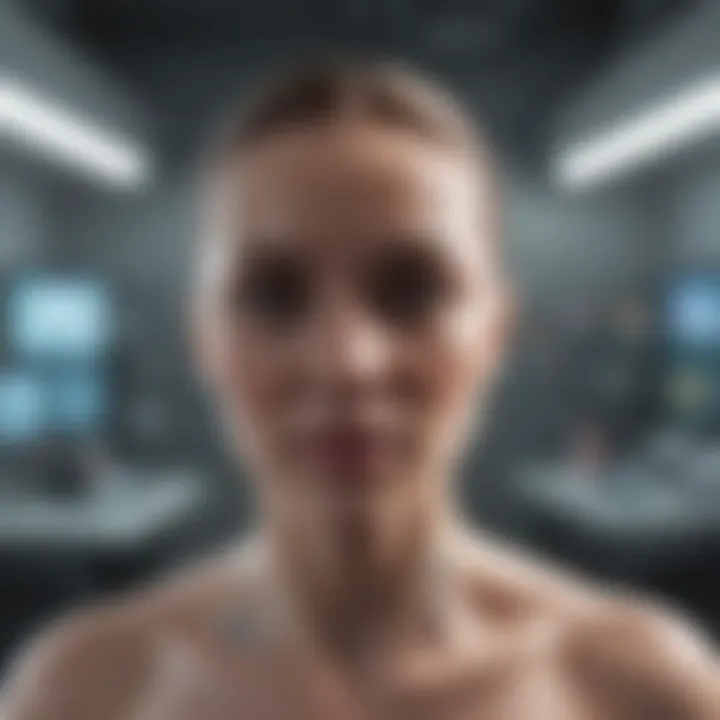
The integration of artificial intelligence in medical diagnosis holds vast potential for improving healthcare outcomes. However, this emerging field is not without its challenges and limitations. Understanding these issues is crucial for healthcare professionals, researchers, and educators who seek to navigate the complexities of AI-driven diagnostics. These elements not only inform the efficacy of AI systems but also affect their adoption and acceptance in clinical settings.
Data Privacy and Security Issues
One of the pressing challenges in AI diagnosis is data privacy and security. AI systems rely on large datasets for training, including sensitive patient information. The need to collect, store, and analyze this data raises significant privacy concerns. An unauthorized data breach could compromise patient confidentiality and lead to dire consequences.
Healthcare institutions must adhere to regulations like the Health Insurance Portability and Accountability Act (HIPAA) in the United States. These laws mandate stringent data protection measures, but the dynamic nature of AI technology makes compliance complicated.
Moreover, concerns about data ownership and consent arise. Patients may not fully understand how their data will be used or have hesitations about sharing it, impacting the quality and size of datasets. Thus, maintaining trust among patients is paramount. Only with robust security measures can AI systems function effectively without infringing upon patient rights.
Algorithmic Bias and Fairness
Algorithmic bias refers to the unintended favoritism an AI system might show due to biased training data. This poses a vital concern in medical diagnosis, as it can result in unfair outcomes in patient care. For instance, if an AI system is trained primarily on data from one demographic, its diagnostic accuracy may diminish for underrepresented populations.
Bias could have severe ramifications, potentially leading to misdiagnoses or inadequate treatment plans. Therefore, ensuring fairness in AI systems is critical. This requires a commitment to diversity in datasets and ongoing audits of AI algorithms to identify biases. Continuous training and adaptation of AI tools are necessary to mitigate this issue, ensuring they can serve a diverse patient population effectively.
Integration with Existing Medical Systems
Integrating AI diagnosis systems with traditional medical frameworks presents significant challenges. Many healthcare providers still rely on legacy systems that are not readily compatible with newer AI technologies. The transition can be both costly and complex, requiring time and resources that many institutions may not afford.
Additionally, there may be resistance from healthcare professionals who are skeptical of AI's capabilities. Without proper training and education, staff may feel ill-equipped to work alongside AI systems. Therefore, it is essential to create streamline processes that facilitate compatibility while also providing adequate training for healthcare providers.
In summary, while AI has the potential to revolutionize medical diagnostics, it encounters hurdles that must be addressed. Issues related to data privacy, bias, and system integration require careful consideration and strategic planning.
"To truly harness the power of AI in healthcare, we must navigate these challenges thoughtfully. Only then can we ensure better patient outcomes and trust in technology."
By understanding these challenges, stakeholders can better prepare for the future landscape of AI in medical diagnosis.
Ethical Considerations in AI Diagnostics
In the field of AI diagnostics, ethical considerations play a pivotal role in shaping how these technologies are integrated into healthcare systems. The adoption of AI in medical practices raises significant questions around accountability, liability, informed consent, and patient autonomy. It is necessary to address these issues to ensure that AI is used responsibly and effectively, ultimately benefiting both healthcare providers and patients.
Accountability and Liability Issues
One of the foremost concerns in AI diagnostics is accountability. When an AI system provides a diagnosis, who is responsible if that diagnosis is incorrect? The ambiguity surrounding liability can create a chilling effect on the integration of AI in clinical environments. Various stakeholders such as healthcare providers, developers, and institutions must navigate this uncertain terrain.
Legal frameworks for accountability in cases of diagnostic errors are still developing. It becomes crucial to establish clear guidelines that delineate the responsibilities of each party involved. For instance, if an AI system from IBM Watson for Oncology suggests a treatment plan and a patient suffers due to adverse effects, should the blame rest on the system, the healthcare provider who followed the recommendation, or the developers behind the technology? This complexity emphasizes the necessity of a robust legal framework that holds parties accountable while fostering innovation.
Informed Consent and Patient Autonomy
Informed consent is another critical ethical consideration. Patients should have a clear understanding of how AI systems contribute to their diagnosis and treatment. This involves transparent communication regarding the technologies used, the data collected, and the limitations inherent in AI diagnostic tools. Educating patients about AI methodologies empowers them, enabling informed decision-making.
Moreover, patient autonomy must be respected. AI should not replace human judgment but rather enhance it. Clinicians should maintain the final say in diagnostic processes, ensuring that patient preferences and individual circumstances are reflected in treatment decisions. This balance between leveraging AI capabilities and preserving clinician input is essential for ethical AI deployment in healthcare.
"Ethical considerations in AI are not just about compliance; they are about fostering trust and enhancing the relationship between healthcare providers and patients."
To foster a responsible implementation of AI in diagnostics, engaging in ongoing dialogue is necessary. Ethical frameworks must evolve alongside technological advancements to ensure a balance between innovation and patient welfare. This requires collaboration among technologists, ethicists, healthcare professionals, and patients.
Future Directions in AI Diagnosis
The field of AI in healthcare is continuously evolving. This section focuses on the future directions in AI diagnosis. Understanding this topic not only highlights advancements but also cautions against overoptimism. As AI technologies develop, the emphasis should be on balancing innovative applications with ethical considerations. Future advancements will shape diagnosis accuracy and overall patient care, making it crucial for stakeholders to remain informed and proactive.
Advancements in Algorithms and Technologies
As technology progresses, the algorithms behind AI diagnostic systems will also improve. These advancements will enhance the ability of AI to learn from data, thereby increasing precision in diagnoses. Key areas of focus include:
- Adaptive Learning: Future algorithms will be capable of self-improvement over time, as they analyze larger datasets. This means they can refine their predictive analytics without constant human intervention.
- Integration of AI with Genomic Data: Incorporating genetic information allows for personalized medicine, catering to individual patient needs.
- Enhanced Natural Language Processing: Future advancements could enable AI to understand and interpret complex medical jargon and patient histories with more accuracy. This can significantly improve diagnostic capabilities.
Moreover, the rise of edge computing will reduce latency, allowing AI to process data more quickly. This is especially beneficial in critical care scenarios where timing can be life-saving.
Collaborative Models Between AI and Human Experts
The most effective future diagnosis systems will likely be those that foster collaboration between AI and human experts. While AI can process vast quantities of data rapidly, human professionals provide context and clinical judgment that machines cannot replicate. Considerations for developing these collaborative models include:
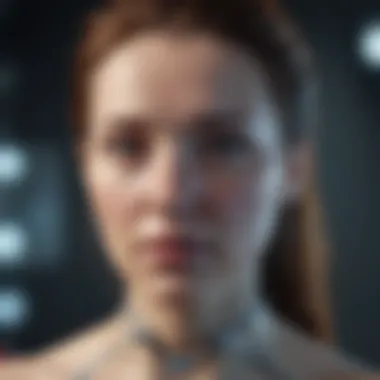
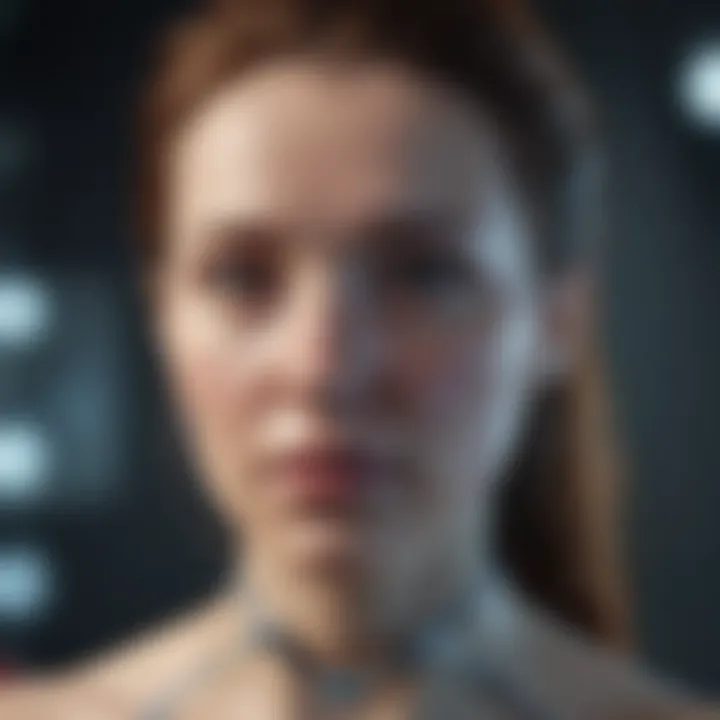
- Augmented Intelligence: Rather than replacing healthcare professionals, AI should serve as a tool that enhances their capabilities. This means providing insights that aid in decision-making rather than predictions that wholly dictate treatment plans.
- Continuous Training Programs: For healthcare providers to utilize AI effectively, they must receive training that includes the latest technologies. Ongoing education is vital to familiarize them with AI tools and their limitations.
- Feedback Loops: Implementing systems for continuous feedback from healthcare professionals allows AI systems to learn and adapt in real time based on expert input.
"The true potential of AI in diagnostics lies in effective collaboration rather than competition with human intelligence."
Fostering this synergy will lead to more accurate and personalized patient care, where AI supports medical experts in deriving insights that enhance treatment outcomes.
Case Studies: Successful Implementations
Case studies serve as pivotal examples highlighting the successful application of AI in medical diagnosis. They provide concrete evidence of AI’s effectiveness and reliability in real-world healthcare scenarios. In dissecting these case studies, healthcare professionals, researchers, and educators can glean insights into best practices, potential pitfalls, and future areas for development. The examination of successful implementations also sheds light on the adaptability of AI solutions across various medical fields, ultimately paving the way for broader acceptance in the healthcare community.
AI in Oncology Diagnostics
In recent years, AI has made significant strides in oncology diagnostics. AI algorithms analyze medical images, pathology slides, and genomic data with impressive accuracy. The deployment of AI in detecting cancers, such as lung and breast cancers, has demonstrated notable success. For instance, a model developed by Google Health analyzed mammograms and reached a diagnostic accuracy that exceeded human radiologists’ performance. This model was able to identify malignant tumors more effectively while reducing false positives.
Such advancements highlight the importance of AI in early cancer detection, which is crucial for improving patient outcomes. The precision with which AI systems can process vast amounts of data directly impacts diagnosis speed and effectiveness.
"AI systems, when properly trained, can learn patterns and nuances that may be missed by the human eye, enhancing the diagnostic process."
Integrating AI in oncology not only streamlines the diagnostic process but also aids in personalized treatment planning. By analyzing patient data, AI can help predict responses to particular therapies, thus tailoring oncological care to individual patient profiles. Furthermore, this application of AI has implications for resource allocation within healthcare systems, potentially leading to reduced costs and improved access to necessary treatments.
AI for Cardiovascular Diseases
AI's role in cardiovascular diagnostics is rapidly evolving. Machine learning models assist in interpreting electrocardiograms (ECGs), analyzing cardiac imaging, and predicting disease trajectories. One notable implementation is the use of AI in identifying atrial fibrillation from ECG readings with remarkable accuracy. This condition is a significant risk factor for strokes, and early detection can significantly alter patient outcomes.
Projects such as the one conducted by Stanford University have demonstrated that AI can match or surpass human cardiologists in interpreting ECGs. These systems learn from vast databases of patient data, allowing them to continuously improve their predictive capabilities.
Not only does AI facilitate early diagnosis, but it also contributes to risk stratification. By evaluating multiple risk factors, AI can forecast future cardiovascular events, guiding interventions timely.
In summary, AI applications in oncology and cardiology underscore the transformative potential of technology in modern medicine. The case studies emphasize how AI can complement healthcare providers, ensuring more precise, quicker, and efficient diagnostic processes.
Practical Implications for Healthcare Professionals
The integration of artificial intelligence into medical diagnostics has significant implications for healthcare professionals. Understanding these implications is crucial as practitioners navigate the changing landscape of healthcare technology. AI diagnostic systems present not just tools, but paradigm shifts that can affect various aspects of healthcare delivery. Therefore, medical professionals must engage comprehensively with the developments in AI to enhance their practice, improve patient care, and remain relevant in their field.
Training and Education for Healthcare Providers
A critical component of effectively implementing AI in medical settings is ensuring that healthcare providers receive adequate training. Education focused on AI technologies should not be a mere addition to curriculums but rather a fundamental part of medical training. Future and current healthcare providers should understand how AI works, its capabilities, and limitations to efficiently incorporate it into their practice.
- AI Literacy: Training programs should prioritize AI literacy, enabling healthcare providers to critically engage with AI tools. This includes knowledge about data sources, the functioning of algorithms, and how to interpret AI-generated insights.
- Hands-On Experience: Simulation exercises utilizing AI applications can provide practical understanding. Providers need exposure to real-world scenarios where AI assists in diagnosis to build confidence in its use.
- Continuing Education: As AI technologies evolve rapidly, continuous education is essential. Regular workshops and updates will help providers adapt to new developments and refine their skills accordingly.
Enhancing Patient-Provider Interactions
The use of AI in diagnosis can also fundamentally alter how healthcare providers interact with their patients. Understanding these changes is crucial for optimizing the doctor-patient relationship and ensuring that patient care remains at the forefront.
- Data-Driven Conversations: AI can provide actionable data that enhances patient discussions. Providers can present diagnostic results with greater clarity, fostering informed conversations. This encourages patient involvement in treatment decisions.
- Personalized Care: AI allows for tailored medical advice and treatment plans based on individual patient data. This customization can improve trust and satisfaction among patients, enhancing the overall dynamics of their interactions with providers.
- Time Management: With AI handling routine diagnostics, healthcare providers can allocate more time to engage with patients on a personal level. This human connection is vital for building rapport and understanding patient concerns more deeply.
Culmination
The conclusion serves as a crucial element in summarizing the key points discussed in this article about AI in medical diagnosis. It synthesizes the insights gathered through the various sections, emphasizing the significant role AI plays in transforming healthcare. Thorough examination of advancements, applications, and ethical concerns related to AI denotes the nuanced understanding of its impact.
Summary of Key Insights
AI diagnostic systems offer profound advantages, including enhanced accuracy, efficiency, and cost-effectiveness. These capabilities are reshaping how healthcare providers diagnose and treat patients. Furthermore, several case studies illustrate successful implementations across disciplines, affirming AI as a valuable partner in clinical settings. However, challenges such as data privacy and algorithmic bias necessitate careful consideration.
In summary, the following insights emerge:
- AI enhances diagnostic accuracy, leading to better patient outcomes.
- The technology increases efficiency by reducing diagnosis time.
- Cost savings in healthcare delivery can be achieved through AI applications.
- Ethical dilemmas arise, necessitating ongoing discussions about accountability and privacy.
Final Thoughts on the Future of AI Diagnosis
Looking ahead, the future of AI in medical diagnosis is promising yet complex. Continuous improvements in algorithms and integrated systems are expected to enhance the collaborative models between AI and healthcare professionals.
The move towards more personalized medicine will likely mean that AI systems become integral in tailoring treatment plans to individual patient needs. Furthermore, healthcare education may evolve to include training on AI tools, preparing future professionals to work alongside AI technology effectively.
Ultimately, embracing AI diagnostics offers the potential for a paradigm shift in patient care, making healthcare more precise and efficient.
"The successful application of AI in diagnostics is not just about technology; it is about fostering a responsible and ethical integration into clinical practice."
AI has the ability to address some of the most pressing challenges in healthcare today, but it must be done with vigilance to ensure equity and safety. The journey is ongoing, and discussions about its implications will shape the future of health services for years to come.